GenAI and HFT: A competitive edge?
Timing is critical in the capital markets. IBM researchers say genAI’s predictive abilities in combination with AI/ML models can translate into profitable HFT opportunities.
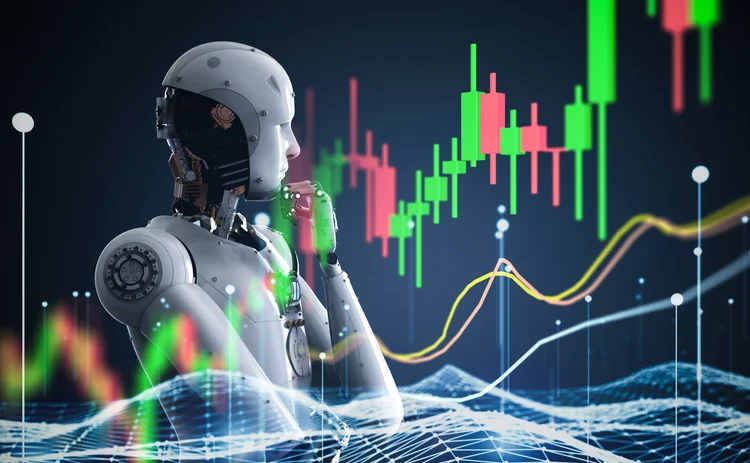
Need to know
Anjanita Das, Director AI Practice, Cognizant Technology Solutions
Kenneth Schoff, Principle Partner-Sustainability, IBM
Raja Basu, Business Architect (Financial Markets), IBM
Generative AI, rooted in predictive recommendation capabilities, operates on the foundations of deep learning foundational models, which use large language models (LLMs) to delve into intricate patterns and relationships within complex data. These models can be fine-tuned and contextualized to deliver specific tasks, demonstrating an impressive ability to foresee outcomes.
For instance, the advanced language model GPT4 showcases its predictive prowess by anticipating the next word in a sentence, allowing it to construct coherent and meaningful responses to questions. This predictive capacity extends beyond language applications, and in the realm of trading, one can envision the development of a model adept at forecasting market behavior in combination with traditional AI/ML models.
In the dynamic financial markets, where timing is critical, the predictive abilities of genAI in combination with AI/ML models are particularly significant. In the context of high-frequency trading (HFT), even predictions spanning mere seconds can translate into profitable opportunities. These models, equipped with the capability to analyze and interpret market trends swiftly, offer traders a competitive edge. In this article, we will explore some of the potential impacts of generative AI and a form of geofencing on the financial markets in the context of high-frequency institutional trading.
The combination of traditional AI/ML-based predictive modeling and genAI can help in trading scenarios that can lead to effective decision-making and the execution of trades within the narrow windows of opportunity presented by rapidly evolving market conditions. However, there are inherent challenges and ethical considerations associated with leveraging genAI in trading. The reliance on genAI algorithms introduces complexities, such as the potential for unforeseen market anomalies and the need for constant model adaptation to evolving market dynamics. And questions surrounding the ethical use of AI in financial systems, particularly regarding market manipulation and data security, underscore the importance of responsible and transparent deployment of these technologies in the financial domain.
To make a prediction, we need a triggering event. The everyday usage of the term geofencing refers to the detection of the location of a person or object based on GPS, radio-frequency identification (RFID), or other means to trigger a response. For the markets, we are not talking about phone apps that detect when you are about to enter store A, triggering a text message with incentives to redirect you to store B. However, the concept can be applied to transactions moving between markets.
Consider arbitrage. An institution’s genAI system monitors the markets and sees a price and regulations for one instrument in one market (New York, for example) that differs from the price in another market (say, London). This triggers simultaneous trades to buy at the lower price and sell at the higher price. If the transactions are substantial, this will affect the market. The predictive algorithms of the AI system may be able to predict how prices will change for that instrument and other related instruments, and make additional trades based on the changes.
One concern about automated trading based on algorithms is the potential for large transactions to trigger widespread negative consequences. A generative AI system in combination with AI/ML-based predictive algorithms could predict these consequences and act to keep the market stable without interrupting normal market operations.
Finally, we need to consider the quality of the data used to train the foundational models that are used to underpin these financial applications. Foundation models are trained with terabytes, perhaps petabytes, of general knowledge to make them adaptable to many applications.
In earlier AI efforts in the 1990s, it was recognized that effective AI systems required broad general knowledge, and we now have the hardware and software technology to address this. The training data in these foundational models must be curated to remove inappropriate bias and prejudices to assure fairness in system recommendations. This might include reasoning to prevent significant market upheavals in the interest of a single participant’s gains. Generative AI is beneficial in creating synthetic data for better prediction capabilities.
These applications of AI start with a general foundational model, such as those available from IBM, Google, AWS, Microsoft Azure, and others. These are tuned to address a particular task—in this case, financial market activities, using data tagged for the specific application. This focuses the learning process on the goals of the application. This data must also be appropriately curated, as in the case to contextualize the foundation models.
The role of genAI in algo trading
Automated trading choices are made using complicated mathematical models and historical data in algorithmic trading, also known as quantitative or high-frequency trading. There are numerous significant ways in which genAI can improve algorithmic trading.
- Predictive analytics: GenAI can examine massive datasets, such as market tendencies, news sentiment, and previous price changes, to spot patterns and forecast the market’s direction with the help of AI/ML algorithms. Informed trading decisions are made possible by these forecasts.
- Management of risk: GenAI algorithms can analyze market risk and adapt trading strategies in real time to reduce exposure to losses. Capital preservation in times of market uncertainty requires this risk management skill.
- Insights in real time: Traders can get up-to-the-minute information from the market thanks to genAI’s real-time analysis.
- Efficiency: Trades powered by AI-enabled automated trading systems are executed quickly and efficiently without the interference of human lag or emotional bias.
- Portfolio optimization: Asset selection is based on risk appetite, investment objectives and market conditions, and genAI with the help of deep learning optimization algorithms (neural network-based) can aid in optimizing investment portfolios.
Here is what the logical genAI-driven process looks like:
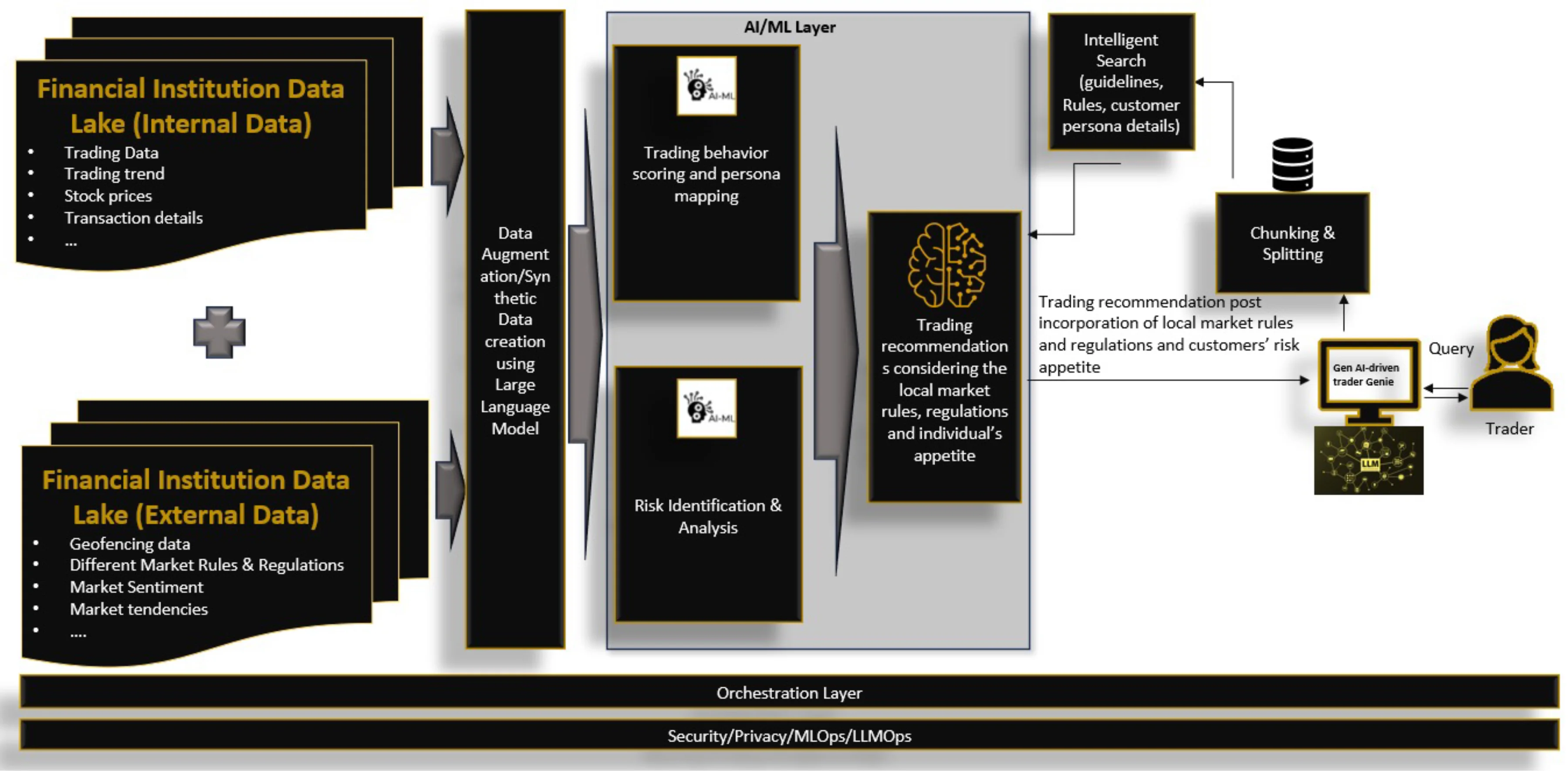
Using generative AI models for HFT automation offers an opportunity to improve market liquidity with enhanced control over the results to avoid adverse market consequences. Since institutional trading represents the highest trade volumes in the markets and these institutions are most likely to have the wherewithal to build such systems, we might predict improved stability, liquidity, and fairness in the markets for the future.
Anjanita Das, Director AI Practice, Cognizant Technology Solutions
Kenneth Schoff, Principle Partner-Sustainability, IBM
Raja Basu, Business Architect (Financial Markets), IBM
Only users who have a paid subscription or are part of a corporate subscription are able to print or copy content.
To access these options, along with all other subscription benefits, please contact info@waterstechnology.com or view our subscription options here: http://subscriptions.waterstechnology.com/subscribe
You are currently unable to print this content. Please contact info@waterstechnology.com to find out more.
You are currently unable to copy this content. Please contact info@waterstechnology.com to find out more.
Copyright Infopro Digital Limited. All rights reserved.
As outlined in our terms and conditions, https://www.infopro-digital.com/terms-and-conditions/subscriptions/ (point 2.4), printing is limited to a single copy.
If you would like to purchase additional rights please email info@waterstechnology.com
Copyright Infopro Digital Limited. All rights reserved.
You may share this content using our article tools. As outlined in our terms and conditions, https://www.infopro-digital.com/terms-and-conditions/subscriptions/ (clause 2.4), an Authorised User may only make one copy of the materials for their own personal use. You must also comply with the restrictions in clause 2.5.
If you would like to purchase additional rights please email info@waterstechnology.com
More on Emerging Technologies
This Week: Startup Skyfire launches payment network for AI agents; State Street; SteelEye and more
A summary of the latest financial technology news.
Waters Wavelength Podcast: Standard Chartered’s Brian O’Neill
Brian O’Neill from Standard Chartered joins the podcast to discuss cloud strategy, costs, and resiliency.
SS&C builds data mesh to unite acquired platforms
The vendor is using GenAI and APIs as part of the ongoing project.
Chevron’s absence leaves questions for elusive AI regulation in US
The US Supreme Court’s decision to overturn the Chevron deference presents unique considerations for potential AI rules.
Reading the bones: Citi, BNY, Morgan Stanley invest in AI, alt data, & private markets
Investment arms at large US banks are taken with emerging technologies such as generative AI, alternative and unstructured data, and private markets as they look to partner with, acquire, and invest in leading startups.
Startup helps buy-side firms retain ‘control’ over analytics
ExeQution Analytics provides a structured and flexible analytics framework based on the q programming language that can be integrated with kdb+ platforms.
The IMD Wrap: With Bloomberg’s headset app, you’ll never look at data the same way again
Max recently wrote about new developments being added to Bloomberg Pro for Vision. Today he gives a more personal perspective on the new technology.
LSEG unveils Workspace Teams, other products of Microsoft deal
The exchange revealed new developments in the ongoing Workspace/Teams collaboration as it works with Big Tech to improve trader workflows.