AI model uses quantum math to learn like a human
Could the next big breakthrough in machine learning come from the world of finance?
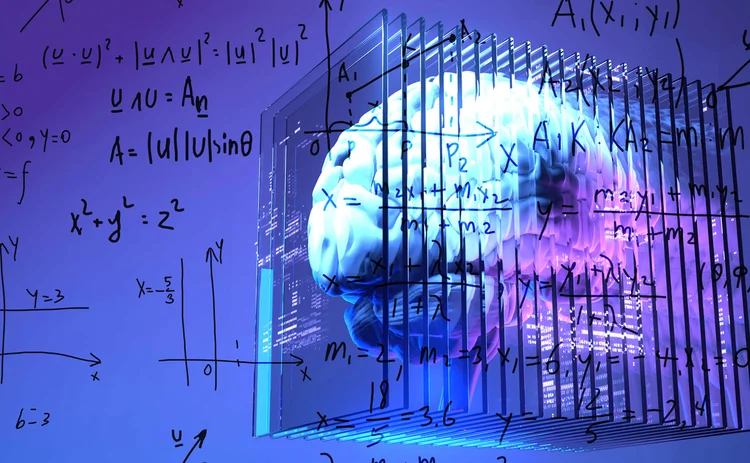
Two hedge fund quants say they have built a machine learning model that—like humans—is able to learn broad ideas from small amounts of data.
Kharen Musaelian and Dario Villani, co-founders of artificial intelligence hedge fund Duality Group, say that by using the math of quantum mechanics to encode data in a richer form, they have created a model with the capacity for abstraction and analogy that might unlock true quantum supremacy.
In a benchmark AI test, reading handwritten digits from the industry-standard MNIST dataset, the model learned even with a few hundred images. Other machine learning models might take tens of thousands of images to achieve a similar level of accuracy.
Musaelian and Villani claim this is the first example of pre-statistical learning, where a model is able to structure data into self-generated concepts and categories, as humans do.
The more data, the sharper the concept. With lots of data, the model might see a cat as a cat, with less data, as a feline. The system endogenously decides how many concepts to generate, commensurate to the amount of data, rather than following predetermined rules.
Sceptics might suspect hype, but backers of the start-up—which will operate under the name Qognitive, Inc—include tech venture capital firms Ribbit Capital as lead investor and Raptor Capital Management.
The company counts on its advisory board, among others, Alessio Figalli, a Fields Medallist, and Tomaso Poggio, a professor and the director of the Center for Brains, Minds and Machines at the Massachusetts Institute of Technology, who has worked with high-profile AI start-ups including DeepMind.
At the time of writing, Villani and Musaelian’s hedge fund, Duality Group, has just started using investing signals generated using the new approach. But possible applications in areas such as robotics and medicine go far beyond quant finance.
Because the model uses the mathematics of quantum mechanics—although for now it runs on classical computers—the approach is tailor-made for quantum computers, Musaelian and Villani say.
Quantum encoding
A key innovation has been to encode the data in a way that captures richer meaning at the cost of some uncertainty. The model encodes data “as a quantum state”—in technical terms, as a vector in a Hilbert space, Villani explains.
“Every observable in the model is an operator, not a number—in the same way as the position or momentum of an atomic particle in quantum mechanics is an operator of which you can compute the expected value but not a sharp number. It’s a number with uncertainty around it.”
Where statistical models label each piece of information and effectively isolate it from other data, the quantum model treats data as fluid—the expected value of an operator can change depending on what’s happening to other data elsewhere.
Because other machine learning models categorize data, effectively organizing it into discrete buckets, they require exponentially larger datasets to train as models increase in complexity – the so-called curse of dimensionality.
We’ve evolved to be able to process gigantic amounts of inputs without facing the curse of dimensionality
Dario Villani, Qognitive, Inc
For a stock market time series, a model might use categories, or ‘features’, such as price levels, volatility and company sector, say. To determine something meaningful about a given stock, the model must have trained on enough data with the relevant set of labels.
A model with four features that adds another feature needs enough data to learn about the fifth in combination with all other combinations of the existing four. A model with 15 features, each allowing only two possible outcomes, would have more than 32,000 combinations. The number of data points required for a model to learn about each combination could be many millions.
The Qognitive model, though, learns from all the data, rather than relying on data in the most relevant categories. Adding new features demands much less extra data to train because the computational demand grows linearly, rather than exponentially.
The new system is also able to represent relationships in the data that are context-dependent, its creators say.
Useful errors
There’s a price to pay, though. Like humans, Qognitive’s system makes mistakes. In fact, just as in the human brain, uncertainty is the cost of the model being able to learn by analogy.
“We’ve evolved to be able to process gigantic amounts of inputs without facing the curse of dimensionality,” says Villani. “But the price we pay is cognitive fallacies, as has been demonstrated in the nascent field of quantum cognition.”
This marries with the view of some AI experts that believe making mistakes is related to the ability for human-like thought. Alan Turing famously said in 1947 that if a machine were expected to be infallible, it could not also be intelligent.
AI scientists that shoehorn data into the rigid language of statistics create machines that are more certain, but require masses of data to learn. “The confines of statistics prevent the system from abstraction, creating analogies, having a sense of context,” says Villani.
Ambitions
The quants emphasize that the new approach will not supplant statistical systems, and instead has different strengths. “Newtonian physics is what takes you to the moon,” says Villani. “It’s not quantum mechanics.”
That said, the quantum approach replicates statistical learning in the limit of a small enough number of features and a large enough volume of data, he explains. Statistical learning, by contrast, cannot learn its way to replicating the quantum approach.
Qognitive has filed for patents for its processes.
Meanwhile, the quant signals generated by the model for Villani and Musaelian’s hedge fund are orthogonal to conventional signals created using the same data. That creates an opportunity to diversify across signals, Villani says.
The signals also exhibit less alpha decay, he says, most likely because other hedge funds are not trading them, and possibly because the model is able to adapt to changing markets more quickly due to the need for less training data.
Only users who have a paid subscription or are part of a corporate subscription are able to print or copy content.
To access these options, along with all other subscription benefits, please contact info@waterstechnology.com or view our subscription options here: http://subscriptions.waterstechnology.com/subscribe
You are currently unable to print this content. Please contact info@waterstechnology.com to find out more.
You are currently unable to copy this content. Please contact info@waterstechnology.com to find out more.
Copyright Infopro Digital Limited. All rights reserved.
As outlined in our terms and conditions, https://www.infopro-digital.com/terms-and-conditions/subscriptions/ (point 2.4), printing is limited to a single copy.
If you would like to purchase additional rights please email info@waterstechnology.com
Copyright Infopro Digital Limited. All rights reserved.
You may share this content using our article tools. As outlined in our terms and conditions, https://www.infopro-digital.com/terms-and-conditions/subscriptions/ (clause 2.4), an Authorised User may only make one copy of the materials for their own personal use. You must also comply with the restrictions in clause 2.5.
If you would like to purchase additional rights please email info@waterstechnology.com
More on Emerging Technologies
This Week: Startup Skyfire launches payment network for AI agents; State Street; SteelEye and more
A summary of the latest financial technology news.
Waters Wavelength Podcast: Standard Chartered’s Brian O’Neill
Brian O’Neill from Standard Chartered joins the podcast to discuss cloud strategy, costs, and resiliency.
SS&C builds data mesh to unite acquired platforms
The vendor is using GenAI and APIs as part of the ongoing project.
Chevron’s absence leaves questions for elusive AI regulation in US
The US Supreme Court’s decision to overturn the Chevron deference presents unique considerations for potential AI rules.
Reading the bones: Citi, BNY, Morgan Stanley invest in AI, alt data, & private markets
Investment arms at large US banks are taken with emerging technologies such as generative AI, alternative and unstructured data, and private markets as they look to partner with, acquire, and invest in leading startups.
Startup helps buy-side firms retain ‘control’ over analytics
ExeQution Analytics provides a structured and flexible analytics framework based on the q programming language that can be integrated with kdb+ platforms.
The IMD Wrap: With Bloomberg’s headset app, you’ll never look at data the same way again
Max recently wrote about new developments being added to Bloomberg Pro for Vision. Today he gives a more personal perspective on the new technology.
LSEG unveils Workspace Teams, other products of Microsoft deal
The exchange revealed new developments in the ongoing Workspace/Teams collaboration as it works with Big Tech to improve trader workflows.