Using genAI for post-trade processing could reduce failures, fines
Shortening settlement times are pressuring firms to do more, faster. IBM’s Soren Mortensen argues that genAI and ML can help eliminate errors and speed up post-trade processes.
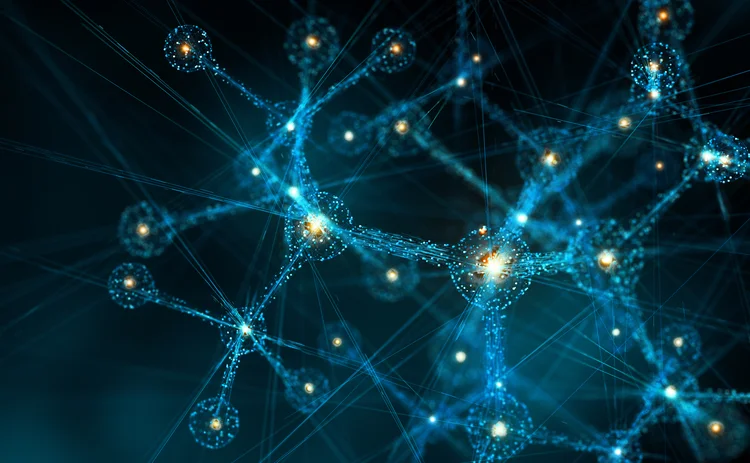
Post-trade processing in capital markets is inherently complicated. Multiple parties—including brokers, banks, clearinghouses, custodians, and central securities deposits—must handle and move data quickly to successfully transfer ownership of securities from the seller to the buyer.
Recent regulatory requirements, such as the Central Securities Depository Regulation (CSDR), add to the complexity. Officially implemented in February 2022, the CSDR’s settlement discipline regime established daily penalties for settlement failures for trades settling with a European Economic Area central securities depository that are traded on an EU trading venue or cleared by an EU central counterparty. This requires capital markets firms to implement solutions to avoid settlement delays and increase their straight-through processing (STP). AI can help with this and ensure human intervention is rarely needed.
While regulations like the CSDR are intended to increase post-trade processing efficiency and reduce risk, they can increase market participants’ costs through penalties and buy-ins. Shortening settlement cycles to T+1—the US transition is planned for May 2024—could increase these additional costs. While the EU has no formal plans to move to T+1, the Association for Financial Markets in Europe (Afme) recently announced a new industry task force to consider this possibility. At this point, it’s not a matter of if, but when, T+1 will become the norm.
Need for speed
With settlement cycles shortening, post-trade processes have to move faster. What was once done in two or three days will have to be accomplished in one. This opens the door for new inefficiencies and potentially exacerbates existing ones, such as human error. While most trades in secondary markets today are processed with a very high level of STP and settle successfully, the European Securities and Markets Authority (Esma) reported that as of December 2022, 6% of all equity trades and 4% of fixed-income trades still failed. With penalties ranging from 0.5 to 1 basis points depending on the asset, this is a significant—and preventable—cost that capital markets firms must address.
Financial institutions have always strived to increase STP rates and reduce settlement failures, so what is different today? While they can have high STP rates, this does not mean all transactions will settle on time since STP is primarily an internal process. The amount of data available today, combined with AI and machine learning, can provide the additional functionality to increase STP rates within a firm and reduce settlement failures.
In addition, it is critical that banks automate external processes, such as reconciling trade details with counterparties, and ensure the correct reference data is available for proper settlement. Adding to the urgency is the growth of digital assets, which have trades that settle automatically. This puts additional pressure on capital markets firms to streamline these processes even further across all asset classes.
Enter generative AI
Capital markets firms will need a multi-faceted approach to tackle shorter settlement cycles, and AI can play a significant role by increasing efficiency and providing post-trade insights. A generative AI platform built specifically for business that ensures the use of trusted data could capture a bank’s data over a long period of time and then detect patterns in how transactions either settle or fail by looking at the historical and real-time data.
Capital markets firms can apply AI and machine learning to the data in various ways and for various purposes. Here are a few examples:
- To manage client requests regarding balances, transaction status, corporate actions, and other basic information without any manual intervention: An example is a tier-one bank that receives several thousand emails per day from counterparties. Using a natural language model, IBM captured the content of the emails, classified and normalized the data, reviewed key details against transaction details held in the bank’s systems, and proposed a resolution. This solution directly impacted 80% of the middle-office time and ultimately reduced the time spent on this process by over 60%, enabling middle-office staff to concentrate on more value-adding activities.
- To increase securities settlement efficiency, reduce settlement failures, and prevent costly penalties and buy-ins on failed transactions: By leveraging AI-driven predictive analytics, firms can assign a risk rating to transactions to highlight those more likely to fail, along with the causes of these failures. That would enable middle- and back-office staff to take preventative measures to avoid such failures. In other words, AI can do more than just inform banks that a specific trade is likely to fail in real time—it can also offer more general insight into why certain kinds of trades typically fail. AI can even recommend exactly how to fix such settlement problems, although humans are still needed to authorize those solutions.
- To provide support and recommendations for a potential resolution and to help prevent future issues with specific products, counterparties, or currencies: With insights from AI, firms can adjust their operations and formulate long-term strategies to avoid settlement failures. They can also assess collateral eligibility and prepare operations to meet future requirements for securities and collateral.
Next steps
Amid the rapidly changing post-trade landscape, financial services companies need a way to operate faster and more accurately than ever before. Generative AI, traditional AI, and automation can provide the speed and insight necessary for banks to settle trades successfully, avoid fines, and gain a competitive advantage.
T+1 is fast approaching in the US, so the onus is now on firms to either integrate AI into their post-trade processes or bolster the AI they already have. Fortunately, putting open, trusted, targeted, enterprise-grade generative AI platforms to work on this vexing industry challenge can reduce complexity and deliver improvements in a timely fashion. Banks can bring the technology to their data, and generative AI will do the rest.
Soren Mortensen is the director of global financial markets at IBM.
Only users who have a paid subscription or are part of a corporate subscription are able to print or copy content.
To access these options, along with all other subscription benefits, please contact info@waterstechnology.com or view our subscription options here: http://subscriptions.waterstechnology.com/subscribe
You are currently unable to print this content. Please contact info@waterstechnology.com to find out more.
You are currently unable to copy this content. Please contact info@waterstechnology.com to find out more.
Copyright Infopro Digital Limited. All rights reserved.
As outlined in our terms and conditions, https://www.infopro-digital.com/terms-and-conditions/subscriptions/ (point 2.4), printing is limited to a single copy.
If you would like to purchase additional rights please email info@waterstechnology.com
Copyright Infopro Digital Limited. All rights reserved.
You may share this content using our article tools. As outlined in our terms and conditions, https://www.infopro-digital.com/terms-and-conditions/subscriptions/ (clause 2.4), an Authorised User may only make one copy of the materials for their own personal use. You must also comply with the restrictions in clause 2.5.
If you would like to purchase additional rights please email info@waterstechnology.com
More on Emerging Technologies
This Week: Startup Skyfire launches payment network for AI agents; State Street; SteelEye and more
A summary of the latest financial technology news.
Waters Wavelength Podcast: Standard Chartered’s Brian O’Neill
Brian O’Neill from Standard Chartered joins the podcast to discuss cloud strategy, costs, and resiliency.
SS&C builds data mesh to unite acquired platforms
The vendor is using GenAI and APIs as part of the ongoing project.
Chevron’s absence leaves questions for elusive AI regulation in US
The US Supreme Court’s decision to overturn the Chevron deference presents unique considerations for potential AI rules.
Reading the bones: Citi, BNY, Morgan Stanley invest in AI, alt data, & private markets
Investment arms at large US banks are taken with emerging technologies such as generative AI, alternative and unstructured data, and private markets as they look to partner with, acquire, and invest in leading startups.
Startup helps buy-side firms retain ‘control’ over analytics
ExeQution Analytics provides a structured and flexible analytics framework based on the q programming language that can be integrated with kdb+ platforms.
The IMD Wrap: With Bloomberg’s headset app, you’ll never look at data the same way again
Max recently wrote about new developments being added to Bloomberg Pro for Vision. Today he gives a more personal perspective on the new technology.
LSEG unveils Workspace Teams, other products of Microsoft deal
The exchange revealed new developments in the ongoing Workspace/Teams collaboration as it works with Big Tech to improve trader workflows.