Trade reporting challenges require data re-think
Data accuracy issues are hampering some firms’ regulatory reporting abilities. So why aren’t data professionals more involved? And how can compliance costs be turned into revenue drivers?
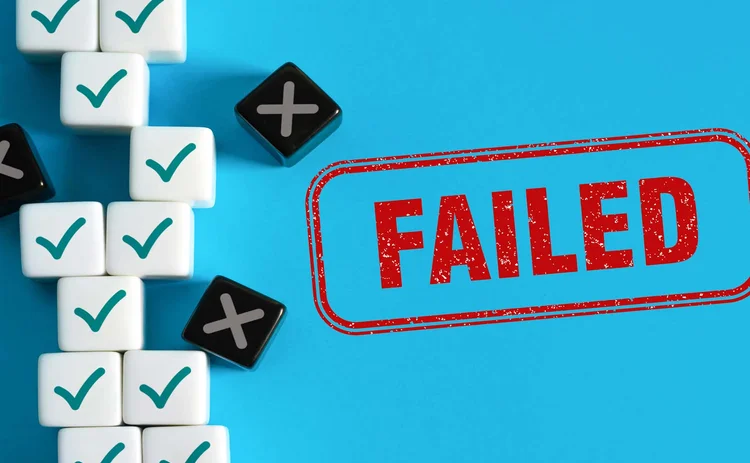
As the amount of regulation governing participants in the financial markets increases, so does the compliance burden on these firms. Many require voluminous amounts of data to report an event or transaction, with each report containing dozens of fields that must be filled out, often using data stored in different systems within a firm.
For example, when the revised Mifid II and Mifir rules came into force governing financial transactions in Europe in 2018, the number of fields in a transaction report almost tripled—growing from 23 to 65. Meanwhile, in the US, the amount of data reported for securities lending transactions will increase under the Securities and Exchange Commission’s (SEC’s) Rule 10C-1a.
And consolidated tapes on both sides of the Atlantic promise to deliver further increases in reporting requirements. In Europe, an ongoing review of Mifir is attempting to agree what data elements should be included on a proposed European consolidated tape of equities data. In the US, the Consolidated Audit Trail, which has seen trades reported to the SEC via the CAT, will introduce additional reporting requirements starting on January 31, 2025.
Until now, sources say, regulators have been content to take a light-touch approach to enforcing compliance, preferring to point out errors and work with firms to remediate them, while accepting resubmissions of erroneous or incomplete data. However, there are signs that the regulators’ patience is starting to wear thin: Last year, the Financial Industry Regulatory Authority (Finra) fined broker-dealer Instinet $3.8 million for failures to accurately report data to the CAT, starting in 2020, and required the broker to retain an independent consultant to conduct a review of the adequacy of its reporting capabilities.
There are 84 fields in a transaction report, and around 10% are high-risk, another 10% are medium-risk, and the rest are low-risk. I’m certain that almost every high-risk field could be turned into medium-risk with some automation
Alex Wolcough, GreenBirch Group
The errors, which in total affected at least 26 billion reportable events, covered timeliness and 180 other types of errors, including share quantity, handling instructions, department-type codes, customer display instruction flags, and event timestamps. In addition, Finra criticized Instinet for not having adequate written procedures and reviews in place.
In its findings, Finra noted the importance of accurate and timely reporting to market integrity. “CAT data is an integral part of Finra’s automated market surveillance program. Finra uses CAT data to detect manipulative activity and other potential violations of federal securities laws and Finra rules. Inaccurate, incomplete, or untimely transaction and order reporting can negatively affect the regulatory audit trail and the quality of Finra’s surveillance patterns, as well as Finra’s ability to accurately reconstruct market events,” the authority wrote.
“These reports are a form of investor protection,” says Adrian Whelan, global head of market intelligence at Brown Brothers Harriman. But he also notes that regulatory reporting is an ever-shifting goalpost—“continually evolving and fragmented.”
And as the volume and criticality of reporting increases, getting reporting right becomes even more important. As such, those who get it wrong should anticipate stiffer penalties in the future. Peter Gargone, founder and CEO of N-Tier Financial Services, a New York-based provider of information management software, expects the number of fines to increase as regulators become frustrated with repeated failures to report accurately.
“As the volume and complexity of regulations has grown, you find that the data [to meet their requirements] doesn’t exist in a single place within a firm. The firm may have synthesized it in different places or aggregated it in different ways. So, going back to the original data and pinning it to the regulatory reports is technically very hard,” Gargone says.
He notes that CAT reporting, in particular, is unique because of its breadth, as it requires information about the interactions of order flow. To go back over that data and look at it historically to figure out which parts are working and are correct “is a huge time effort,” he says. “And a lot of firms don’t have the technical ability to perform a resubmission over years of submissions.”
N-Tier’s platform is designed to identify reporting issues, both current and historical, across an array of regulatory regimes. In many cases, N-Tier will be engaged as part of remediation efforts after a firm has already received a fine or undertaken multiple attestations and resubmissions, Gargone says. By combining its technology platform and background in data quality, reporting and remediation, the vendor is able to detect and address further inconsistencies before they become a problem, while providing control and assurance over the completeness and accuracy of the firm’s remediation program, he adds.
BBH’s Whelan says that with so much potentially at stake, firms are showing a willingness to re-think the data aspects of their reporting. “What used to be a tactical reactive solution to each and every regulation … now firms are pausing to look for a strategic solution.”
Common challenges
While banks and brokers may shoulder the heaviest burden, they aren’t the only ones subject to reporting rules.
“These problems are common to banks, asset managers and trading venues,” says Alex Wolcough, founder and CEO of London-based consultancy GreenBirch Group.
In bilateral trades, each party must report their side of a transaction, but for trades executed on trading venues, the venue itself must also report, Wolcough notes. Indeed, it was reporting challenges encountered by an exchange client of GreenBirch that led the company to set up a new division, dubbed GreenBirch Data, to help market participants and operators demonstrate effective data governance to regulators.
GreenBirch’s exchange client had already been fined for issues relating to the timeliness and completeness of its reporting, a result of omitting fields that it had believed to be “optional” but were actually “conditional”—i.e., that those fields must be reported in certain circumstances.
The regulator noted that the exchange had addressed its reporting errors, but then asked a more fundamental question, Wolcough says: “Your reports are now complete and timely, but are you sure that everything is accurate?”
Wolcough believes a contributor to errors, and to their ability to slip through firms’ checks and procedures, is the adoption of agile development methodologies—not agile itself, but rather the way firms have implemented the methodology. He notes that the industry is moving away from the waterfall methodology, where there would be a document that extracts information from the regulation and translates it into a “functional requirements” document that someone could “code against,” he says.
“More recently, people have switched to a ‘sprint’ methodology, where they say, ‘We don’t need that document upfront; we can be more agile by building stories and coding against those, getting the requirements as you go, rather than documenting everything upfront.”
After paying its fine and fixing its reporting, the exchange went looking for its original functional requirements document and realized it didn’t have one.
GreenBirch’s approach involves mapping existing data lineage and performing a risk assessment, establishing data quality principles and standards, and reviewing existing controls and building the requirements for new ones to monitor any shortfalls in data quality. Part of this process involves understanding which fields in a report may have been entered manually versus which have been captured automatically from another system. The reason is that different systems may treat fields differently, such as one system considering a field mandatory, while another considers the same field optional. From there, it considers which fields are high-risk versus low-risk.
For example, manually captured data items, such as an LEI or a passport number, are considered high-risk, so a firm can use a control like Google Cloud Vision to request additional information, such as a photograph of a passport in addition to the passport number, and can match them together, Wolcough says. Or, since LEIs can change if a company’s status changes, a firm could implement a control to check LEI databases every week.
“There are 84 fields in a transaction report, and around 10% are high-risk, another 10% are medium-risk, and the rest are low-risk,” he says. “I’m certain that almost every high-risk field could be turned into medium-risk with some automation.”
GreenBirch’s exchange client is currently in the process of reviewing all its high-risk fields, Wolcough says.
“By ensuring checks are done before the creation of a report … you should find that the number of errors found in spot checks is dramatically reduced as a result of doing this up front. So, it should be a rare event that you find an error.”
Missing link
However—perhaps unusually, given that reporting issues generally stem from data issues—N-Tier’s Gargone says that in his experience, responsibility for tackling these issues lies with compliance or regulatory operations teams, rather than chief data officers. So far, he hasn’t seen CDOs being a part of these conversations.
And maybe that’s where firms are going wrong. After all, who better to ensure the accuracy of the data being used in regulatory reports than the one person in an organization with ultimate responsibility for ensuring the accuracy of the data it holds?
Once a firm’s data lineage—including the ability to map data from an endpoint back to its original source and determine which are low-, medium-, or high-risk—is properly organized, responsibility for each aspect of it can be assigned to specific individuals responsible for managing it.
“A chief data officer should have, in any organization, a data steward for each system who is responsible for making sure everything is accurate as well as complete and timely,” Wolcough says.
Those stewards, with GreenBirch, can then design whether something classified as high-risk can be made medium- or low-risk.
Walcough says this allows a firm to tell a regulator that they know where the data is coming from, that they have control over that data, and they have a high degree of confidence that the data is accurate. “It’s unlikely that all data will always be 100% accurate, but—using controls—you can prove to regulators that reports are accurate today, and if something changes in the future, that you are using systems that will capture these processes and make sure they are capturing all the data accurately for the future.”
Big Brother and beyond
Most data investigations and remediation efforts are triggered by regulator reviews, Gargone says. But the costs involved—from fines to other costs resulting from inaccurate reporting—should be sufficient incentive for firms to be more proactive about cleaning up their data to ensure correct reporting. Fines themselves don’t remain static: the penalties grow as the number of infringements increases—potentially leading to a firm being shut down by the Federal Reserve if it repeatedly ignores penalties and orders to correct its problems. And there are other costs that can mount up.
“Resubmission efforts tend to involve lawyers. These get very time- and resource-intensive, so they can become quite expensive,” says Gargone, who estimates that correcting data issues after discovering errors—or after regulators discover errors—costs five times as much as the cost of getting it right in the first place.
“If you just correct the problem one time and don’t change the processes that produced the error, you’re still in the same position,” he says. And that means the same risks exist, and the potential for penalties also still exists.
But it’s better to get things right up front than fix them after the fact. And firms can’t assume that regulators won’t spot errors, or delve deeper once they find an initial error, especially with so many reporting regimes requiring similar data—for example, CAT, Electronic Blue Sheets (EBS) files of trade and account data, OCC reporting, Reg 605, and Reg 606 reports in the US alone. If a firm displays an inability to report data accurately for one regime, it’s likely to also be reporting inaccurately for others.
“If you find data wrong in a CAT report, that might also have an impact on Reg 605 or 606 reporting. That’s why it’s best to invest up front in cross-regulation reporting,” Gargone says. “Regulators are learning and investing in their processes over time. They are improving their technology, and if something’s wrong, they’ll find it.”
BBH’s Whelan agrees. “Regulators are getting better at observing poor and untimely data, and are starting to use big data and tools like artificial intelligence to monitor and assess datasets.”
But there’s another aspect to data quality efforts beyond just accurate, complete, and timely reporting that is leading firms to reimagine the motivation for these programs. For example, BBH is building a single data repository that will allow it to use data that can be guaranteed accurate for other purposes, such as in a machine-learning tool that learns from reconciliations issues. By using this tool in the periods of volatility during the Covid-19 pandemic, BBH was able to realize “significant” savings, Whelan says.
By being able to leverage the confidence it has in its data, BBH is able to act as not just a custodian, but as a “data connector” to its community of clients and counterparties.
Herein lies one of many potential unintended payoffs of data quality initiatives: Because large banks and asset managers experience the biggest problems of “data dispersion,” they need a strategic solution to the challenges of reporting, Whelan says. But once in place, that strategic solution can be used to solve for more than just reporting. And once firms stop thinking of these data efforts on a regulation-by-regulation basis, they’ll see that what was once a compliance-related data cost can become an investment in driving new business initiatives that can generate revenues.
Further reading
Only users who have a paid subscription or are part of a corporate subscription are able to print or copy content.
To access these options, along with all other subscription benefits, please contact info@waterstechnology.com or view our subscription options here: http://subscriptions.waterstechnology.com/subscribe
You are currently unable to print this content. Please contact info@waterstechnology.com to find out more.
You are currently unable to copy this content. Please contact info@waterstechnology.com to find out more.
Copyright Infopro Digital Limited. All rights reserved.
As outlined in our terms and conditions, https://www.infopro-digital.com/terms-and-conditions/subscriptions/ (point 2.4), printing is limited to a single copy.
If you would like to purchase additional rights please email info@waterstechnology.com
Copyright Infopro Digital Limited. All rights reserved.
You may share this content using our article tools. As outlined in our terms and conditions, https://www.infopro-digital.com/terms-and-conditions/subscriptions/ (clause 2.4), an Authorised User may only make one copy of the materials for their own personal use. You must also comply with the restrictions in clause 2.5.
If you would like to purchase additional rights please email info@waterstechnology.com
More on Data Management
New working group to create open framework for managing rising market data costs
Substantive Research is putting together a working group of market data-consuming firms with the aim of crafting quantitative metrics for market data cost avoidance.
Off-channel messaging (and regulators) still a massive headache for banks
Waters Wrap: Anthony wonders why US regulators are waging a war using fines, while European regulators have chosen a less draconian path.
Back to basics: Data management woes continue for the buy side
Data management platform Fencore helps investment managers resolve symptoms of not having a central data layer.
‘Feature, not a bug’: Bloomberg makes the case for Figi
Bloomberg created the Figi identifier, but ceded all its rights to the Object Management Group 10 years ago. Here, Bloomberg’s Richard Robinson and Steve Meizanis write to dispel what they believe to be misconceptions about Figi and the FDTA.
SS&C builds data mesh to unite acquired platforms
The vendor is using GenAI and APIs as part of the ongoing project.
Aussie asset managers struggle to meet ‘bank-like’ collateral, margin obligations
New margin and collateral requirements imposed by UMR and its regulator, Apra, are forcing buy-side firms to find tools to help.
Where have all the exchange platform providers gone?
The IMD Wrap: Running an exchange is a profitable business. The margins on market data sales alone can be staggering. And since every exchange needs a reliable and efficient exchange technology stack, Max asks why more vendors aren’t diving into this space.
Reading the bones: Citi, BNY, Morgan Stanley invest in AI, alt data, & private markets
Investment arms at large US banks are taken with emerging technologies such as generative AI, alternative and unstructured data, and private markets as they look to partner with, acquire, and invest in leading startups.