Untapped Potential:The Road to Semantic Heaven
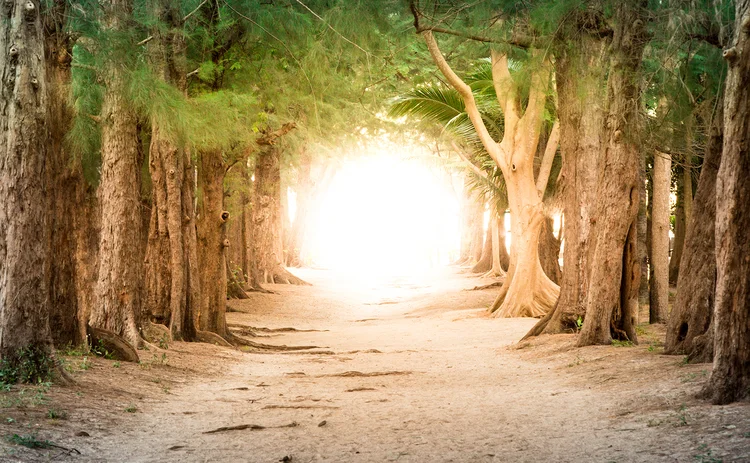
To foster a deeper understanding of the benefits of semantics, what the path from here to industry-wide standards looks like, and the challenges data leaders face along the way, it may useful to examine a case study of sorts: the humble Legal Entity Identifier (LEI).
A global standard in its own right, the LEI was conceived after the 2008 financial crisis, but it took about four years for the industry and regulators to agree on how the identifiers would be obtained, paid for, governed and maintained. Many decisions had to be made surrounding a simple 20-character alphanumeric standard before identifiers could be issued. And even then, it took the revised Markets in Financial Instruments Directive (Mifid II) to amplify the message of the LEI, communicating the need for identifiers and, in many cases, making them mandatory.
Semantic ontologies, which precisely define data points and provide representation for how they interrelate, are traveling down a similar path. The data problem—where the underlying technology that drives the financial industry has been built up and is managed in silos, which results in repositories of data aligned vertically to their applications—is well-known. Mature standards exist, and there is a collaborative effort underway to improve, implement and adopt them. The technology required to make it all work is under development or already built, and there is near-universal agreement about the potential of semantics to unleash unprecedented data insights.
It’s just a matter of getting there.
Regulatory Reformers
Much like the establishment of the LEI, regulators have the power to raise the profile of semantics, and stand to gain from the adoption of standards.
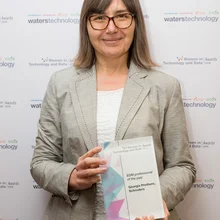
“Having financial organizations and regulators use a common language will be fantastic,” says Georgia Prothero, principal data modeler at Schroders. “Currently, regulators issue new directives that inevitably require some sort of reporting and every organization then needs to spend time and effort understanding the regulation and generating data that supports it. Imagine how different it would be if the regulator provided a semantic ontology with clear definitions of the data they want and the relationships between that data. Millions would be saved across the industry in analysis efforts.”
Immediately following the financial crisis, when regulations were being written, most regulators didn’t have a chief data officer (CDO) or a data department, and policymakers wrote many of the new rules, with good intentions, but while ignoring the data aspect.
“Regulation, even within the same jurisdiction, from one year to the next, might require largely the same information, but cut in a different way, and with a different set of rules and requirements around it,” says Gary Goldberg, CDO of Mizuho Bank, adding that this approach not only increases the complexity of compliance, but it makes the regulators themselves less efficient. “From a regulators’ standpoint, it makes it harder to assess systematic risk because these reports submitted by different entities contain information that isn’t additive. The data can’t readily be aggregated.”
Goldberg says that if many reports contain the same underlying data and aim to accomplish similar objectives, they could be consolidated. Right now, he adds, regulators are attempting to deploy analytics to better understand risk, where there’s exposure in the market and to ask intelligent questions, but the data is not available to support that approach.
“The perfect state for a semantic ontology is a consistent set of labels and definitions where the definitions are specific and accurate enough to ensure there’s no ambiguity,” he says. “A lot of the existing standards in the marketplace are very good, but they tend to standardize the container. Standardizing the labels helps to clarify the message protocol but how the container is populated varies and needs consistency.”
For instance, if the key data that needs to be submitted to regulators could be uniformly described through semantics, rather than reports, banks could simply file that information, as opposed to filing reports, a process that ideally would be automated. In turn, the regulators could access that information, not simply to answer a question pertaining to a particular regulation, but to answer any question the regulators want to know.
“There are a small number of key data sets but you could probably represent those in an infinite number of possible variations,” Goldberg says.
Quality Campaign
Efficiency is a big deal, but quality is a bigger deal, both for regulators and market participants.
When asked what the industry has to gain by adopting semantic ontologies, David Saul, senior vice president and chief data scientist at State Street, puts it succinctly: “To get to the heart of it; it’s data quality.”
Poor data quality is an issue in and of itself, but more troubling, it is the kind of problem that compounds.
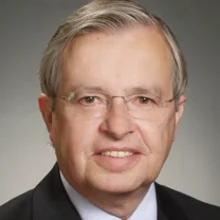
“In conversations I have with regulators, in multiple different countries, they tell me their biggest problem is data quality,” Saul says. “If regulators can’t trust the data they’ve received, when they try and aggregate that data and do their job, which is to reduce risk in the industry, starting with various pieces of questionable data and adding them together creates even more questionable data.”
Whenever two parties walk away from a trade lacking the exact same understanding and interpretation of all the data fields, it leads to miscalculation and confusion. Saul says a lot of reconciliation work is one party saying to another party, “Oh, I thought you meant something else,” and tying definitions down through semantics leads to precise calculations, the elimination of reconciliation and ultimately, vastly improved data quality.
“Having ontologies means that I can avoid translating between those different definitions, and I can do it using computer technology and automate the process. I don’t need to have a human being in the middle,” Saul says.
A common standard would improve data quality by facilitating faster, better and smoother flow of information around the industry.
“Mature standards and ontologies make settlement operations less ambiguous, with fewer exceptions,” Goldberg says. “There would be less complexity in business operations internally within organizations and between organizations. If we look beyond efficiencies, the consistent representation of data through a common ontology allows greater opportunities for analytics and data science. It’s very hard to run modeling and machine learning against data that isn’t consistent. Most data science teams spend a lot of time cleaning and standardizing data. We can instead spend the time focused on the models and the technology to drive value for customers and be more efficient in the process.”
Process Meets Profits
The biggest potential payoff in implementing semantics is also the most ephemeral: the possibilities for insights unlocked when widespread, workable semantic ontologies are in place.
“When we first took the interest-rate swap data and mapped it semantically and created that semantic map, and then we showed it to business operations people—these are the people who are heads down, pushing the transactions through on a daily basis—they could visually see the data and how it related to one another,” Saul says, a view that “gave them all kinds of ideas through views of the data that they possibly might have gotten before, but they couldn’t see them with the same degree of immediacy. To me, that’s really exciting. That opens up business opportunities.”
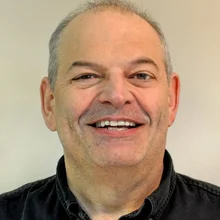
Jim Northey is a technical committee co-chair for the FIX Trading Community and in January 2019, will become chair of Technical Committee (TC) 68, which authors, supports and maintains ISO 20022, a key financial services standard currently under revision with the goal of adding semantic capability. In the past, however, he worked for a large Chicago hedge fund. When describing the advantages ontologies bring, there is often a focus on post-trade insights, but Northey warns against overlooking the benefits they can bring upstream.
“If I were a hedge fund and I had the right semantics person and the access to not just reference data but other forms of data in a semantic format, I could start to uncover relationships in terms of the underlying signals for trading. I would have a much more powerful toolset for discovering new relationships and signals that might help and inform my trading,” he says. “Given the openness of the semantic model, it can be used as another way of integrating disparate data sources readily that might be much easier and more flexible and dynamic than doing it with our static database structures and existing tooling. That’s another area that could permeate from trading all the way through the processing.”
Matthew Rawlings, CDO for Bloomberg Enterprise Data, says another key benefit of semantics is that it allows firms to capture data not yet in their data model or worldview—data that is unanticipated.
“That’s groundbreaking, because you’re capturing information before it’s needed and it’s data that might be needed later. In the past, when I was doing this back in the 1990s, we struggled with performance and making things efficient, working on the computers of the day. Now, we can store so much data, we can go so fast—our problem is managing complexity,” Rawlings says, adding that this is where the technology saves the day.
The flip side, Saul says, is that risk can be identified early via concentrations of activities in a particular currency or country that could prevent problems from the onset. “I’d like to believe that if we had this in place we could have identified Lehman Brothers’ difficulties a lot earlier and maybe done something about them,” he says.
According to Northey, banks and enterprise data providers are already using the toolsets, and although he sees “some challenges in the tooling area in standardization,” the tech and database structures are sufficient to get to work.
“There are learning curves. We’re all still learning, and there is a level of expertise required to really operate some of these tools that you’re just not going to turn it over to your normal reference data person at a bank. My concerns are around standardization across tooling, which we’ve always had as a problem, the level of interchange between tooling and the level of knowledge and the expertise needed to actually gain some of these benefits,” he says, adding that the movement is toward fewer workers who are more highly skilled. “Reference data often was hordes of people, just sifting through manually cleaning up data. We’re starting to automate a lot of that now. I’ve seen enough real things being done in banks with this stuff to know that the time is right now.”
And it’s not enough to simply have the right people in place: They have to collaborate.
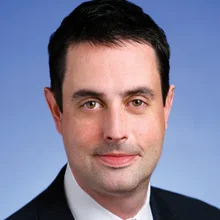
“It really comes back to, as much as you have some of these initiatives succeeding, as long as they’re succeeding in a silo, you’re never going to get to that more promising future world where T+2 becomes T+0 and firms are saving billions of dollars in failed trades and middle- to back-office functions are completely streamlined. As long as all of these initiatives don’t have one ring to bind them, we’re really not going to get there. It’s interesting to see which ones of these are actually going to pan out,” says Matthew Bastian, director of market and business development and West Coast operations at Cusip Global Services. “It’s natural in the early days of a hype like this, when everyone is running in the same direction, to see some initiatives that succeed and some that fail.”
In Bastian’s view, the failures are likely a project with no use case in mind—“a solution in search of a problem”—and initiatives that take use case into account early are the ones with the most promise.
“Down the road, if the industry can find a way to coalesce around standards that link all of these up, that’s where you’re really going to see the rubber hit the road,” he says, noting that there are good projects underway working from a proof-of-concept standpoint, “but as long as it’s all not disintermediated and not connected the way it needs to be, you’re just not going to see that revolution in the markets that people were talking about two years ago.”
State Street’s Saul says the industry is seeing amplified adoption of standards because “we’ve gotten to a critical mass,” and foresees continued building upon initiatives as collaboration increases, resulting in standards that are more widespread and accurate.
Interestingly, collaboration and connection do not require all firms and regulatory bodies to use the exact same standards.
“Certainly, we see the rise of a lot of standards vocabularies cross-industry, and we certainly see industry-specific ontologies that are rising up. I don’t think the goal is to ever get to one ontology for financial services. Different people have got different views of the world, they’ve got different purposes, and they’ve got different needs. We don’t all need the same worldview. We play different roles in the financial services industry,” Bloomberg’s Rawlings says. “The ‘road to Damascus’ moment for me is we were working on the ISO 20022 standard and we were essentially creating a large canonical data model. At some point, the world moved on, and we’re getting to the point now where people are saying you can have a shared vocabulary, but different organizations can have different ways of using that vocabulary and can combine that data in different ways for different purposes.”
Rawlings’ view hints at what might be the biggest challenge for semantics evangelists: the people problem. Revolutionary change requires work, and the people necessary to do the work might need to be convinced that it’s worth the effort. And while there seems to be consensus among data managers that the payoffs are worth it, there is significant work to be done.
“Every single piece of data, and there are literally thousands of them, has to be defined. That’s why it’s taking so long. And by the way, we’ll not stop at a particular point in time. Every time someone creates a new financial instrument, we’ll have to map that. The good news is we’ll only have to map the differences,” says State Street’s Saul.
Mizuho’s Goldberg has established a forum of banks and regulators to talk through the standards in a data-focused—and not policy-driven—manner.
“Our aim is to start with an initial proof-of-concept using a small number of attributes used for regulatory reporting. Then we’ll extend that data-object-by-data-object across the financial-services universe until we have a complete set. It sounds great, and it’s very easy to say but the work to agreeing those definitions will take time. Getting clarity across even a single jurisdiction can be a challenge. But, when we consider a global standard, there are a lot of conversations to be had,” Goldberg says, and when they reach a consensus of definitions across all jurisdictions, they will need regulators to sign off and implement.
Data doesn’t go wrong in a vacuum, he says; it goes wrong because a business process made it wrong, and not only that, right and wrong are contextual—what’s right in one place can be wrong in another.
“A lot of the work that CDOs undertake in our own businesses is to get a common understanding of the data. That’s complex enough in a large company. Trying to do that on a global scale across the marketplace will take time,” Goldberg says. “But it’s important enough that we need to proceed.”
Only users who have a paid subscription or are part of a corporate subscription are able to print or copy content.
To access these options, along with all other subscription benefits, please contact info@waterstechnology.com or view our subscription options here: http://subscriptions.waterstechnology.com/subscribe
You are currently unable to print this content. Please contact info@waterstechnology.com to find out more.
You are currently unable to copy this content. Please contact info@waterstechnology.com to find out more.
Copyright Infopro Digital Limited. All rights reserved.
As outlined in our terms and conditions, https://www.infopro-digital.com/terms-and-conditions/subscriptions/ (point 2.4), printing is limited to a single copy.
If you would like to purchase additional rights please email info@waterstechnology.com
Copyright Infopro Digital Limited. All rights reserved.
You may share this content using our article tools. As outlined in our terms and conditions, https://www.infopro-digital.com/terms-and-conditions/subscriptions/ (clause 2.4), an Authorised User may only make one copy of the materials for their own personal use. You must also comply with the restrictions in clause 2.5.
If you would like to purchase additional rights please email info@waterstechnology.com
More on Data Management
New working group to create open framework for managing rising market data costs
Substantive Research is putting together a working group of market data-consuming firms with the aim of crafting quantitative metrics for market data cost avoidance.
Off-channel messaging (and regulators) still a massive headache for banks
Waters Wrap: Anthony wonders why US regulators are waging a war using fines, while European regulators have chosen a less draconian path.
Back to basics: Data management woes continue for the buy side
Data management platform Fencore helps investment managers resolve symptoms of not having a central data layer.
‘Feature, not a bug’: Bloomberg makes the case for Figi
Bloomberg created the Figi identifier, but ceded all its rights to the Object Management Group 10 years ago. Here, Bloomberg’s Richard Robinson and Steve Meizanis write to dispel what they believe to be misconceptions about Figi and the FDTA.
SS&C builds data mesh to unite acquired platforms
The vendor is using GenAI and APIs as part of the ongoing project.
Aussie asset managers struggle to meet ‘bank-like’ collateral, margin obligations
New margin and collateral requirements imposed by UMR and its regulator, Apra, are forcing buy-side firms to find tools to help.
Where have all the exchange platform providers gone?
The IMD Wrap: Running an exchange is a profitable business. The margins on market data sales alone can be staggering. And since every exchange needs a reliable and efficient exchange technology stack, Max asks why more vendors aren’t diving into this space.
Reading the bones: Citi, BNY, Morgan Stanley invest in AI, alt data, & private markets
Investment arms at large US banks are taken with emerging technologies such as generative AI, alternative and unstructured data, and private markets as they look to partner with, acquire, and invest in leading startups.