Former Goldman analyst aims to blend GenAI and synthetic data with start-up
Synthera.ai is taking a novel approach to calculating risk. While promising, industry observers are skeptical.
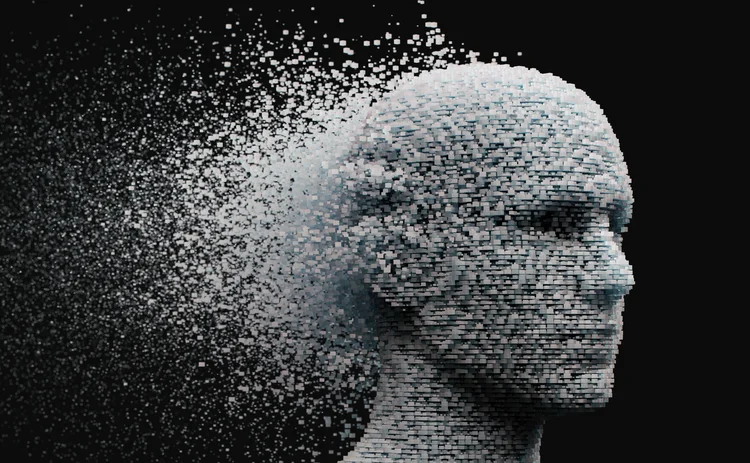
Monte Carlo simulations were born from the same minds that brought the world the nuclear bomb. Mathematicians Stanislaw Ulam and John Von Neumann, partners on the Manhattan Project, worked together to develop and refine these probabilistic models, which are commonly used for back-testing and “what-if” scenarios in risk management.
In finance, these simulations often run through scenarios thousands or millions of times, using random inputs each time, and then present the outcomes to a portfolio manager, quant, or risk manager for analysis. Monte Carlos are commonly used in pricing stock options and portfolio valuation, which is where Mariana Barona first encountered them.
Barona, CEO and co-founder of Synthera.ai, had been an analyst at Goldman Sachs Asset Management (GSAM) until September of last year, after she found that some risk models used in the industry were too reliant on specific inputs. She says there is only so much one can do with Monte Carlos, as their results tend to be less reliable during times of market stress and heightened volatility. However, she had assumed that this was just the nature of predictive risk solutions at the market’s disposal.
We have early results that show that we are 10 times more accurate at forecasting VaR than the normal distribution
Mariana Barona, Synthera.ai
“After you spend so much time in an organization, some things you stop acknowledging as problems, and you just accept them as truths,” Barona says. “It’s like, ‘Oh, yeah, well, everyone knows risk models aren’t great,’ or, ‘Everyone knows these predictive models are quite inflexible.’ There’s only so much you can do. And [Goldman Sachs Asset Management] is a world-leading institution. It has a state-of-the-art, massive tech team. There are very, very clever people working on it. So you’re probably not going to get much more sophisticated than that, right?”
But now, Barona is betting against her own previous assumption through Synthera.ai, which officially launched in January and seeks to bring an innovative approach to risk management through the synthesis of synthetic data and generative AI to provide “realistic” yield curves, stock prices, FX rates, and other metrics.
In the news
The hype around the new field of GenAI has led to the release of several new initiatives by many prominent financial services and data firms, due to both high expectations for the scope of generative AI, as well as keeping pace with competition from other companies.
Last month, FactSet released a new portfolio commentary tool leveraging AI solutions, and Chris Ellis, executive vice president and head of strategic initiatives at FactSet, told WatersTechnology that the company had a careful approach to using AI. “To use a Risk analogy—as in the board game—I don’t want to parachute into no man’s land, and then work my way back to what we do,” he said.
This cautious approach to AI implementation is mirrored on the buy side. The continual re-examination of workflow processes to see whether generative AI could augment them has led to a period of introspection for buy-side firms, which—outside of the most sophisticated quant shops—are typically reluctant to experiment with new technology until it has proven to be a consistent value-add.
Barona left GSAM after two years as an analyst and joined startup incubator Entrepreneur First, which paired her with former quant engineer Lukas Schreiner, Synthera.ai’s co-founder and CTO. The two developed models using synthetic data and deep learning.
Synthetic data is data generated artificially rather than produced by real-world events. Barona and Schreiner say that since historical data can be incomplete, it means Monte Carlo simulations are prone to errors when it comes to estimating tail risk, or the chance of a loss occurring due to a rare black swan event predicted by a probability distribution. In scenarios with limited data availability, such as forecasting yield curves in asset management, synthetic data performs comparably well to real-world data in model testing and can be generated much faster, Barona says.
“We are still around three months away from having a minimum viable product, but we have results that are performing really well, and the statistical similarity between the real and the synthetic data is very, very high—over 99%,” Barona says. “We have early results that show that we are 10 times more accurate at forecasting value at risk (VaR) than the normal distribution.”
Round peg, square hole?
The pair have lofty goals for their start-up. Barona says the goal is to be almost like a Bloomberg Terminal in terms of data provision, but in which the data is all synthetic as opposed to real-time and real-life. That way, Synthera.ai would operate as an engine that customers can test their portfolios on every day if needed.
The problem is risk numbers are backward-looking, and yet, risk is what we can’t see that lies ahead, so if someone tells me that they have used AI to nail risk, I’m deeply skeptical
Portfolio manager at a UK equity fund
Barona and Schreiner are also backed with technical knowledge from noted mathematician Rama Cont, statutory professor of mathematical finance at Oxford University and Synthera.ai’s scientific advisor. In this capacity, he helps to provide guidance on the more academic side of the company’s research.
While Synthera.ai’s proposition is exciting, it is not without its critics. The CEO of a risk technology vendor says that models that capture tail risk both implied by the options market (the risk-neutral measure) or implied historically by the real world (the physical measure) can already be constructed through Monte Carlo simulations. And they believe that it is unlikely AI would be able to do a better or more detailed job of analyzing these models.
“An AI might be able to do a reasonable job of inferring the physical measure from historical data, but it is questionable, given the data available, whether it will do any better than conventional inference techniques,” they say.
Barona explains that the machine-learning model Synthera.ai uses internally learns how data is distributed instead of making an assumption about data and putting a standard formula onto it, thus capturing more information.
“You can train it so it learns the correlations between one asset and macro factors,” she says. “For example, let’s say you’re an investor, and you have a portfolio of 30 stocks. Then if you put it through our model, it would calculate the correlation between each one of those stock prices—let’s say the Apple stock price over time—with a whole bunch of macro factors. It dynamically adapts over time. And it also understands the correlation between Apple’s stock price and the other assets in your portfolio.”
The CEO of the risk tech vendor says that in their experience, the increase of information would be unlikely to make an actionable difference in whether the models are better analyzed by a human or an AI.
“It seems a stretch to believe that there is enough information in the market for an AI to do a better job than a human of inferring one model vs. another,” they say. “It would be remarkable if an AI were able to do this consistently over time given the relative paucity of the available datasets, and even more remarkable for that AI to come up with consistent results with different sample windows and data selections.”
A portfolio manager at a UK equity fund shares this doubt, adding that the nature of risk management is a problem with no perfect solution, as risk models are always blown up by unforeseen events, no matter how much future-proofing went into them.
“The problem is risk numbers are backward-looking, and yet, risk is what we can’t see that lies ahead, so if someone tells me that they have used AI to nail risk, I’m deeply skeptical,” they say.
Filling in a blank
Barona and Schreiner explain that since the technology used by Synthera.ai is so nascent and the financial investment to build in-house is so substantial, they don’t believe there is much risk of their business offering being replaced by their would-be clients building synthetic data solutions in-house.
“Other banks and hedge fund managers are looking into this, but it’s a very new field of research, and many of the breakthroughs on the scientific side that make this actually useful and not just a nice science experiment have been made in the last one or two years,” Schreiner says. “All the big firms are deploying a few PhD students to look into it, but to our knowledge, nobody has built something in production yet.”
This affords Synthera.ai some breathing room to work on its product without looking over its shoulder while the team seeks to raise capital in San Francisco. Capital is also one of the reasons Barona believes they have managed to carve out a niche that a large bank or hedge fund could not fill.
“From a unit economics perspective, it does not make sense for most of these people to build in-house,” she says. “We’ve heard of one or two people experimenting with it in-house, but once they build a sensor for a specific use-case, they use it and that’s it—while what we’re selling is really an infrastructure tool. We want to be integrated into the workflow of an investor, and we want them to use our data to inform their investment decisions.”
Barona says that for a lot of bigger banks and investment management funds, it would likely cost between $1 and $2 million per year to build and maintain a synthetic data solution similar to Synthera.ai’s offering in-house. Once the startup is taking clients, the current plan is that the product will cost roughly $50,000 per team, per year.
Whether Synthera.ai’s solution is remarkable or not in practice remains to be seen, as the company is still getting off the ground. But Barona is confident in the company’s value proposition.
“We started this in November, so it’s very, very new, and at the moment it is just us,” Barona says. “That said, we do have early signs it is working well.”
Only users who have a paid subscription or are part of a corporate subscription are able to print or copy content.
To access these options, along with all other subscription benefits, please contact info@waterstechnology.com or view our subscription options here: http://subscriptions.waterstechnology.com/subscribe
You are currently unable to print this content. Please contact info@waterstechnology.com to find out more.
You are currently unable to copy this content. Please contact info@waterstechnology.com to find out more.
Copyright Infopro Digital Limited. All rights reserved.
As outlined in our terms and conditions, https://www.infopro-digital.com/terms-and-conditions/subscriptions/ (point 2.4), printing is limited to a single copy.
If you would like to purchase additional rights please email info@waterstechnology.com
Copyright Infopro Digital Limited. All rights reserved.
You may share this content using our article tools. As outlined in our terms and conditions, https://www.infopro-digital.com/terms-and-conditions/subscriptions/ (clause 2.4), an Authorised User may only make one copy of the materials for their own personal use. You must also comply with the restrictions in clause 2.5.
If you would like to purchase additional rights please email info@waterstechnology.com
More on Emerging Technologies
This Week: Startup Skyfire launches payment network for AI agents; State Street; SteelEye and more
A summary of the latest financial technology news.
Waters Wavelength Podcast: Standard Chartered’s Brian O’Neill
Brian O’Neill from Standard Chartered joins the podcast to discuss cloud strategy, costs, and resiliency.
SS&C builds data mesh to unite acquired platforms
The vendor is using GenAI and APIs as part of the ongoing project.
Chevron’s absence leaves questions for elusive AI regulation in US
The US Supreme Court’s decision to overturn the Chevron deference presents unique considerations for potential AI rules.
Reading the bones: Citi, BNY, Morgan Stanley invest in AI, alt data, & private markets
Investment arms at large US banks are taken with emerging technologies such as generative AI, alternative and unstructured data, and private markets as they look to partner with, acquire, and invest in leading startups.
Startup helps buy-side firms retain ‘control’ over analytics
ExeQution Analytics provides a structured and flexible analytics framework based on the q programming language that can be integrated with kdb+ platforms.
The IMD Wrap: With Bloomberg’s headset app, you’ll never look at data the same way again
Max recently wrote about new developments being added to Bloomberg Pro for Vision. Today he gives a more personal perspective on the new technology.
LSEG unveils Workspace Teams, other products of Microsoft deal
The exchange revealed new developments in the ongoing Workspace/Teams collaboration as it works with Big Tech to improve trader workflows.