The IMD Wrap: Quality drivers—the sticks and carrots accelerating the data quality race
Like a Formula One Grand Prix, data management is a race that can be won or lost. And just as each race is part of a larger F1 championship that pays large sums of TV money to the winning team, winning or losing one race can contribute to winning or losing an endgame with much more at stake.
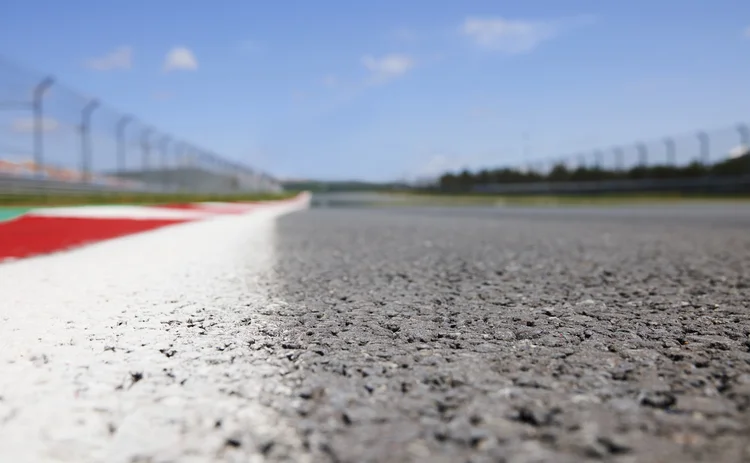
Anyone who watched this weekend’s Hungarian Formula One Grand Prix may have been shocked to see and hear some of the supposedly best race car drivers in the world whining over their team radios like petulant children. Max Verstappen, for example, admittedly an incredibly talented driver, managed to blame his strategists, his car, his long-suffering race engineer Gianpiero “GP” Lambiase, and other drivers—though notably not himself for staying up late the night before playing simulation racing games online—before his team boss basically told him to stop the “childish” back-and-forth over public broadcast.
But these aren’t the kind of quality (or not) drivers I plan to address in this column. Instead, I’m talking about the drivers of data quality—though I may throw in a smattering of F1 references—which, in today’s capital markets, have multiple sources.
In the past, the pursuit of data quality had fairly simple motivations: bad data—be it inaccurate, inconsistent, or not timely—leads to bad decisions, bad trades and lost money.
In F1 parlance, imagine deciding a race strategy without knowing your car’s top speed or lap times, and the effect of a lightening fuel load and tire degradation. Yes, it’s a lot to compute; yes, it’s hard work, but knowing those figures and having confidence in their accuracy is table stakes.
But planning a strategy for a race is one thing; data strategy is quite another. While a race has a set number of laps from start to finish, data strategy is a forever-moving target.
And just when you think you’re on the final lap, a new business need or a new regulation comes along requiring higher standards and more data.
Or it could be requiring the completion of regulatory reports that demand the utmost accuracy, or new alternative datasets that might deliver a source of alpha for traders or algorithms, but which have never been thought of as a data input before, so haven’t had the same rigorous standards of data quality applied to them. And suddenly, what was good enough before is no longer good enough.
While a race has a set number of laps from start to finish, data strategy is a forever-moving target
At this point, let me interrupt myself to ask two questions: First, can data ever be good enough? And second, how do you measure data quality? How do you put a numerical value on it? And, just as you need to be sure the data is accurate, how can you be sure of your own assumptions and methodologies when arriving at that figure?
Anyway, moving on: The most urgent respective carrot and stick of today’s renewed focus on data quality are the industry’s embrace of artificial intelligence and generative AI applications, and more stringent requirements from regulators.
In the case of AI, the reason is simple: If you expect an AI to deliver accurate answers, you first need accurate underlying data, and it needs to be consistent. And that accuracy and consistency needs to be the same across an enterprise: you cannot have two people in different areas of a bank or asset manager asking the same question and getting different results. That’s a recipe for disaster.
For the most part, these early AI experiments are not incredibly complex (says the person who couldn’t tell his AI from his elbow).
Broadly speaking, they are designed to provide better context and analytics around a rising tide of data, being able to combine Big Data analysis with unstructured content, and deliver responses to queries on volumes of information beyond the capacity of the human brain, but with an element of human-like reasoning.
Or, to cut a long story short, they’re supposed to help cut long stories short, cut through the noise, and be more productive by finding the right data faster.
Last week, my colleague Eliot Raman Jones profiled a recent project by SigTech, a buy-side portfolio analytics tech spinoff from European asset manager Brevan Howard. Since being spun out of the firm in 2019, the wizards at SigTech have been experimenting with Magic to answer financial data challenges—not illusions or tricks, but Multi-Agent Generative Investment Co-Pilots, which combine GenAI and large language models to retrieve insights within minutes that would take quants or researchers hours to uncover.
Magic uses a range of LLMs and different programs called agents that have been trained on different datasets—effectively creating a committee of virtual experts to analyze specific datasets.
Of course, if you’ve done any experimentation with AI, you’ll know it doesn’t always get things right the first (and often the second or third) time, and there’s a lot of time invested in training these tools to deliver quality answers. And while this may get better over time, there’s a lot of double-checking that needs to be done in the meantime. But if the underlying data isn’t accurate, even if the AI performs its task correctly, you’ll get inaccurate results.
SigTech, for example, is so confident in the quality of its underlying data, that the vendor uses it as the source for its AI agents, making API calls to its dataset to minimize the potential for AI-generated errors, dubbed “hallucinations.”
To be sure, GenAI has the potential to do much more than this. But, between firms not wanting to move too quickly and risk something going wrong (or even just the perception of being too quick to embrace technology that’s often likened to The Terminator and The Matrix movies), and regulators deciding what applications are suitable or not for AI, many use cases may remain off limits for the foreseeable future.
And speaking of regulators, there are a slew of new regulations or amendments planned for next year. From January 1, 2025, the Fundamental Review of the Trading Book capital rules take effect in Europe, as part of Basel III. The same date also marks the beginning of ESG-related rules on the Hong Kong Stock Exchange, with more in the works from other jurisdictions, such as the EU’s Corporate Sustainability Due Diligence Directive. At the same time, some firms are still struggling with expanded trade reporting requirements in Europe and the US.
And while for these functions, the buck may still stop with a firm’s chief compliance officer or chief risk officer, the bulk of the grunt work will likely fall under the remit of the chief data officer—or chief data and analytics officer—because it’s their job to organize and unify data from across an organization so that everyone who needs it, from the CEO to those in compliance and risk, can have a full and unobstructed view of the business, its revenues, risks, and exposures, from the bottom to the top.
After all, most reporting issues are, for all intents and purposes, data issues. Do you have the data to demonstrate that you are compliant? How much of something do you have? What did you trade, how much, when, and why? Do you have enough money in one place to cover what you’re doing in another? Have you overexposed yourself beyond your risk limits? Do you even know what your exposure and your limits are?
Much like the driver that is AI, achieving this requires accurate, universal, and consistent data that is also readily accessible to those who need it. And while in the past enterprise data management initiatives may have seemed like a chore to those outside of data management functions, now the carrot of AI and the stick of regulation are highlighting the importance of these programs and related developments such as data meshes.
Ironically, in the future, AI may be able to assist with making some data management functions—such as creating end-user profiles and matching datasets to those profiles based on user requirements, spotting areas of duplicative use of data, or identifying comparable data sources to replace incumbent datasets—easier. In the short term, though, the demands of serving up data for AI and regulatory functions are making data management much harder now than in the past.
AI may be a technology issue, and regulation may be a compliance issue, but ultimately, they’re both data issues. And you can’t be sure of your ability to do either right without first getting your data right.
Have thoughts? Share them with me at max.bowie@infopro-digital.com.
Further reading
Only users who have a paid subscription or are part of a corporate subscription are able to print or copy content.
To access these options, along with all other subscription benefits, please contact info@waterstechnology.com or view our subscription options here: http://subscriptions.waterstechnology.com/subscribe
You are currently unable to print this content. Please contact info@waterstechnology.com to find out more.
You are currently unable to copy this content. Please contact info@waterstechnology.com to find out more.
Copyright Infopro Digital Limited. All rights reserved.
As outlined in our terms and conditions, https://www.infopro-digital.com/terms-and-conditions/subscriptions/ (point 2.4), printing is limited to a single copy.
If you would like to purchase additional rights please email info@waterstechnology.com
Copyright Infopro Digital Limited. All rights reserved.
You may share this content using our article tools. As outlined in our terms and conditions, https://www.infopro-digital.com/terms-and-conditions/subscriptions/ (clause 2.4), an Authorised User may only make one copy of the materials for their own personal use. You must also comply with the restrictions in clause 2.5.
If you would like to purchase additional rights please email info@waterstechnology.com
More on Data Management
New working group to create open framework for managing rising market data costs
Substantive Research is putting together a working group of market data-consuming firms with the aim of crafting quantitative metrics for market data cost avoidance.
Off-channel messaging (and regulators) still a massive headache for banks
Waters Wrap: Anthony wonders why US regulators are waging a war using fines, while European regulators have chosen a less draconian path.
Back to basics: Data management woes continue for the buy side
Data management platform Fencore helps investment managers resolve symptoms of not having a central data layer.
‘Feature, not a bug’: Bloomberg makes the case for Figi
Bloomberg created the Figi identifier, but ceded all its rights to the Object Management Group 10 years ago. Here, Bloomberg’s Richard Robinson and Steve Meizanis write to dispel what they believe to be misconceptions about Figi and the FDTA.
SS&C builds data mesh to unite acquired platforms
The vendor is using GenAI and APIs as part of the ongoing project.
Aussie asset managers struggle to meet ‘bank-like’ collateral, margin obligations
New margin and collateral requirements imposed by UMR and its regulator, Apra, are forcing buy-side firms to find tools to help.
Where have all the exchange platform providers gone?
The IMD Wrap: Running an exchange is a profitable business. The margins on market data sales alone can be staggering. And since every exchange needs a reliable and efficient exchange technology stack, Max asks why more vendors aren’t diving into this space.
Reading the bones: Citi, BNY, Morgan Stanley invest in AI, alt data, & private markets
Investment arms at large US banks are taken with emerging technologies such as generative AI, alternative and unstructured data, and private markets as they look to partner with, acquire, and invest in leading startups.