US Fed reveals its five use cases for generative AI
Internal sandbox used to assess viability and risks; coding and content generation on the agenda.
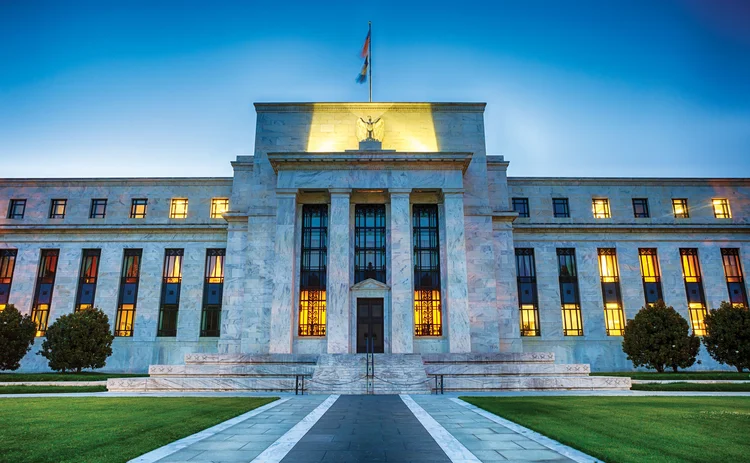
While commercial banks are actively exploring generative artificial intelligence (GenAI), the US Federal Reserve is also embracing the technology, identifying five primary internal use cases among a hundred or so experiments within its AI incubator, according to staff leading the project.
One of the standout uses of GenAI is its application in cleaning and processing terabytes of unstructured and multimodal datasets within the Fed system. This improves both accuracy and clarity of the datasets efficiently, which allows the agency’s financial analysts to concentrate on more advanced analyses instead of spending time on routine tasks.
“When you think about an analyst’s job at the Fed… you need to spend a good amount of time combing through all that data, getting into that data to do some analysis,” said Margaret Riley, senior vice-president at the payments unit of the Fed’s financial services division. “So when I think about the potential of GenAI, it is kind of [as] a super analyst.”
Riley was speaking at a conference in Chicago on June 25, together with the Fed’s chief innovation officer, Sunayna Tuteja. They discussed five key use cases the Fed has so far identified: data cleansing, customer engagement, content generation, translating legacy code and enhancing operational efficiency across the Fed’s large network of regional nodes. The two experts also set out how the agency has been managing the risk associated with adopting the relatively new technology.
The Fed’s financial services division—the only business line at the agency that provides services to external stakeholders, including commercial banks—is looking at how AI can boost its customer engagement.
“We see huge potential for making the customer experience better through this technology and really having a tailored experience for the financial institutions,” said Riley. “Think about a community bank… their [needs] are definitely going to be different from what Wells Fargo needs, and you can think about how to achieve that customized experience based on the use of GenAI.”
Content generation is also in focus because the Fed produces large amounts of written materials for both internal and external use every year, often building on previous documents. GenAI can streamline content creation by leveraging existing materials. However, this application is most effective in scenarios where the new content is relatively predictable and consistent with the past.
“You can’t create content for topics that have a high degree of volatility” using GenAI, Tuteja noted.
Cracking the code
Commercial banks are already tending to prioritise AI-powered coding assistants, and the Fed experts speak highly of GenAI’s coding capability. Tuteja emphasized that the potential lies not only in generating new code, but more importantly, in using large language models (LLMs) to refine legacy code. This modernization capability is vital for the 110-year-old monetary authority, aiding the update and maintenance of several generations of software systems still in use internally.
“It’s hard to justify [hiring] coding developers to update all old code to new code, but now you can leverage LLMs and then the developer becomes the auditor or the editor versus the primary doer,” Tuteja said. This reduced workload when tackling legacy code will also free up their time for “higher order work”, she added.
When I think about the potential of GenAI, it is kind of [as] a super analyst
Margaret Riley, Federal Resrve
Another use case under active exploration involves leveraging the technology to enhance operational efficiency across what Tuteja described as the Fed’s “hyper complex multi-location, multi-dimensional networks.”
Riley added that the structure of the Fed is also a reason why having a centralized innovation office in the system to co-ordinate AI experiments is critical: “If you think about a diversified, decentralized system, you get the Federal Reserve Bank of San Francisco, New York, Chicago, [and others]. Everybody can be doing these experiments and not communicating with each other.”
Responsible innovation
Centralized experiments can also help ensure consistency in the regulation and supervision of the technology moving forward, Riley said. In this way, each office may leverage different data for their customized use cases, but they can all adhere to “the same idea” of risk management and experimental procedure. The Fed carefully scrutinizes banks’ use of AI as a supervisor, so it is essential that its assessment of the technology internally is equally rigorous.
“We should think about all the risks of doing something new, but we should also ask ourselves: what is the risk of not doing something?” said Tuteja. “Because sometimes the risk of inaction is greater than the risk of action, but the way to go forward has to be responsible.”
Tuteja said the Fed’s innovation journey started “from a place of curiosity” but they also approached it “with a healthy dose of humility”. The initial action the agency took when deciding to explore GenAI was to enhance education, ensuring that Fed staff have an adequate understanding of the technology.
Specifically, the agency hosts monthly regulator education sessions that are open to all staff members, inviting both internal leaders and external experts to join the discussions. In addition to exploring specific use cases, the innovation office also examines the technology from a broader ecosystem perspective. For example, it hosted a roundtable on the nexus of GenAI and the wider economy a few weeks ago, exploring how technology can affect economic indicators in the future.
The agency has also been through an enablement phase, in which it set up a “launch pad” that allows all staff members, regardless of their technological expertise, to access an internal GenAI system and experiment with it on their own.
“When you’re innovating, you can’t do it with assumptions. You enable people to put hands on keys and learn by doing,” said Tuteja. “It’s our colleagues that are closest to the end-product, the end-customer and end-platform, so the more they are exposed to the technology, the more use cases will be curated that can deliver value.”
The agency is now in an experimentation phase that involves exploring use cases and assessing risks before moving to the final stage of execution.
“Learning by doing experimentation is not just about all the upside… really, it’s also to understand where the downside is, and how we then mitigate and minimize the downside,” said Tuteja.
In terms of developing protocols and policies to address the risks, such as cyber security threats and AI bias, Tuteja argued that no-one needs to start with “a blank piece of paper”. Instead, she urged the industry to work together to identify areas where such risks are heightened and update the related policies accordingly.
Both regulators expressed optimism about the future of GenAI. Riley believes there may be “a tipping point” when the technology becomes the norm in the industry.
“I don’t think we’re there yet,” she said, but “I feel like we’re on our way there.”
Editing by Philip Alexander
Further reading
Only users who have a paid subscription or are part of a corporate subscription are able to print or copy content.
To access these options, along with all other subscription benefits, please contact info@waterstechnology.com or view our subscription options here: http://subscriptions.waterstechnology.com/subscribe
You are currently unable to print this content. Please contact info@waterstechnology.com to find out more.
You are currently unable to copy this content. Please contact info@waterstechnology.com to find out more.
Copyright Infopro Digital Limited. All rights reserved.
As outlined in our terms and conditions, https://www.infopro-digital.com/terms-and-conditions/subscriptions/ (point 2.4), printing is limited to a single copy.
If you would like to purchase additional rights please email info@waterstechnology.com
Copyright Infopro Digital Limited. All rights reserved.
You may share this content using our article tools. As outlined in our terms and conditions, https://www.infopro-digital.com/terms-and-conditions/subscriptions/ (clause 2.4), an Authorised User may only make one copy of the materials for their own personal use. You must also comply with the restrictions in clause 2.5.
If you would like to purchase additional rights please email info@waterstechnology.com
More on Emerging Technologies
This Week: Startup Skyfire launches payment network for AI agents; State Street; SteelEye and more
A summary of the latest financial technology news.
Waters Wavelength Podcast: Standard Chartered’s Brian O’Neill
Brian O’Neill from Standard Chartered joins the podcast to discuss cloud strategy, costs, and resiliency.
SS&C builds data mesh to unite acquired platforms
The vendor is using GenAI and APIs as part of the ongoing project.
Chevron’s absence leaves questions for elusive AI regulation in US
The US Supreme Court’s decision to overturn the Chevron deference presents unique considerations for potential AI rules.
Reading the bones: Citi, BNY, Morgan Stanley invest in AI, alt data, & private markets
Investment arms at large US banks are taken with emerging technologies such as generative AI, alternative and unstructured data, and private markets as they look to partner with, acquire, and invest in leading startups.
Startup helps buy-side firms retain ‘control’ over analytics
ExeQution Analytics provides a structured and flexible analytics framework based on the q programming language that can be integrated with kdb+ platforms.
The IMD Wrap: With Bloomberg’s headset app, you’ll never look at data the same way again
Max recently wrote about new developments being added to Bloomberg Pro for Vision. Today he gives a more personal perspective on the new technology.
LSEG unveils Workspace Teams, other products of Microsoft deal
The exchange revealed new developments in the ongoing Workspace/Teams collaboration as it works with Big Tech to improve trader workflows.