Snowflake: Data strategy essential for AI
The cloud data platform provider continues to make investments in data quality and cleansing.
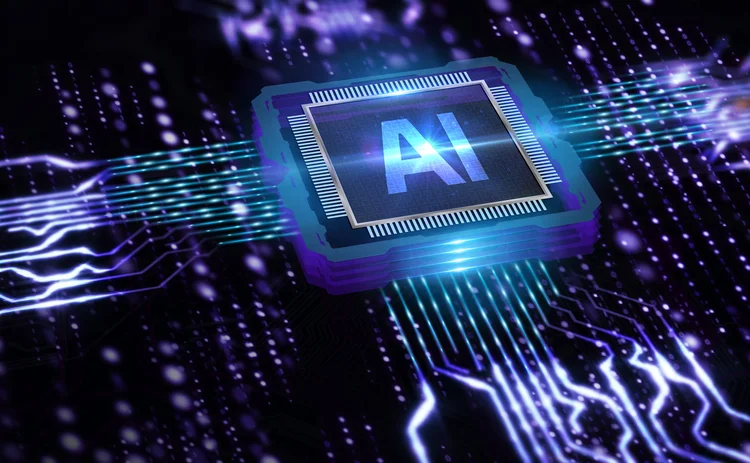
During this latest earnings call season, many banks, exchanges, and financial services providers have touted their large language model (LLM) capabilities and their focus on generative AI. But having an AI strategy—and that includes a generative AI strategy—cannot work without a data strategy.
While there is huge potential for institutions to leverage generative AI and LLMs, setting the data foundations to enable firms to execute their AI strategies is more important, Frank Slootman, chairman and CEO at Snowflake, said during the company’s Q2 earnings call on August 23.
“Generative AI is at the forefront of customer conversations. However, enterprises are also realizing that they cannot have an AI strategy without a data strategy to base it on. We have a head start in this race, as the epicenter of highly curated, optimized and trusted enterprise data,” he said.
Particularly when it comes to deploying LLMs, firms need to have highly organized, optimized, trusted, and sanctioned data.
“If you think you can just drop a model on top of a data lake and just see what happens, that’s not going to end well and that’s what people are realizing. … If you don’t have a good foundation, there’s not much you can build on top of that,” he said.
There are also governance and regulatory issues that firms must contend with. Slootman said determining who can access what data also needs to be translated into the world of LLMs too.
This is where being “extremely organized in your data” will have a premium.
Christian Kleinerman, SVP of product at Snowflake, added that the result of traditional machine learning or generative AI must be a function of having the right data, quality, and metrics.
“The technology will be as good as the data that is fed in, so all the investments we make on data quality and cleansing and pipelines—all of that is very important,” he said.
On top of that, it is equally important to measure and get feedback to determine how good the solutions are and if there are potential biases in the data or gaps in understanding the model’s performance.
During the quarter, Snowflake’s product revenue grew 37% year-on-year to $640 million. Customers that are data sharing also grew 20% against the same period a year ago.
Meanwhile, Snowflake’s Snowpark, which allows developers to write code in their preferred language and run it directly on Snowflake, had more than 400 customers. Slootman said 63% of Snowflake’s global 2,000 customers are using Snowpark on a weekly basis.
Snowpark exposes new interfaces for development in Python, Scala, or Java to supplement Snowflake’s original SQL interface.
During the Snowflake Summit 2023 in June, Snowflake launched Document AI and Snowpark Container Services, both of which are available for private preview.
Document AI uses a “leading LLM” to help customers extract information from documents. “With Document AI, customers can use natural language to ask questions of unstructured data. Legal contracts or invoices are now available for inquiry and analytics. This is an early example of how language models are expanding our opportunity,” Slootman said.
Meanwhile, Snowpark Container Services helps firms bring full-stack applications, LLMs, and other data products to the data securely.
Further reading
Only users who have a paid subscription or are part of a corporate subscription are able to print or copy content.
To access these options, along with all other subscription benefits, please contact info@waterstechnology.com or view our subscription options here: http://subscriptions.waterstechnology.com/subscribe
You are currently unable to print this content. Please contact info@waterstechnology.com to find out more.
You are currently unable to copy this content. Please contact info@waterstechnology.com to find out more.
Copyright Infopro Digital Limited. All rights reserved.
As outlined in our terms and conditions, https://www.infopro-digital.com/terms-and-conditions/subscriptions/ (point 2.4), printing is limited to a single copy.
If you would like to purchase additional rights please email info@waterstechnology.com
Copyright Infopro Digital Limited. All rights reserved.
You may share this content using our article tools. As outlined in our terms and conditions, https://www.infopro-digital.com/terms-and-conditions/subscriptions/ (clause 2.4), an Authorised User may only make one copy of the materials for their own personal use. You must also comply with the restrictions in clause 2.5.
If you would like to purchase additional rights please email info@waterstechnology.com
More on Data Management
New working group to create open framework for managing rising market data costs
Substantive Research is putting together a working group of market data-consuming firms with the aim of crafting quantitative metrics for market data cost avoidance.
Off-channel messaging (and regulators) still a massive headache for banks
Waters Wrap: Anthony wonders why US regulators are waging a war using fines, while European regulators have chosen a less draconian path.
Back to basics: Data management woes continue for the buy side
Data management platform Fencore helps investment managers resolve symptoms of not having a central data layer.
‘Feature, not a bug’: Bloomberg makes the case for Figi
Bloomberg created the Figi identifier, but ceded all its rights to the Object Management Group 10 years ago. Here, Bloomberg’s Richard Robinson and Steve Meizanis write to dispel what they believe to be misconceptions about Figi and the FDTA.
SS&C builds data mesh to unite acquired platforms
The vendor is using GenAI and APIs as part of the ongoing project.
Aussie asset managers struggle to meet ‘bank-like’ collateral, margin obligations
New margin and collateral requirements imposed by UMR and its regulator, Apra, are forcing buy-side firms to find tools to help.
Where have all the exchange platform providers gone?
The IMD Wrap: Running an exchange is a profitable business. The margins on market data sales alone can be staggering. And since every exchange needs a reliable and efficient exchange technology stack, Max asks why more vendors aren’t diving into this space.
Reading the bones: Citi, BNY, Morgan Stanley invest in AI, alt data, & private markets
Investment arms at large US banks are taken with emerging technologies such as generative AI, alternative and unstructured data, and private markets as they look to partner with, acquire, and invest in leading startups.