Firms worry that lack of ‘explainability’ will be regulatory roadblock for AI
Industry experts share their concerns about advanced AI’s ‘black-box’ nature and how that may attract fragmented regulatory scrutiny.
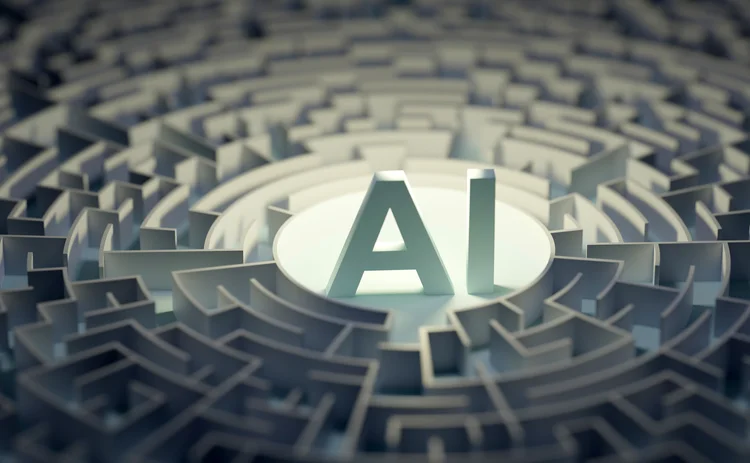
Those responsible for leading AI initiatives at financial firms fear that the opaque nature of generative AI models and the large language models (LLMs) on which they are trained may significantly delay its uptake for certain tasks as regulators seek greater clarity into its use and operation.
At last week’s North American Financial Information Summit, hosted by WatersTechnology, speakers on different panels repeatedly cited the challenge of explainability—i.e., the ability to explain how and why an AI model generates a particular result or arrives at a decision.
While only a few years ago firms were holding back on AI development, citing a lack of regulatory guidance, the current push to move quickly to take advantage of GenAI is resulting in a rushed and fragmented response from regulators, leading to fears that firms will be asked to comply with multiple and inconsistent rules from different jurisdictions, and that these rules will demand greater transparency into AI models and LLMs, which they may not be able to provide.
This amounts to knowing what technology platforms your firm is using, knowing how those platforms work, and being able to demonstrate that knowledge and understanding to customers—because customers also want to know how the sausage is made—and regulators, said Elena Treshcheva, program manger at Exactpro systems.
Tarun Sood, chief data officer at American Century Investments, said that while traditional AI and GenAI share certain key similarities, such as the importance of data quality and good data management, where GenAI differs is this explainability factor.
Other speakers also argued that GenAI can’t be treated in the same way as traditional AI: it’s smarter and more complex. But with that comes additional challenges, said Kristof Horompoly, former executive director and head of responsible AI at JPMorgan Chase.
“This is a shift in the industry mindset. Until now, we’ve had high expectations of AI and machine learning models to be explainable in some way … and to have insight into the underlying data points. But when you move into the GenAI space, you have to relax those expectations, and so for anything that’s regulated, you can’t use it for those tasks now.” He recently left the bank and earlier this month joined ValidMind, a Palo Alto, California-based provider of technology that performs automated documentation and validation of AI models for risk and regulatory purposes.
“There could be some use cases that are off limits to GenAI forever—which I find unlikely—or we figure out transparency and explainability,” said Horompoly, who serves as the company’s vice president of AI risk management. “Or we use the models’ performance over time to demonstrate they’re safe to use without fully understanding them.”
In theory, a firm could record and present the code used to build the model, the data sources used as inputs, the questions used to train it, and the results it generates, as well as how those results change over time as the model learns. But, warned Horompoly, “If you are using [an AI tool built by] a vendor, you have to accept that you’ll have no insight into what data was used to train a model.”
Weighing the trade-offs
Beyond potential regulatory interest, this lack of insight or explainability also poses challenges to firms managing these projects and trying to control and contain them in-house, requiring them to move more slowly than they would ideally like in the interests of reliability, accuracy and security—not to mention the risk of a model leaking confidential information, such as customer data, which would lead to reputational damage and also attract the attention of regulators.
“Traders want to build fast, because in financial services, speed is alpha,” Sood said. “We don’t want to halt innovation, but we have to safeguard our data.”
Vaibhav Bang, vice president of compliance advisory at Societe Generale, agreed, urging a cautious and controlled approach to rolling out AI models. “The saying, ‘Go fast and build things.’ shouldn’t be applied in this case. The expanded use of AI has to be done in a very slow and ethical fashion.”
Traders want to build fast, because in financial services, speed is alpha. We don’t want to halt innovation, but we have to safeguard our data
Tarun Sood, American Century Investments
But establishing guidelines and rules to keep financial firms on the straight and narrow and consistent with each other would require—at the very least—cooperation between regulators, if not the establishment of a global rulemaking body for the use of AI in finance. Rather than dealing with fragmented regulatory regimes, Horompoly advocated for a more global approach and standards, but said that any governing body to meaningfully address AI must do so without barriers between jurisdictions.
“Any governing body will need to be international, and will need to be powerful,” he said.
While much of the AI regulation enacted so far has focused on consumer privacy and protection, as well as IP protection, and combatting deepfakes, rather than anything specifically emerging from authorities that regulate capital markets, a large part of firms’ concerns in the meantime is who will be using AI within their organizations, and what level of understanding they have about it.
“We’re seeing a much broader audience using these [GenAI] implementations,” Horompoly said. “Traditional models were very quantitative and were used by subject matter experts to build quant models. But we’re seeing GenAI users across a whole company with different levels of understanding. So, what’s becoming more important is education. There might need to be training before people start using it; the risks and responsibilities need to be made clear; and people need to not take outputs for granted.”
Barriers to explainability
It’s the sometimes inconsistent and inaccurate results of AI—called hallucinations—that require people to still check results without relying blindly on them, or risk suffering monetary loss, reputational damage, or penalties if they use incorrect data generated by AI. These hallucinations are also a barrier to explainability, especially if the industry adopts the performance-monitoring option for demonstrating reliability.
How do we get rid of hallucinations? We probably don’t. Models always make errors. It’s about how you deal with hallucinations
Kristof Horompoly, ValidMind
“A lot of the ongoing monitoring will have to come from hard-coded guardrails or filters—either from a vendor or internally—and there needs to be a feedback channel for users to report issues,” Horompoly says. And while there are ethical questions surrounding the use of filters for protection—such as, who decides what type or level of filter to impose—the fact remains that introducing any filter will slightly degrade a model, so this approach will always involve a trade-off, he warns.
“How do we get rid of hallucinations? We probably don’t. Models always make errors. It’s about how you deal with hallucinations,” Horompoly says. One way could be to run models more than once, since it’s unlikely a model will make the same mistake twice. Another is to have human subject matter experts in the loop to spot hallucinations—though Horompoly warns that these approaches will result in significant increases to the cost of using AI.
He and other speakers all emphasized the importance of keeping people—specifically, staff with expert knowledge of the area being addressed—in the loop who have the experience to identify and monitor the AI’s successes and failures.
“The best risk management is an educated user,” said Iro Tasitsiomi, head of investments data science at T. Rowe Price.
Further reading
Only users who have a paid subscription or are part of a corporate subscription are able to print or copy content.
To access these options, along with all other subscription benefits, please contact info@waterstechnology.com or view our subscription options here: http://subscriptions.waterstechnology.com/subscribe
You are currently unable to print this content. Please contact info@waterstechnology.com to find out more.
You are currently unable to copy this content. Please contact info@waterstechnology.com to find out more.
Copyright Infopro Digital Limited. All rights reserved.
As outlined in our terms and conditions, https://www.infopro-digital.com/terms-and-conditions/subscriptions/ (point 2.4), printing is limited to a single copy.
If you would like to purchase additional rights please email info@waterstechnology.com
Copyright Infopro Digital Limited. All rights reserved.
You may share this content using our article tools. As outlined in our terms and conditions, https://www.infopro-digital.com/terms-and-conditions/subscriptions/ (clause 2.4), an Authorised User may only make one copy of the materials for their own personal use. You must also comply with the restrictions in clause 2.5.
If you would like to purchase additional rights please email info@waterstechnology.com
More on Regulation
Off-channel messaging (and regulators) still a massive headache for banks
Waters Wrap: Anthony wonders why US regulators are waging a war using fines, while European regulators have chosen a less draconian path.
Banks fret over vendor contracts as Dora deadline looms
Thousands of vendor contracts will need repapering to comply with EU’s new digital resilience rules
Chevron’s absence leaves questions for elusive AI regulation in US
The US Supreme Court’s decision to overturn the Chevron deference presents unique considerations for potential AI rules.
Aussie asset managers struggle to meet ‘bank-like’ collateral, margin obligations
New margin and collateral requirements imposed by UMR and its regulator, Apra, are forcing buy-side firms to find tools to help.
The costly sanctions risks hiding in your supply chain
In an age of geopolitical instability and rising fines, financial firms need to dig deep into the securities they invest in and the issuing company’s network of suppliers and associates.
Industry associations say ECB cloud guidelines clash with EU’s Dora
Responses from industry participants on the European Central Bank’s guidelines are expected in the coming weeks.
Regulators recommend Figi over Cusip, Isin for reporting in FDTA proposal
Another contentious battle in the world of identifiers pits the Figi against Cusip and the Isin, with regulators including the Fed, the SEC, and the CFTC so far backing the Figi.
US Supreme Court clips SEC’s wings with recent rulings
The Supreme Court made a host of decisions at the start of July that spell trouble for regulators—including the SEC.