Waters Wrap: As quantum’s skeptics grow in number, believers need better messaging
As you explore ways to use genAI, do you benefit from having ML and NLP experts on staff who have followed AI evolution for years? Anthony thinks that’s an important question when talking about quantum exploration.
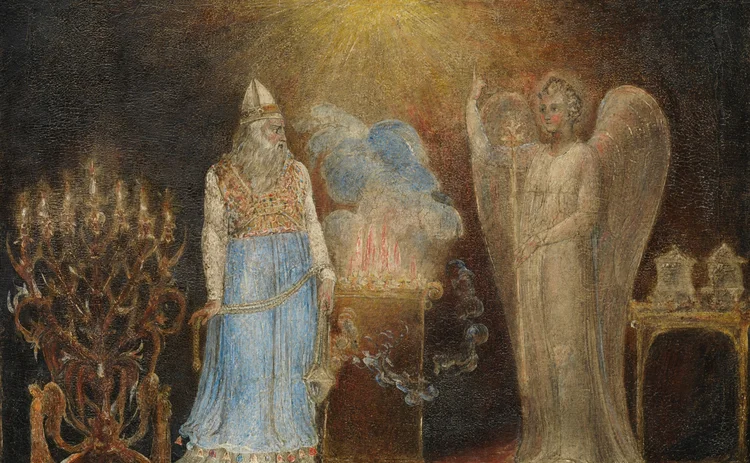
In the October 2011 issue of what was then called Waters magazine, there was a feature that asked the question, Is Wall Street ready for quantum computing? Twelve years later, we’re still basically asking the same question.
In the 1980s, physicist Richard Feynman and then David Deutsch sowed the seeds for what we consider quantum computing today: using the principles of quantum mechanics to build computational systems to run calculations and simulations that classical computers simply do not have the ability to solve today. To borrow from IBM, by utilizing “quantum phenomena” such as superposition (existing in multiple states at the same time) and entanglement (which is even more complex to explain…LOL), quantum computers can solve problems that classical computers cannot because they face limitations relating to complexity or scale.
It’s at this point that I’ll stop trying to describe quantum computing theory. The subject is fascinating and I enjoy reading about it and watching YouTube videos about it…up until the point that it feels like my brain is about to unspool.
Falling behind in the race for innovation discovery is the result of relying on years of short-term decisions—and it costs a lot to buy your way back into the game.
That said, I thought that it would be fun to provide a State of the Union for banks and quantum computing. How did we get here and where are we going?
Over the last decade we’ve written about how some of the largest investment banks are experimenting with quantum computing for things like portfolio optimization, modeling risk, calculating the value of complex derivatives and options, and for settlement.
As with any bleeding-edge technological advancement, there are evangelists and detractors. I’d say I’m in the latter camp when it comes to blockchain but in the former when it comes to something like generative AI. I also am a believer in quantum’s promise for the capital markets. But it feels like more and more banks are falling into the trough of disillusionment (even if QC didn’t fall into that pit in Gartner’s most recent report).
As our Eliot Raman Jones wrote last week, “With quantum computers not expected to be commercially viable for at least—by some estimates—another 10 to 15 years, there is growing debate on whether banks should reconsider their current investments and strategies related to quantum technology.”
Earlier this year, UBS’s former chief data officer and head of the bank’s innovation lab, Lee Fulmer, said the bank had abandoned a multi-year effort to utilize quantum computing for trading after concluding it currently offered no advantage over other technologies.
“In investment banking, you live and die by microseconds,” he said. “The end result of all of that effort was that we found we weren’t getting a substantive uplift.” UBS had concluded that the technology did not offer an immediate way of either speeding up existing machine-learning models or giving the bank an advantage over its competitors.
And a quant at a tier-one US bank that has been experimenting with quantum computing told Eliot that without the correct infrastructure behind it, investing in quantum computing is “not meaningful”.
“Quantum computing is amazing, but it cannot operate at high speed because we don’t have the right infrastructure for it,” he said. “It’s like if you have a Ferrari in London—it’s a great car, but you can’t speed because London is full of potholes and your car will break.”
It’s a swelling refrain. In October 2020, we published a deep-dive feature with this as the headline: “Science Friction: Some Tire of Waiting for Quantum’s Leap”.
In that story, Jezri Mohideen, global chief digital officer for Nomura’s wholesale business, said that his bank was likely to wait on becoming an adopter. “Two years ago, I was a lot more bullish, and I felt the evolution would come through a lot faster. Practical quantum computers can’t be used for a lot of tasks that we typically face in 98% of financial domain applications.”
And the head of digital at one investment bank said: “It always feels like we’re two years away from a real quantum use case. The order of magnitude of the efficacy of the processing just wasn’t where everybody thought it would be.”
So, in 2020, some early adopters were becoming skeptical, if not outright skeptics. Three years later, even more are dubious. Now here’s the problem—we’re still a while off from commercial quantum computers…do the QC evangelists at banks have the ability to continue to carve out a budget for what is largely a race to discovery, but not (yet) implementation?
Are you better off today?
There are no black and white answers here, but let me pose a few questions: Over the past 11 months, how many times have you been asked something along the lines of, “What are we doing with generative AI/large language models? Are we behind our competitors? How did we fall so far behind?”
While it might seem that LLMs and genAI hit the capital markets overnight, it was a slow burn that involved years-long evolutions in deep learning, natural language processing, and data structuring, acquisition and housing. Sprinkle in some high-level engineering mumbo-jumbo that I’m too stupid to explain, and you get the release of ChatGPT.
The OpenAI-developed chatbot was built on something called GPT-3, with the GPT standing for Generative Pre-trained Transformer. GPT-3 was released by OpenAI in June 2020. Those in the know were watching closely. Those that weren’t watching closely were caught off guard on November 30, 2022, when ChatGPT was officially released. And it only took a few months for this powerful chatbot to catch the eyes of technologists and laypeople, alike. Today, LLMs and genAI are on the lips of not only CTOs, but also those of CEOs and CFOs.
I do not think it’s a bold prediction to say that there will come a similar tipping point for quantum computing in the future, though no one today can predict with accuracy how distant that future will be and what that tipping point will look like.
Over the past 11 months, how many times have you been asked something along the lines of, “What are we doing with generative AI/large language models? Are we behind our competitors? How did we fall so far behind?”
One more question: When it comes to genAI, do firms today benefit from having experts on staff who have been working with deep neural networks and understand the evolution of NLP tools like Google’s Bidirectional Encoder Representations from Transformers (BERT)? I’d argue the answer is yes, even though it wasn’t that long ago that senior (non-tech) executives at banks likely had no clue what those things were.
Falling behind in the race for innovation discovery is the result of relying on years of short-term decisions—and it costs a lot to buy your way back into the game.
The believers
We do not have concrete numbers, but most of the major investment banks are still—as best we can tell via anecdotal evidence—experimenting with QC…a mass exodus, this is not. So let’s take a look at why the believers believe.
In December 2020, Goldman Sachs and IBM published a report that gave an estimate of the quantum computing resources needed to achieve quantum advantage in derivatives pricing. William Zeng, then the head of quantum research at Goldman Sachs, told WatersTechnology, “If we do it just a little bit better, because the problem is so big, it could matter a lot. So that makes it appealing as a place to start.”
Zeng later explained the reason why he thinks it’s important for banks to be early explorers of quantum technologies. “There’s a really big ecosystem out there—there’s probably $22 or $23 billion in government funding that’s been announced globally in recent years, with maybe a little more than $1 billion in private VC investment. There’s a lot of resources going toward building this technology and so we want to help guide that towards the opportunities that we see in finance.”
I haven’t spoken to Zeng since, but my guess is that he still believes in what he said to me previously—this past summer he left Goldman and is now a partner at Quantonation, a VC fund dedicated to investing in quantum technologies.
Another early evangelist is Lee Braine, who is, fittingly, the brain behind Barclays’ bleeding-edge technology efforts. He told us last year that these early experiments are “voyages of discovery that could lead to radically novel solutions for some of today’s greatest computational challenges.”
But Braine has always advised caution when managing expectations for these projects. Back in 2019, when the bank was experimenting with QC for settlement optimization, he told us this: “I think the challenge in financial services will be in scaling up that number of qubits, but from our perspective, the important thing is to be what is called quantum ready.”
A bank doesn’t have to dedicate a massive amount of resources to quantum exploration—a couple highly qualified experts with PhDs can, at this point, do the trick
And back to that quantum computing article we published last week. It wasn’t all naysayers.
“From the creation of the first transistor to its integration onto a wafer at a reasonable size took about 10 years, and then it was another 10 to 15 years or so before the first commercial microchip became available, with which you could do something,” said Franco Severini, chief technology officer at multinational Japanese IT services provider Fujitsu. “We’re looking at the same kind of timescale. Just because something is far out, doesn’t mean it’s not worth pursuing.”
Fabio Sanches, director of quantum computing at the US Federal Reserve, said that in the mid-2010s, “unrealistically optimistic expectations” were given, perhaps similar to the irrational exuberance around blockchain that was unfolding around the same time. That last piece about blockchain is my words, but I’d argue that while both can technically be described as hammers looking for a nail, quantum computing is—at this stage—essentially a very important science experiment, while in the world of distributed ledger technology, people selling commercially ready snake oil are far more common.
It’s also important to remember that a bank doesn’t have to dedicate a massive amount of resources to quantum exploration—a couple highly qualified (and, yes, well paid) experts with PhDs that report directly to the chief technology and/or innovation officer can, at this point, do the trick, according to Eliot’s sources.
Additionally, the vendor community—including Fujitsu, IBM, Google, Microsoft, along with niche specialists like D-Wave Systems and Rigetti Computing—are willing to help at a reasonable cost, as they want test subjects to help improve their own understanding of quantum computing and proofs-of-concept for finance. Also, just like how IBM, Google, Microsoft and Amazon are fighting to win over cloud converts, those Big Tech companies that can develop deep ties to the largest banks through quantum PoCs today, will also likely be at the front of the line when the day finally does come when commercialized quantum computers are available to the banking public.
Tough to say?
It’s November, so that means that technologists are fighting for budget to build cutting-edge things like generative AI, causal AI and other forms of machine learning, and (ugh…fine) even things in the world of crypto. Others are laser-focused on day-to-day data challenges, handling the fees around those data needs, and addressing regulatory requirements. Oh, and there are staffing concerns around all those initiatives.
So how does one advocate for something that will offer more failure than incremental success over the next three years (and likely much longer)? Once again, are you better off today for having (or not having) experts in the fields of ML and NLP as you’re trying to develop your genAI strategy for the future?
Perhaps that’s too simplistic a question to ask considering that budgets are finite, not infinite…I guess similar to classical computers and quantum computers.
The image accompanying this column is “The Angel Appearing to Zacharias” by William Blake, courtesy of The Met’s open-access program.
Only users who have a paid subscription or are part of a corporate subscription are able to print or copy content.
To access these options, along with all other subscription benefits, please contact info@waterstechnology.com or view our subscription options here: http://subscriptions.waterstechnology.com/subscribe
You are currently unable to print this content. Please contact info@waterstechnology.com to find out more.
You are currently unable to copy this content. Please contact info@waterstechnology.com to find out more.
Copyright Infopro Digital Limited. All rights reserved.
As outlined in our terms and conditions, https://www.infopro-digital.com/terms-and-conditions/subscriptions/ (point 2.4), printing is limited to a single copy.
If you would like to purchase additional rights please email info@waterstechnology.com
Copyright Infopro Digital Limited. All rights reserved.
You may share this content using our article tools. As outlined in our terms and conditions, https://www.infopro-digital.com/terms-and-conditions/subscriptions/ (clause 2.4), an Authorised User may only make one copy of the materials for their own personal use. You must also comply with the restrictions in clause 2.5.
If you would like to purchase additional rights please email info@waterstechnology.com
More on Emerging Technologies
This Week: Startup Skyfire launches payment network for AI agents; State Street; SteelEye and more
A summary of the latest financial technology news.
Waters Wavelength Podcast: Standard Chartered’s Brian O’Neill
Brian O’Neill from Standard Chartered joins the podcast to discuss cloud strategy, costs, and resiliency.
SS&C builds data mesh to unite acquired platforms
The vendor is using GenAI and APIs as part of the ongoing project.
Chevron’s absence leaves questions for elusive AI regulation in US
The US Supreme Court’s decision to overturn the Chevron deference presents unique considerations for potential AI rules.
Reading the bones: Citi, BNY, Morgan Stanley invest in AI, alt data, & private markets
Investment arms at large US banks are taken with emerging technologies such as generative AI, alternative and unstructured data, and private markets as they look to partner with, acquire, and invest in leading startups.
Startup helps buy-side firms retain ‘control’ over analytics
ExeQution Analytics provides a structured and flexible analytics framework based on the q programming language that can be integrated with kdb+ platforms.
The IMD Wrap: With Bloomberg’s headset app, you’ll never look at data the same way again
Max recently wrote about new developments being added to Bloomberg Pro for Vision. Today he gives a more personal perspective on the new technology.
LSEG unveils Workspace Teams, other products of Microsoft deal
The exchange revealed new developments in the ongoing Workspace/Teams collaboration as it works with Big Tech to improve trader workflows.