GenAI offers promise, complexity for research management
Research management system providers are building on their AI capabilities using LLMs to improve the research process, but challenges remain.
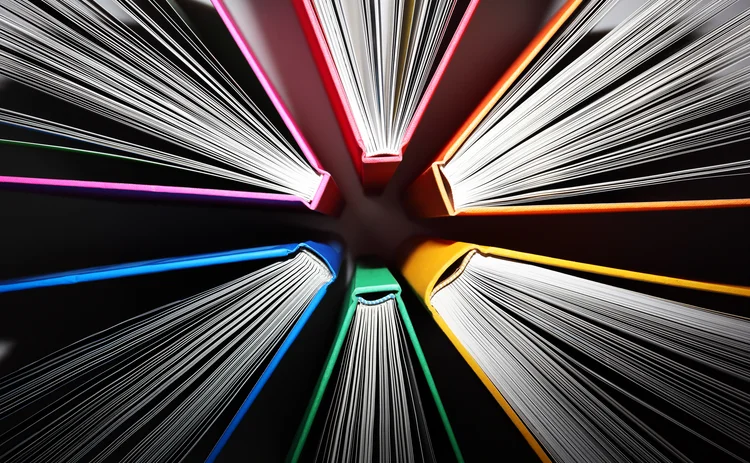
In 2018, Google unleashed BERT onto the world. The Bidirectional Encoder Representations from Transformers language model was a game changer in the world of artificial intelligence research. It marked a turning point in AI being able to understand natural language at levels comparable to humans. But BERT ultimately amounted to a mere stepping stone in the evolution of modern AI systems.
The “T” in BERT stands for transformers, a deep-learning architecture first introduced in a 2017 Google study titled, “Attention Is All You Need.” Google’s researchers were initially looking to improve the technology behind Google Translate, but that study is now widely considered to be what ushered in the modern AI movement as transformers are the main architecture underpinning large language models (LLMs).
On the demand side, think about how you could summarize and quickly go over data, especially if you have your data organized
Brad Bailey, Burton-Taylor International Consulting
As the story goes, that study also got the attention of a San Francisco start-up that had been reading Google research papers to keep up. At the end of 2022, that start-up released ChatGPT, setting off a frenzy of interest and investment into AI.
As capital markets firms examine where generative AI and LLMs can best be applied, one area has already emerged as an early contender: research.
The perfect fit
Brad Bailey, research director at Burton-Taylor International Consulting, says generative AI fits “perfectly” into the supply and demand side of research management. “On the demand side, think about how you could summarize and quickly go over data, especially if you have your data organized,” he says, adding that the ability to ask more advanced questions and tie different names to different data and supply chains has massive potential.
“On the other side of the coin is the supply side and people that produce research,” he says. How the research is being consumed, who is using it, the depth and extent of the organizations using it, and how they are sharing it are all questions that could be answered for providers.
Verity, a research management system provider created from the 2021 merger of MackeyRMS and text data specialist InsiderScore, has spent the last year leveraging LLMs so that users don’t have to be as prescriptive about the content they want to see. Instead, the system can study the corpus of their firm to propose relevant content. Verity CEO Andrew Robson sees this as a move from the third-generation RMS to the fourth.
“Based on a lot of new developments in technology like generative AI, we’re starting to have more and more tools at our disposal to allow the RMS to be more proactive in highlighting insights and enabling better discovery,” he says.
Robson told WatersTechnology in 2021 that the third-generation RMS was “moving from a system where clients meet to share and act on information to one that begins to alert the investment team to trends, insights, and correlations in their internal and external data that are relevant, timely, and actionable.”
Verity’s first discussions around generative AI came during the company’s first board meeting of 2023. Robson says one board member mentioned he was using ChatGPT for his start-up, and the commercial opportunities interested the board. “We immediately pulled together a team of about half a dozen, kind of a skunkworks team across product, engineering, and research,” he says. They then began examining possible use cases and started to plot out what they might build.
They started with summarizing documents—the “low-hanging fruit,” Robson says—and quickly realized how fast they could get up and running with something in beta to show customers before putting it into production. This was followed last summer by generating and tagging. Verity had existing technology for tagging mechanisms but found that generative AI further augmented it.
For example, if a user published a note but didn’t include the ticker of the referenced company—say, Nike—the system could create metadata that establishes its connection to the NKE ticker.
Building on the foundation
The combination of AI and research isn’t new to the capital markets. Long before “large language model” entered Wall Street’s lexicon, deep learning and natural language processing captured similar levels of attention.
Data and research provider FactSet is looking to build on its history in AI with enhancements to its research products. “We’ve had these capabilities in different formats for years,” says Danielle Karr, senior vice president of research management systems at FactSet. “So we’re well positioned to jump from there.”
The BERT-based language models were really good at classification tasks, like sentiment analysis, but they were not good at text generation tasks. That was the big use-case breakthrough that came with getting into models that were in the billions or tens of billions of parameters
Chris Ackerson, AlphaSense
In December, the vendor launched FactSet Mercury, an LLM-based knowledge agent, into its FactSet Explorer product preview program. The beta offering allows users to access FactSet data as well as regulatory information through a chat interface. “It will be the mainstay of the FactSet user experience because it will be able to provide you the opportunity to ask contextual questions wherever you are in the workstation,” Karr says. As an example, the interface could be used to prepare for earnings call season by pulling up year-by-year commentary on companies.
Mercury is just one piece of FactSet’s generative AI roadmap. In November, Kristina Karnovsky, executive vice president and chief product officer at FactSet, laid out to WatersTechnology the company’s three-pillar approach to AI, which includes mile-wide discoverability, mile-deep workflow automation, and mile-high innovation acceleration.
In a similar vein, research analytics provider AlphaSense has considered itself AI-driven from day one. Chris Ackerson, vice president of product at AlphaSense, says the company started incorporating deep learning in 2015 and 2016.
“The really big breakthrough with language models was BERT, and we migrated many of our deep-learning models to these BERT-based architectures—these language models that were at that time very large but much smaller than the current generation,” he says. “So, with what occurred in late 2022 to early 2023, the models got much larger, and that was sort of an evolutionary change for us and how we did our AI development.”
When Google published BERT, it supplied the code and downloadable versions of the model already pre-trained on Wikipedia and a dataset called the BookCorpus—about 3 million words in total. Anyone could download and run the model without having to duplicate the costly and energy-intensive process of training it, so companies that offered NLP products and services were able to update their offerings to transformer models for increased efficiency and speed.
Ackerson, who previously worked on IBM Watson in engineering and product roles, says the evolution in AI has allowed newer models to be capable of more than their predecessors. “The BERT-based language models were really good at classification tasks, like sentiment analysis, but they were not good at text generation tasks,” he says. “That was the big use-case breakthrough that came with getting into models that were in the billions or tens of billions of parameters.”
In 2022, AlphaSense acquired competitor Sentieo, which offered a cloud-based research management system for investment analysts and researchers. The year prior, AlphaSense bought Stream, a provider of expert interview transcripts. AlphaSense's offering now comprises over 1,000 sell-side research providers, market news, trade journals and regulatory information, leveraging AI to organize all the content and millions of documents flowing through its system and provide search on top of it.
As some firms have looked to third parties for their AI technology, AlphaSense is crafting an internal strategy. It has developed its own LLM, AlphaSense Large Language Model. “It’s verticalized, so it’s trained in the domain, and it understands the complexity and nuance of financial language, capital markets, and business context,” Ackerson says. “We’ve fine-tuned it on the specific types of questions, tasks, and workflows that users want to execute in AlphaSense.”
He says that even with a smaller model, it is able to deliver higher accuracy and higher performance than some of the largest models that are on the market. And it means AlphaSense does not send client data to any third-party systems.
“What we saw at the end of 2022 into 2023 is that these generative models have finally became good enough that they could take that next step of not just identifying a trend, or helping understand important metadata associated with a document,” he says. “We could go a step further and actually generate summaries on top of that information, so human-readable language could be created.”
Through the AlphaSense platform, a user can ask a question and the system can help them find the right documents to answer it. With generative AI, Ackerson says, the system can go a step further and summarize that information without a user having to go into individual documents to find the relevant pieces of information.
In applying any new or experimental technology, there are always risks, and a significant one posed by generative AI systems is hallucination. “For us in a professional context, we had to take those challenges head-on, [and] we had to solve those challenges before we could release these generative AI features,” Ackerson says. “Because our users rely on us to meet a certain threshold of reliability accuracy in a professional context.”
To tackle this, AlphaSense looked at earnings call summarization first. Every summary bullet is auditable to the exact statement in the source data. Ackerson says accuracy rose to more than 99.9%, reducing the risk of hallucination to near zero.
“Once we solved those problems, we went after more and more ambitious use cases,” he says.
Further reading
Only users who have a paid subscription or are part of a corporate subscription are able to print or copy content.
To access these options, along with all other subscription benefits, please contact info@waterstechnology.com or view our subscription options here: http://subscriptions.waterstechnology.com/subscribe
You are currently unable to print this content. Please contact info@waterstechnology.com to find out more.
You are currently unable to copy this content. Please contact info@waterstechnology.com to find out more.
Copyright Infopro Digital Limited. All rights reserved.
As outlined in our terms and conditions, https://www.infopro-digital.com/terms-and-conditions/subscriptions/ (point 2.4), printing is limited to a single copy.
If you would like to purchase additional rights please email info@waterstechnology.com
Copyright Infopro Digital Limited. All rights reserved.
You may share this content using our article tools. As outlined in our terms and conditions, https://www.infopro-digital.com/terms-and-conditions/subscriptions/ (clause 2.4), an Authorised User may only make one copy of the materials for their own personal use. You must also comply with the restrictions in clause 2.5.
If you would like to purchase additional rights please email info@waterstechnology.com
More on Emerging Technologies
This Week: Startup Skyfire launches payment network for AI agents; State Street; SteelEye and more
A summary of the latest financial technology news.
Waters Wavelength Podcast: Standard Chartered’s Brian O’Neill
Brian O’Neill from Standard Chartered joins the podcast to discuss cloud strategy, costs, and resiliency.
SS&C builds data mesh to unite acquired platforms
The vendor is using GenAI and APIs as part of the ongoing project.
Chevron’s absence leaves questions for elusive AI regulation in US
The US Supreme Court’s decision to overturn the Chevron deference presents unique considerations for potential AI rules.
Reading the bones: Citi, BNY, Morgan Stanley invest in AI, alt data, & private markets
Investment arms at large US banks are taken with emerging technologies such as generative AI, alternative and unstructured data, and private markets as they look to partner with, acquire, and invest in leading startups.
Startup helps buy-side firms retain ‘control’ over analytics
ExeQution Analytics provides a structured and flexible analytics framework based on the q programming language that can be integrated with kdb+ platforms.
The IMD Wrap: With Bloomberg’s headset app, you’ll never look at data the same way again
Max recently wrote about new developments being added to Bloomberg Pro for Vision. Today he gives a more personal perspective on the new technology.
LSEG unveils Workspace Teams, other products of Microsoft deal
The exchange revealed new developments in the ongoing Workspace/Teams collaboration as it works with Big Tech to improve trader workflows.