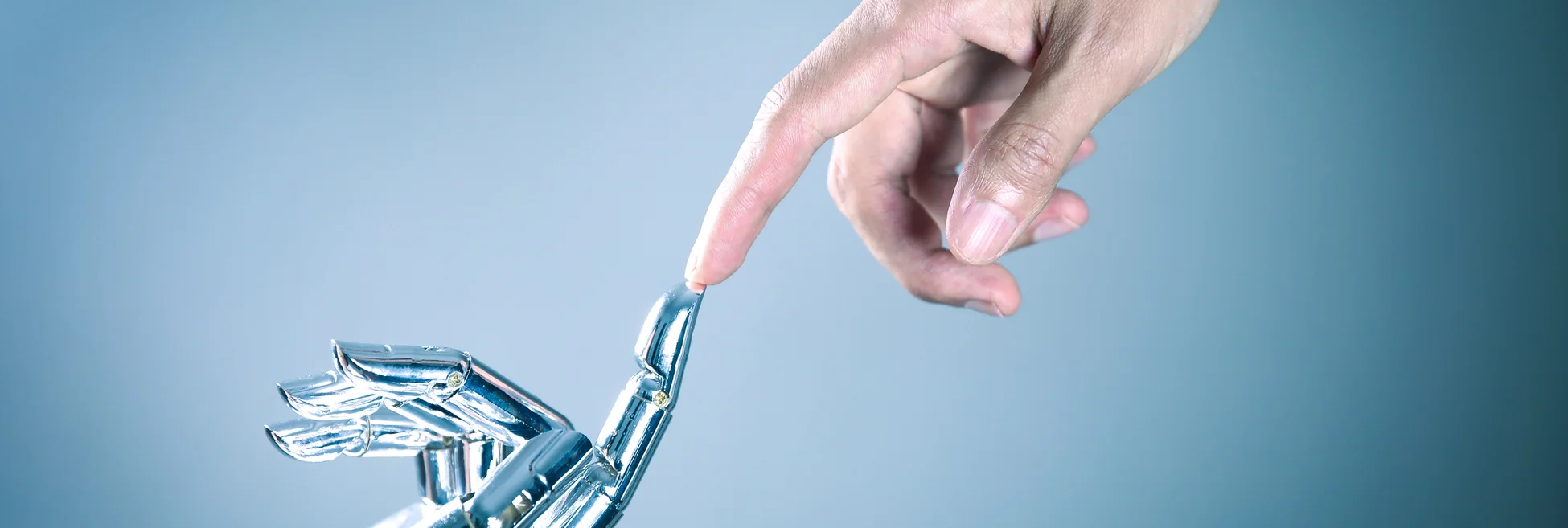
UBS AM’s Quant & Data Science Unit Looks to Blend Human & Machine in 2021
The QED team within the asset manager is aligning its focus next year to deliver a holistic AI platform to its investment professionals—including a recommendation engine.
Need to know
- The QED team aims to drive adoption of data science and AI amongst the asset manager’s investment professionals by building AI that generates investment conclusions, rather than building piecemeal, customized projects.
- QED is also working on a Netflix-esque recommendation engine for analysts that extracts info from previous investment decisions and spits out recommendations on new securities.
- This is challenging, though, as cautious value investors, for example, do not favor things the same way someone watching a movie at home “likes” a film.
- The team is working along with the firm’s investment professionals to better “emphasize” with how their investment processes work, and build tools around those “mental models”.
Empathy is not a word that is often connected with the investment industry, which likes to present itself as ruled by the head rather than the heart. But for Bryan Cross, it is at the core of what makes a good investment professional.
The head of UBS Asset Management’s quantitative evidence and data science unit, known as QED, says empathy is “the secret sauce” of being human, the key to making forecasts and solving problems, a critical attribute of those who manage the assets of others. And it forms the basis for QED’s focus going into 2021 and beyond—partnering the empathetic capabilities of human investment professionals with artificial intelligence (AI).
“The real value-add that humans bring to the [investment] equation is their ability to do complex learning tasks, to be able to look into the future. And it’s about being able to understand the motivations and intentions of both the people involved in the particular business that you are about to invest in, or the goals of your own stakeholders and asset owners. Are they saving for retirement? Do they have philanthropic goals, or an environmental, social and governance (ESG) focus?” Cross says.
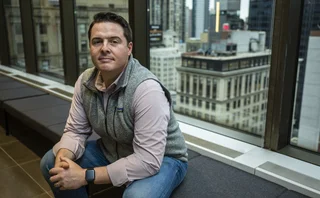
QED, which WatersTechnology profiled last year, was set up about two-and-a-half years ago within the asset manager to incorporate quant and data science principles into the traditional fundamental investment process, working toward welding the long-term, complex thinking of humans with the quantitative, evidence-based decision-making of AI.
As Cross wrote in an editorial in WatersTechnology in November, while analogous efforts across the industry are established, the vast majority of actively managed assets are still non-quantitative in nature; seduced by the hype around alternative data, participants in the field are rushing to outperform one another with niche data to reach task-specific solutions, rather than thinking about solutions that could generalize across a broad spectrum of investment problems.
Cross believes that because markets are forever changing, the best investors are generalists who apply “mental models”—systems of thought that they use repeatedly to make decisions, like handymen selecting the right tools from their toolkits. QED’s approach is to use AI to understand what these models are, and then make personalized recommendations to investment professionals.
QED has already spent a lot of time learning about analysts’ workflows to understand how they make decisions, in parallel with “record engagement” of UBS’s investment professionals with the unit, globally and across asset classes, in 2020. The next step for the unit is to provide portfolio managers and analysts with AI-built, data-driven solutions that can automate drudge work and free up their time to do the higher order, value-add thinking. In 2021, QED’s focus will shift to generalizing the investment professionals’ workflows and combining them with heuristics to form investment conclusions—buy, sell, hold, Cross says.
We have that direct connection into the investment teams, so that we can understand and empathize with what they are trying to accomplish. That isn’t easily extracted from pure data mining.
Bryan Cross
He thinks QED can deliver a holistic platform that will enable the asset manager’s investment professionals to partner with AI all along the chain of the investment process.
“One of the ways in which we are going to drive increased adoption among our investment professionals of data science and AI is to build AI that generates investment conclusions, rather than doing piecemeal, customized projects as a consultant. We need to build a platform that starts at the top of the funnel in that search function, goes down to the evaluation function, and then ultimately spits out a recommendation,” Cross says.
“And each one of those different steps—from the top of the funnel to the middle of the funnel, where you do the evaluation, and the bottom of the funnel where you make a recommendation—can be consumed by analysts, portfolio managers, other bots, even external parties potentially,” he says.
Cross doesn’t use the word “chain” to describe the investment process, though; rather, he imagines it as a funnel, with search and selection functions at the top, evaluation functions in the middle, and an investment conclusion at the end. QED could provide tools for use all the way along the funnel, which users can pick and choose to use together or in isolation.
“So at the very top of the investment ‘funnel,’ we have things like our recommendation engine, which is a machine learning, AI-driven recommendation engine that considers a portfolio and recommends new securities to take a look at,” Cross says.
That would comprise the search functionality. “Then one level down, on the evaluation functionality, now we take all the data we have—from alternative data to fundamental data, structured and unstructured—and synthesize that into an investment conclusion,” he says.
Many of the component parts of this platform are in place already, such as an automated write-up solution for fixed-income analysts. When QED started out, the unit was primarily focused on working with equities products, but in 2020, it expanded to working with fixed-income teams, and that is now rivaling the equities business, Cross says. The product for the fixed income teams leverages natural language generation (NLG) and the data that UBS consumes from vendors to deliver an automated write-up—a summary of the investment thesis of a security or company that analysts use to communicate the thesis to their team—to an analyst. This is a helpful task to automate in fixed income, Cross says, because analysts have a different scale problem than they do in equities: While there are thousands of issuers in both asset classes, unlike in equities, fixed-income issuers have issued thousands of securities that need to be analyzed.
Other components that will form part of the platform are still in development. One of the most ambitious of these is the recommendation engine, which Cross hopes will deliver personalized recommendations of investment opportunities for analysts and portfolio managers to consider.
Other industries, like retail and media, already do this kind of thing well, Cross says. Take Netflix, for example: If you spent your Thanksgiving bingeing romantic comedies on the streaming service, chances are that it now throws up a bunch of suggestions for The Holiday or You’ve Got Mail. If you love Cameron Diaz or Meg Ryan, you might give these recommendations a thumbs up (on Netflix that is called “I like this”), and Netflix builds up an understanding of your tastes over time. Perhaps an AI-driven recommendation engine could do the same thing for an analyst, extracting information from their previous investment record, and spitting out recommendations on new securities, leveraging UBS’s data.
To do this, the engine will use artificial general intelligence and the work that QED has done in understanding the investment professionals’ workflows and their mental models.
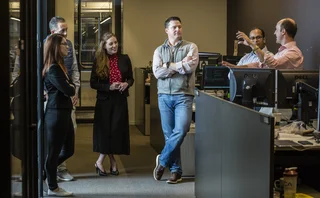
The asset management firm, one of the world’s largest, has seasoned portfolio managers with long track records of investment decisions made over the years, Cross says. This data becomes the basis for understanding their mental models, which QED is doing by using machine learning to extract commonalities that show up in portfolios repeatedly over time.
“Analysts and portfolio managers can never understand and fully appreciate all the complexities of the world. So they say, ‘OK, well, I have a mental model around value,’ or ‘I have a mental model around industrial utilities,’ or something like that. And then that provides structure to how you would evaluate a particular company. So the AI portion is for understanding the mental models that humans construct, and determining when and how we can apply that in a systematic fashion,” he says.
It also becomes the basis for engaging with those portfolio managers, refining the mental models derived with machine learning in an iterative process, in which incidentally, empathy plays a central role, Cross says.
“We have that direct connection into the investment teams, so that we can understand and empathize with what they are trying to accomplish. That isn’t easily extracted from pure data mining. We need to understand their investment philosophies, where they think they have made their biggest mistakes, where they had their biggest successes,” he says.
“And they might say, ‘Oh hey, we look at low-margin, low-valuation companies, and we take a three- to five-year view’—that kind of thing. But having that direct connection with them, we can understand what is behind those decisions. And that results in a higher likelihood that they will engage in what we are producing, and that it will produce a better outcome for our ultimate stakeholders and clients,” he says.
One challenge in developing the recommendation engine is that QED has come up against what in data science is called an imbalanced class problem. If you think about the Netflix example again, it is unlikely that you will give 90 percent of Netflix’s recommendations the “thumbs down.” Cautious value investors, however, of whom there are many at UBS AM, follow that classic Warren Buffet advice of saying “no” to most investment opportunities. This is an established investment approach, but for QED, it skews the sample: Simply put, it’s difficult to build classification models that could form the basis of an engine that produces reliable recommendations when the distribution of decisions is skewed by the analyst or portfolio manager giving those recommendations a “thumbs down” 90 percent of the time.
Cross says there are various techniques, such as Bayesian statistical methods, that can upweight the “thumbs up” selections by deciding a priori what the decision should look like, and then building a model off of that. “So it’s definitely still a problem that we are working through, but there are techniques available to us that we can use,” he says.
In the longer term, QED will be able to build up enough base libraries of the mental models that it can figure out a portfolio manager’s mental model without the portfolio manager having to tell them anything, Cross says. This is still perhaps some time away, but the unit’s focus is still clear for 2021: to drive science and the use of data in the investment process, to find the best blend of human and machine.
Further reading
Only users who have a paid subscription or are part of a corporate subscription are able to print or copy content.
To access these options, along with all other subscription benefits, please contact info@waterstechnology.com or view our subscription options here: http://subscriptions.waterstechnology.com/subscribe
You are currently unable to print this content. Please contact info@waterstechnology.com to find out more.
You are currently unable to copy this content. Please contact info@waterstechnology.com to find out more.
Copyright Infopro Digital Limited. All rights reserved.
As outlined in our terms and conditions, https://www.infopro-digital.com/terms-and-conditions/subscriptions/ (point 2.4), printing is limited to a single copy.
If you would like to purchase additional rights please email info@waterstechnology.com
Copyright Infopro Digital Limited. All rights reserved.
You may share this content using our article tools. As outlined in our terms and conditions, https://www.infopro-digital.com/terms-and-conditions/subscriptions/ (clause 2.4), an Authorised User may only make one copy of the materials for their own personal use. You must also comply with the restrictions in clause 2.5.
If you would like to purchase additional rights please email info@waterstechnology.com
More on Data Management
New working group to create open framework for managing rising market data costs
Substantive Research is putting together a working group of market data-consuming firms with the aim of crafting quantitative metrics for market data cost avoidance.
Off-channel messaging (and regulators) still a massive headache for banks
Waters Wrap: Anthony wonders why US regulators are waging a war using fines, while European regulators have chosen a less draconian path.
Back to basics: Data management woes continue for the buy side
Data management platform Fencore helps investment managers resolve symptoms of not having a central data layer.
‘Feature, not a bug’: Bloomberg makes the case for Figi
Bloomberg created the Figi identifier, but ceded all its rights to the Object Management Group 10 years ago. Here, Bloomberg’s Richard Robinson and Steve Meizanis write to dispel what they believe to be misconceptions about Figi and the FDTA.
SS&C builds data mesh to unite acquired platforms
The vendor is using GenAI and APIs as part of the ongoing project.
Aussie asset managers struggle to meet ‘bank-like’ collateral, margin obligations
New margin and collateral requirements imposed by UMR and its regulator, Apra, are forcing buy-side firms to find tools to help.
Where have all the exchange platform providers gone?
The IMD Wrap: Running an exchange is a profitable business. The margins on market data sales alone can be staggering. And since every exchange needs a reliable and efficient exchange technology stack, Max asks why more vendors aren’t diving into this space.
Reading the bones: Citi, BNY, Morgan Stanley invest in AI, alt data, & private markets
Investment arms at large US banks are taken with emerging technologies such as generative AI, alternative and unstructured data, and private markets as they look to partner with, acquire, and invest in leading startups.