Vanguard cautiously explores neural networks for alt data analysis
John Ameriks, head of Vanguard’s Quantitative Equity Group, explains the rationale behind dataset selection and how the group has been using machine learning.
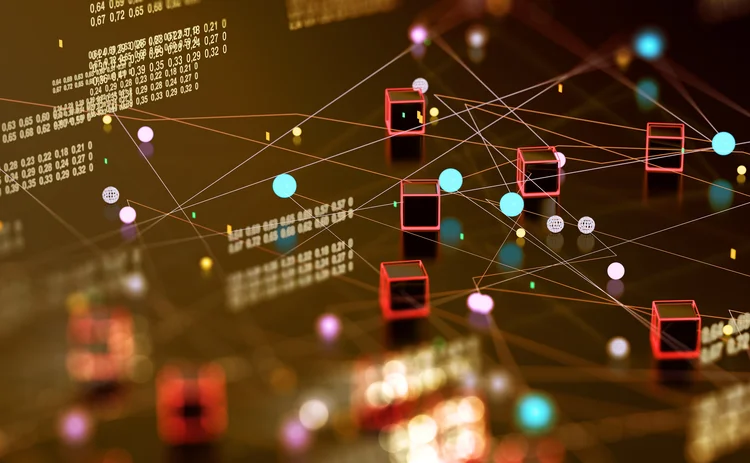
John Ameriks has seen significant movement and change in the data landscape since joining the asset manager in 2003, and he’s done his best to ensure Vanguard’s Quantitative Equity Group has moved and changed with it.
QEG has 30 members, including portfolio managers, analysts, and QEG’s quantitative development and innovation team, which is in charge of sitting in between the portfolio managers and analysts and integrating new technologies into the trading process. QEG’s alpha side looks at analyst reports in order to determine which characteristics make datasets attractive, as well as which data vendors to buy the attractive datasets from.
“We look at tech firms within the tech space, we look at pharma firms within a pharma category, and we’re really trying to figure out what firms have a set of attractive characteristics that we think will outperform over the long term,” says Ameriks, global head of QEG.
Alternative data, which refers to non-traditional sources of information beyond market data and reference data, has been prized by buy-side firms for years due to the unique benefits it can yield compared to traditional datasets. When hunting for new datasets, asset managers like Vanguard want options that give them an edge over their competition, but that also have enough history to be usable over a longer period.
“We stay away from—and probably don’t have a lot of utility for—things that are very, very short-horizon,” Ameriks says. “We don’t pay attention to information that has a very, very short half-life in and of itself; we want to try to get to things that are going to help us suss out fundamental differences in the attractiveness of firms over longer periods of time.”
Ghost in the machine
One of QEG’s largest innovations in its alpha generation process was the introduction more than a year ago of a neural network-driven process into its alpha generation engine. Ameriks says this works as an overlay across the traditional investing process and aims to increase the portfolio managers’ efficiency. However, the decision to use AI at Vanguard was met with skepticism internally, not least from Ameriks himself. He describes the process of incorporating AI within QEG as “restrained.”
“I have just as much skepticism about machine learning and AI as anyone in the industry has,” he says. “I mean, these things were designed to fit data [inside them]. It’s very easy to go back [and analyze] a historical dataset and there’s no uncertainty in a historical dataset. All the uncertainty is in going forward. And it is just a matter of an algorithm trying to fit a historical curve. The big question is, how well is that going to fit the world that arises in, where uncertainty is important going forward?”
Ameriks says that within QEG, there was equal focus on working out how to continuously re-examine and intervene in the AI’s work and on observing the kind of work it would do if it were free of human interaction. Attempting to interpret the AI’s role in QEG’s performance was difficult, so the team created an internal “interpretability engine,” which helps QEG parse what the signals emitted by the neural network mean.
Ameriks is aware that the use of AI in quant shops is controversial. Analyzing risks is difficult when an AI tries to fit extraneous data points onto a curve.
“The machine is able to do so much more work computationally than what we would traditionally try to do with that kind of analysis, that you end up with way too many degrees of freedom,” he says. “You end up with something that is just either data mining or curve fitting—whatever you want to call it—and I think that’s always been a danger for quants.”
Ameriks says that for now, human oversight of AI tools is key.
“People have asked before, ‘Do you worry about AI and ML as these machines get smarter? Will there continue to be a role for humans?’ I guess that’s the most frustrating thing,” he says. “I mean, do we have enough time in the day for the humans? I think we do, to look at this need to really assess the validity of what’s coming out of the machines.”
The trouble with alt data
Daryl Smith, head of research at London-based alternative data consultancy Neudata, says many companies find it difficult to parse datasets that have a unique premise but insufficient history, as the data itself may be too niche for companies like Vanguard to buy without those additional features.
“There’s probably 100 examples I can give you of companies that do weird and wacky things, but the common theme across them is that they are often very niche in terms of applicability,” Smith says. “That’s actually where the likes of Vanguard are probably going to struggle, because they want to find datasets on the edge, so to speak, that very few other funds are using, but they also want to have their cake and eat it in the sense that they want a large universe of applicability.”
Classic alternative data archetypes such as web-scraped data and social media feeds that don’t immediately possess sufficient information are regarded warily by John Ameriks, global head of Vanguard’s Quantitative Equity Group. Reliability of the data is key, and QEG is not in the business of taking risks on unknown datasets.
“Like a lot of other firms, we did spend a lot of time—I would say five or seven years ago—looking at various sources of so-called sentiment data,” Ameriks says. “We spent a lot of time trying to find useful information in that kind of data. And the issue is it’s not always related to fundamentals. If the source of information really doesn’t have some sort of responsibility for it being correct and being accurate—meaning there’s no penalty for it containing a lot of noise—wherever it comes from, the likeliness that it is going to be of use to us is very, very low.”
One of the endemic issues in the alternative data space arises from this problem of picking datasets that are unique, not chosen by a competitor, and have potential long-term value. Competitors learning of valuable datasets already being used by one asset manager vastly reduces the usefulness of that dataset to contribute to the asset manager beating the market—what is known as alpha decay. Neudata’s Smith explains that large firms in the alternative data market are attempting to combat alpha decay by not only buying trending datasets, but also buying smaller datasets to get ahead of the first dataset.
“It’s an evolutionary thing—it’s a survival of the fittest thing—and it’s a constantly moving game,” Smith says.
Josef Schmalfuss, CEO of alternative data vendor Oxford Data Plan, disagrees that without sufficient history, unique datasets are of very low use to potential buyers. Schmalfuss spent seven years as a partner at London-based equity long/short fund Portsea Asset Management, and based on his experience in charge of technology investments at Portsea, he was invited to become a guest lecturer at the University of Oxford. After leaving Portsea in 2022, Schmalfuss launched Oxford Data Plan.
He says that when the alternative data space was new, creating alpha through investment on the buy side was possible, but few people were doing it, and even fewer were doing it well. He likens today’s alt data to Bloomberg market data—a must-have to even be part of the conversation, but having it doesn’t guarantee that it will make money.
“For quants to create good models they need history; they need point-of-time data to understand how they would have performed in the past if they had had the data at that point,” Schmalfuss explains. “The reality is if you have a really cool and unique dataset and you only have two years of history, people will still look at it—there’s still money to be made. If the trade offer is going to be between uniqueness and history, and you can get a unique dataset, it’ll get taken any day over a dataset that is similar to what is out there.”
Further reading
Only users who have a paid subscription or are part of a corporate subscription are able to print or copy content.
To access these options, along with all other subscription benefits, please contact info@waterstechnology.com or view our subscription options here: http://subscriptions.waterstechnology.com/subscribe
You are currently unable to print this content. Please contact info@waterstechnology.com to find out more.
You are currently unable to copy this content. Please contact info@waterstechnology.com to find out more.
Copyright Infopro Digital Limited. All rights reserved.
As outlined in our terms and conditions, https://www.infopro-digital.com/terms-and-conditions/subscriptions/ (point 2.4), printing is limited to a single copy.
If you would like to purchase additional rights please email info@waterstechnology.com
Copyright Infopro Digital Limited. All rights reserved.
You may share this content using our article tools. As outlined in our terms and conditions, https://www.infopro-digital.com/terms-and-conditions/subscriptions/ (clause 2.4), an Authorised User may only make one copy of the materials for their own personal use. You must also comply with the restrictions in clause 2.5.
If you would like to purchase additional rights please email info@waterstechnology.com
More on Data Management
New working group to create open framework for managing rising market data costs
Substantive Research is putting together a working group of market data-consuming firms with the aim of crafting quantitative metrics for market data cost avoidance.
Off-channel messaging (and regulators) still a massive headache for banks
Waters Wrap: Anthony wonders why US regulators are waging a war using fines, while European regulators have chosen a less draconian path.
Back to basics: Data management woes continue for the buy side
Data management platform Fencore helps investment managers resolve symptoms of not having a central data layer.
‘Feature, not a bug’: Bloomberg makes the case for Figi
Bloomberg created the Figi identifier, but ceded all its rights to the Object Management Group 10 years ago. Here, Bloomberg’s Richard Robinson and Steve Meizanis write to dispel what they believe to be misconceptions about Figi and the FDTA.
SS&C builds data mesh to unite acquired platforms
The vendor is using GenAI and APIs as part of the ongoing project.
Aussie asset managers struggle to meet ‘bank-like’ collateral, margin obligations
New margin and collateral requirements imposed by UMR and its regulator, Apra, are forcing buy-side firms to find tools to help.
Where have all the exchange platform providers gone?
The IMD Wrap: Running an exchange is a profitable business. The margins on market data sales alone can be staggering. And since every exchange needs a reliable and efficient exchange technology stack, Max asks why more vendors aren’t diving into this space.
Reading the bones: Citi, BNY, Morgan Stanley invest in AI, alt data, & private markets
Investment arms at large US banks are taken with emerging technologies such as generative AI, alternative and unstructured data, and private markets as they look to partner with, acquire, and invest in leading startups.