BoE model risk rule may drive real-time monitoring of AI
New rule requires banks to rerun performance tests on models that recalibrate dynamically.
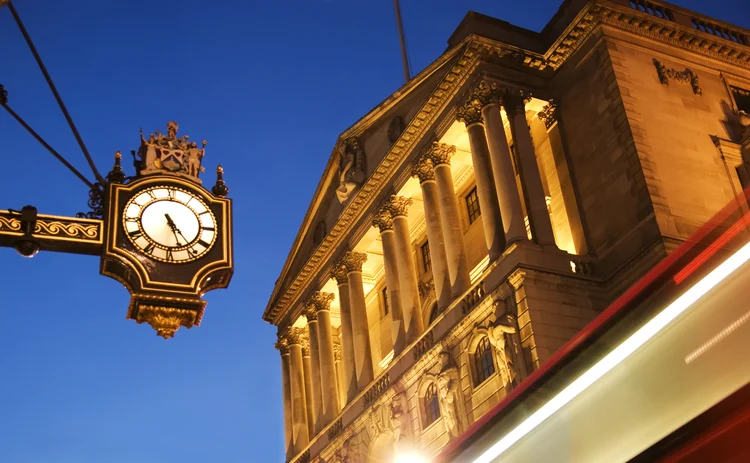
New rules from the Bank of England (BoE) on model risk management could push banks towards much more intensive monitoring of artificial intelligence (AI) and other algorithms that dynamically recalibrate, experts say.
“Algos and AI continuously operate, so it’s necessary to ensure they behave as expected, and that puts lots of pressure on us,” says Karolos Korkas, head of algorithmic trading model risk at Nomura. “We might just need to move into more real-time monitoring.”
The BoE’s Prudential Regulation Authority published its final supervisory statement, SS1/23, in May this year, following a consultation in June 2022. The PRA noted respondents to the original consultation had expressed concern that the requirement to rerun performance tests on models with “dynamic calibration would be computationally intensive and may not be practical across all model types, e.g., algorithmic trading and other pricing models”. But the regulator decided to stick to its guns in the final version of the rules.
“The PRA expects firms to manage the risk that a series of small (immaterial) changes due to recalibrations could accumulate, when uncontrolled or unchecked, into a material change in the model output over time without it being tested,” the PRA concluded.
The sheer volume of data that drives some of these models would make it, if not computationally intensive, then certainly effort intensive to try to figure out how you rewind back to earlier periods
Rod Hardcastle, Deloitte
These are not the first regulatory requirements around models, but, before SS1/23, PRA guidance has been piecemeal and incorporated into other parts of the risk taxonomy. The new rule offers an overarching model risk guidance, and places clear management responsibility on both model users and the board, integrating the requirements into the UK senior managers’ regime.
“How do we explain the models, how do we understand the models, how do we collect the data, how do we monitor them?” says Korkas. “We need to be very cautious about how we scale up the whole AI technology.”
Crucially, the rule also provides a new, very broad definition of models: “The definition of a model includes input data that are quantitative and/or qualitative in nature or expert judgement-based, and output that are quantitative or qualitative.”
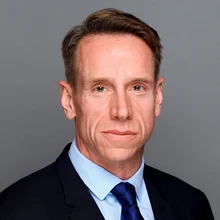
Bankers believe this will vastly increase the model inventory for many banks, stretching validation teams and potentially forcing a cull of models if their use case is inadequate.
The adoption of AI throughout banks in a wide range of functions in recent years presents a further challenge. Whereas models for calculating regulatory capital have already been subject to supervisory validation processes for many years, some AI activity has not been explicitly covered by any existing regulation. Sources mention programs to assist retail loan underwriting decisions and to help human resources departments sift the CVs of job applicants as areas where AI has faced less internal and external scrutiny.
“AI models might have been sold to people in the organizations who aren’t experienced model owners, and they might not even realise that they’re buying an AI product,” says Keith Garbutt, an independent consultant, and former head of model validation at HSBC.
Rewriting history
The fear around implementing the “automatic recalculation of performance test results” for AI stems from the fact that the technology makes decisions via many small changes in the model, which can happen without input from the model owner.
Existing prudential models that are operated and calibrated by risk managers tend to be reviewed every quarter and validated only once or twice a year. An AI model, however, changes independently of the model operator. For example, if the model is used for anti-money laundering transaction monitoring and trained on unsupervised data, it will teach itself how to distinguish suspicious or normal transactions.
The changes in its behavior and decision-making might not be triggered by events such as new regulations, but rather by its own success at flagging suspicious transactions. In this specific example, the model will also be running on a very large amount of data. The more data it ingests, the more likely it is to change its logic and decision-making.
Algos and AI continuously operate, so it’s necessary to ensure they behave as expected, and that puts lots of pressure on us. We might just need to move into more real-time monitoring
Karolos Korkas, Nomura
Performance tests would have to track the history of each of those decisions, and the implications for overall model accuracy. The longer the time lag between each test, the more potential model changes will have taken place, which means the standard quarterly monitoring may not be enough.
“The sheer volume of data that drives some of these models would make it, if not computationally intensive, then certainly effort intensive to try to figure out how you rewind back to earlier periods,” says Rod Hardcastle, head of credit risk regulation at Deloitte, and a former head of prudential risk at the Bank of Ireland.
All of this points to much more frequent monitoring of AI to track any automated changes in the model. For more material models, that might mean a kill switch where appropriate and applicable. Such is the case for dynamically recalculating algo trading models, says Andrew Mackay, former head of model risk at Deutsche Bank.
Nadia Bouzebra, head of model risk at Close Brothers, explains that some models can be tested once at the beginning of their lifecycle, and then an easy retest can be done at a set periodicity. But some need to be monitored every single day.
Benchmarks and key performance indicators are used to ensure models don’t stray from their expected outputs, says Bouzebra. This is another part of governance that will have to be firmly enforced under the new PRA rules, with contingency plans for models that cannot be adequately monitored or fail the tests.
“If you want to use those models, there are things you need to do to ensure they are working properly,” says David Asermely, global head of risk management at technology vendor SAS. “If you are not able to do those things, then maybe you need to use something else—do you have a bunch of challenger models that can replace it?”
Saving graces
Several elements of the PRA rule will make it easier for firms to comply. First, SS1/23 will not apply to banks on the PRA’s simpler firms regime—for which the BoE intends to develop similar rules at a later date. For the banks covered by SS1/23, the compliance date is May 2024.
Second, the requirements also include a tiering process, where the required rigor of model risk management depends on the materiality of risk that each model represents for the bank. Realistically, bankers say the highest tiers are likely to comprise models that are already subject to significant scrutiny, such as those used for calculating regulatory capital.
“Will it be challenging? Yes, but it’s not a new problem,” says Mackay.
For global banks, there is a further source of reassurance: the PRA guidance has some elements in common with US model risk rules set out more than a decade ago by the Federal Reserve and the Office of the Comptroller of Currency in SR 11-7. However, the US rules did not cover AI, since its adoption was much less widespread and has accelerated sharply in recent years.
“If you’ve been through SR 11-7, you can’t just assume you will be compliant with the [PRA] principles,” says Hardcastle.
Moreover, Mackay notes that some non-US banks still struggle to comply with SR11-7 even today. The model risk managers speaking to WatersTechnology’s sibling publication Risk.net give estimates of anywhere between two and five years for banks to bring their processes up to a level that is likely to pass muster with the BoE, depending on how strict the regulator chooses to be.
“The key factor for me is the tone from the top, whether the boards—management and supervisory—can understand the benefits of compliance and costs of non-compliance,” says Mackay.
Further reading
Only users who have a paid subscription or are part of a corporate subscription are able to print or copy content.
To access these options, along with all other subscription benefits, please contact info@waterstechnology.com or view our subscription options here: http://subscriptions.waterstechnology.com/subscribe
You are currently unable to print this content. Please contact info@waterstechnology.com to find out more.
You are currently unable to copy this content. Please contact info@waterstechnology.com to find out more.
Copyright Infopro Digital Limited. All rights reserved.
As outlined in our terms and conditions, https://www.infopro-digital.com/terms-and-conditions/subscriptions/ (point 2.4), printing is limited to a single copy.
If you would like to purchase additional rights please email info@waterstechnology.com
Copyright Infopro Digital Limited. All rights reserved.
You may share this content using our article tools. As outlined in our terms and conditions, https://www.infopro-digital.com/terms-and-conditions/subscriptions/ (clause 2.4), an Authorised User may only make one copy of the materials for their own personal use. You must also comply with the restrictions in clause 2.5.
If you would like to purchase additional rights please email info@waterstechnology.com
More on Emerging Technologies
This Week: Startup Skyfire launches payment network for AI agents; State Street; SteelEye and more
A summary of the latest financial technology news.
Waters Wavelength Podcast: Standard Chartered’s Brian O’Neill
Brian O’Neill from Standard Chartered joins the podcast to discuss cloud strategy, costs, and resiliency.
SS&C builds data mesh to unite acquired platforms
The vendor is using GenAI and APIs as part of the ongoing project.
Chevron’s absence leaves questions for elusive AI regulation in US
The US Supreme Court’s decision to overturn the Chevron deference presents unique considerations for potential AI rules.
Reading the bones: Citi, BNY, Morgan Stanley invest in AI, alt data, & private markets
Investment arms at large US banks are taken with emerging technologies such as generative AI, alternative and unstructured data, and private markets as they look to partner with, acquire, and invest in leading startups.
Startup helps buy-side firms retain ‘control’ over analytics
ExeQution Analytics provides a structured and flexible analytics framework based on the q programming language that can be integrated with kdb+ platforms.
The IMD Wrap: With Bloomberg’s headset app, you’ll never look at data the same way again
Max recently wrote about new developments being added to Bloomberg Pro for Vision. Today he gives a more personal perspective on the new technology.
LSEG unveils Workspace Teams, other products of Microsoft deal
The exchange revealed new developments in the ongoing Workspace/Teams collaboration as it works with Big Tech to improve trader workflows.