Waters Wrap: The path to generative AI is paved with solid data practices
While large language models are likely to proliferate, those that can develop a solid data infrastructure of taxonomies, ontologies, data sourcing, mapping and lineage will be the ultimate winners, Anthony says.
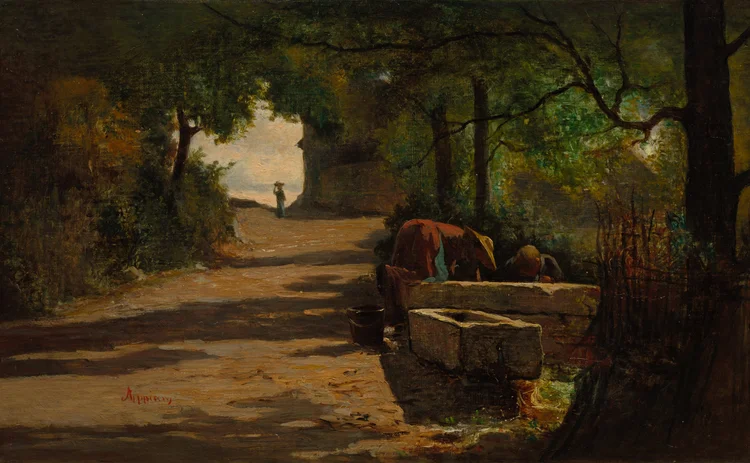
In the highly regulated capital markets, there’s a dichotomy between things you “want to do” and things you “need to do”. Banks and asset managers want to deploy high-powered, machine-learning-driven analytics tools, but they need to solve ever-greater regulatory reporting needs. But does it have to be this way? Is there a way to bridge the gap?
(I hope you’ll follow along with me as this is a bit of a think piece. And I’d love feedback to see how I can improve this theory.)
First, let’s look at the state of reg reporting in the capital markets. While 2008 led to a global regulatory overhaul, that avalanche hasn’t yet stopped rolling. At the end of this month, the first compulsory periodic reports under the European Union’s Sustainable Finance Disclosure Regulation are due. As firms are coming to learn, SFDR is helping to highlight the challenge of sourcing and mapping ESG data.
In a similar vein, there’s also the long-awaited Fundamental Review of the Trading Book. While the deadline for FRTB won’t hit until January 1, 2025, trading firms are starting to realize that they’re facing the mother of all data management problems as they attempt to (once again) map and source incredibly granular data—and by some estimates, a single bank might have to run 1.2 million calculations under the internal model approach for FRTB compliance.
The issue is that banks only do the bare minimum that they legally have to, and that’s not how you make an investment. They need to make an investment for an unknown future.
A head of software engineering
These are just two needles in the haystack of regulatory reporting requirements facing banks and asset managers, but they clearly show that even though the Dodd–Frank Act was enacted in 2010 and Mifid II was approved in 2014, industry participants are still struggling with the idea of data basics: sourcing, mapping, lineage and quality.
Recently I interviewed Neema Raphael, Goldman Sachs’ head of data engineering and its chief data officer. We spoke about the difficulties of untangling systems to improve data quality and lineage. “The big thing is [to] go into those systems without having to do heart surgery; rather, do higher-level skin surgery … That’s the biggest lesson we’ve learned,” he said, describing the decade-long, incremental process of changing the bank's data management infrastructure.
These projects take intestinal fortitude—which is something that few banks have, says the head of software engineering at a large retail company who formerly did consulting work for financial services firms.
“The issue is that banks only do the bare minimum that they legally have to, and that’s not how you make an investment. They need to make an investment for an unknown future. Unless they can find a way to justify that in the present, they’re going to keep cobbling together all these vendor solutions just to get by on skeleton-crew staffing,” they say. “It’s tough to convince people that there’s probably going to be future value there, but, ‘What does that look like? We don’t know.’”
Ambitious data management projects require companies to burn a certain percentage of budget on creating internal standards, taxonomies and ontologies that will—hopefully—be useful later…perhaps after those projects' champions have left the company.
“It’s so hard to define ROI on these projects,” they say. “It’s human management of large organizations—that will always be at the heart of the issue.”
But the engineer does believe that if firms want to take advantage of sophisticated machine-learning-driven analytics platforms, they need to invest in their data management processes. “The future of analytics will require firms to get the basics right.”
The next generat(ive)
Which brings us to the hottest topics sweeping the land: OpenAI’s ChatGPT, the (mainstream) advent of large language models (LLMs), and generative machine learning. (I hate the term generative AI…it’s machine learning, not the broad umbrella of artificial intelligence.)
No matter what anyone wants you to believe, it is still very early days when it comes to LLMs, especially in the regulated world of finance. On a daily basis, I receive some sort of pitch about a ChatGPT-like solution that will change the capital markets forever. LLMs are having their NFT or DLT moment right now, and people need to be wary of charlatans.
But unlike DLT, LLMs are not hammers looking for nails. There’s a fundamental shift underway in the world of financial technology. Cloud is the backbone of modern day tech development. As more data is becoming available (thanks to cloud), APIs are becoming the preferred way to deliver information and services. And to help speed up dev projects, open-source tools are proliferating inside banks. The aim of these developments is to provide better context around a deluge of data so users better understand what it is that they’re looking at so as to make better informed decisions.
Context and analytics…that’s the name of the game. And while cloud, APIs and open-source provide the ecosystem, machine learning does the heavy lifting, and large language models are the next evolution of machine learning.
LLMs are really good at taking a lot of data in and deriving structure from it. The more high-quality/well-structured data you feed into these models, the better the outcomes. So, improving the quality of data (as well as developing robust pre-processing and tuning steps) will separate the winners from the losers. The alpha will be in taking a powerful core, and then extending it with domain-specific helpers for domain-specific tasks, says a machine-learning engineer at a trading technology vendor.
Bloomberg was smart—they took the architecture of the model and they trained it uniquely on proprietary data and then tuned the model on proprietary data for proprietary tasks
A second engineer
As an example, consider Bloomberg and its development of BloombergGPT. (Note: GPT stands for generative pre-trained transformer.) The proprietary LLM is still in production, but the hope is it will help to assist—and provide more depth to—the Terminal’s sentiment analysis, named-entity recognition (NER), news classification, charting, and question-answering capabilities, among other functions.
The engineer, who recently read the research paper published by Bloomberg on the subject, says that the paper has “a lot of cool use-cases”, and they think that others will soon follow in the company’s footsteps.
“Bloomberg was smart—they took the architecture of the model and they trained it uniquely on proprietary data and then tuned the model on proprietary data for proprietary tasks,” they say.
They were able to do this because they’ve created company-wide taxonomies (a hierarchical tier of data) and ontologies (which are concerned with the relationships between those hierarchies). Because Bloomberg had a data foundation in place for its proprietary data, it was more easily able—relatively speaking…the project began in 2020—to build out its own proprietary tasks, says the source.
“You can take the current LLM architecture, get it a good dataset, prepare the dataset appropriately, find a smaller domain of tasks that you want solve with it, and then tune the model accordingly,” they say. “That’s how most people are going to get results out of LLMs. Otherwise, there’s not much differentiation between that and paying 50 grand a month to use OpenAI’s APIs.”
But the key is having a data foundation in place. To me, that’s the bridge between putting in the hard work of data sourcing, mapping and lineage for regulatory reporting needs, and crossing over to high-end analytics.
With CEOs and other C-suiters asking about ChatGPT, perhaps this is the moment to redefine the ROI on these painful data quality projects.
Think I’m making a leap? Perhaps a bridge too far? Let me know: anthony.malakian@infopro-digital.com.
The image accompanying this column is “Well at the Side of a Road” by Adolphe Appian, courtesy of the Cleveland Museum of Art’s open-access program.
Only users who have a paid subscription or are part of a corporate subscription are able to print or copy content.
To access these options, along with all other subscription benefits, please contact info@waterstechnology.com or view our subscription options here: http://subscriptions.waterstechnology.com/subscribe
You are currently unable to print this content. Please contact info@waterstechnology.com to find out more.
You are currently unable to copy this content. Please contact info@waterstechnology.com to find out more.
Copyright Infopro Digital Limited. All rights reserved.
As outlined in our terms and conditions, https://www.infopro-digital.com/terms-and-conditions/subscriptions/ (point 2.4), printing is limited to a single copy.
If you would like to purchase additional rights please email info@waterstechnology.com
Copyright Infopro Digital Limited. All rights reserved.
You may share this content using our article tools. As outlined in our terms and conditions, https://www.infopro-digital.com/terms-and-conditions/subscriptions/ (clause 2.4), an Authorised User may only make one copy of the materials for their own personal use. You must also comply with the restrictions in clause 2.5.
If you would like to purchase additional rights please email info@waterstechnology.com
More on Emerging Technologies
This Week: Startup Skyfire launches payment network for AI agents; State Street; SteelEye and more
A summary of the latest financial technology news.
Waters Wavelength Podcast: Standard Chartered’s Brian O’Neill
Brian O’Neill from Standard Chartered joins the podcast to discuss cloud strategy, costs, and resiliency.
SS&C builds data mesh to unite acquired platforms
The vendor is using GenAI and APIs as part of the ongoing project.
Chevron’s absence leaves questions for elusive AI regulation in US
The US Supreme Court’s decision to overturn the Chevron deference presents unique considerations for potential AI rules.
Reading the bones: Citi, BNY, Morgan Stanley invest in AI, alt data, & private markets
Investment arms at large US banks are taken with emerging technologies such as generative AI, alternative and unstructured data, and private markets as they look to partner with, acquire, and invest in leading startups.
Startup helps buy-side firms retain ‘control’ over analytics
ExeQution Analytics provides a structured and flexible analytics framework based on the q programming language that can be integrated with kdb+ platforms.
The IMD Wrap: With Bloomberg’s headset app, you’ll never look at data the same way again
Max recently wrote about new developments being added to Bloomberg Pro for Vision. Today he gives a more personal perspective on the new technology.
LSEG unveils Workspace Teams, other products of Microsoft deal
The exchange revealed new developments in the ongoing Workspace/Teams collaboration as it works with Big Tech to improve trader workflows.