The causal AI wave could be the next to hit
As LLMs and generative AI grab headlines, another AI subset is gaining ground—and it might solve what generative AI can’t.
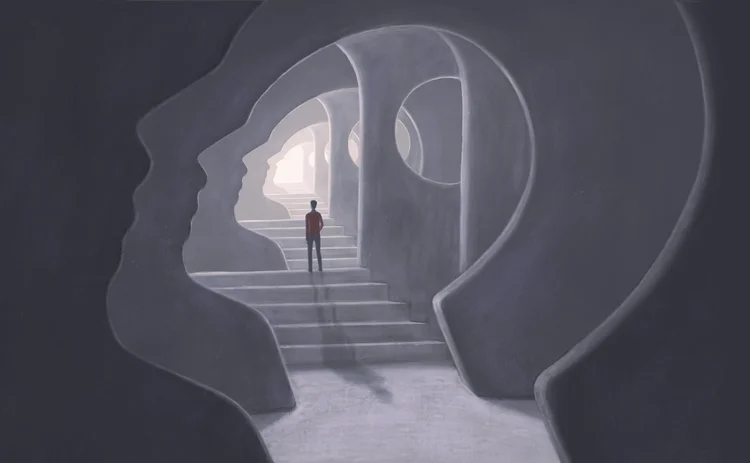
This has been a monumental year for the advancement of artificial intelligence in the capital markets. Since the rollout of OpenAI’s ChatGPT in November 2022, all eyes have been on generative AI and the large language models (LLMs) that underpin them. Across virtually all industries, conversations are taking place about the best ways to utilize the technology and regulate its use.
The 2023 Gartner Hype Cycle for Emerging Technologies put generative AI at the peak of inflated expectations for this year and projected the technology could reach transformational benefit within two to five years. But generative AI wasn’t the only area of AI included in the yearly report.
Causal AI made the list as well, sitting at the lower middle area of the chart in the innovation trigger area of the chart, with a projected two to five years until it plateaus. In fact, Gartner has included it in its past four hype cycles.
This area of AI isn’t new and practitioners tell WatersTechnology that its presence on the hype cycles means it’s getting more attention. Some also argue that in the capital markets, causal AI could offer a solution where there are gaps in generative AI systems.
What is causal AI?
Simply put, causal AI looks at the causation, causality or cause-and-effect relationships of data, which can then impact the outputs of AI models.
“The main issue with existing machine learning and AI is that it’s based on correlations and it learns from past patterns in data,” says Alfonso Parra Garcia, associate director in the office of the CEO at CausaLens, a startup that specializes in causal AI techniques for investing. LLMs, for example, look for patterns based on the patterns in their training data.
“That has some issues because many things are correlated but might not necessarily be causal. They can be correlated just by chance or because there’s something that’s causing two things at the same time.”
The example Garcia gives is the correlation between shark attacks and ice cream sales. “If you throw this data into a machine learning algorithm, it will learn that you can predict the number of shark attacks using ice cream sales, or vice versa, just because those two factors are very highly correlated.”
Humans can easily recognize there is no direct relationship between these two things even though they spike at the same time because they tend to happen in the summer months. In this case, temperature is driving both those things. In the study of causality, this is referred to as a confounding relationship.
Garcia says establishing the cause-and-effect relationships of data points can have an impact on the models that are then built. Causal AI models can be more trustworthy and explainable because they can demonstrate how the model makes decisions. In the example of shark attacks and ice cream sales, a causal graph can explain that temperature is behind the correlation.
While traditional machine learning models can forecast, causal AI is able to simulate the effects of interventions or what-if scenarios. If the temperature increases by x degrees, how does that impact ice cream sales and how does it impact shark attacks?
By establishing a causal structure, it’s understood that changing temperature will impact ice cream sales and shark attacks, but changing the number of ice cream sales will have no bearing on the number of shark attacks.
A growing field
CausaLens was founded in 2017 by Darko Matovski and Maksim Sipos, who came from the hedge fund industry. The story goes that Matovski and Sipos were eating at a Turkish restaurant and discussing how correlation doesn’t imply causation. It was something they felt hadn’t been properly solved but given their finance backgrounds, there was potential to build something. Today, the startup’s platform, decisionOS, allows users to deploy causal models that can then be used by non-technical users. In January of last year, the startup raised $45 million in a Series A funding round.
Another company, Causality Link, provides an AI platform that takes in real-time news and market research to provide a complete view of the events and trends impacting the market. Pierre Haren, co-founder and CEO of Causality Link, says the ability to backtrack and look at what has happened in the past is important for causality.
“If you ask, ‘What was the causal model of the world just before Covid-19?’ our system can do it,” he says. “This is important for the rapid evolution of causal models because if you are trying to do back testing or explaining why a mistake was made a few years ago, you cannot use the current knowledge; you have to go back to the knowledge you had at that time.”
Haren is doubtful that the technology that underpins ChatGPT can do this. In tests, he says the chatbot can recall the net worth of Bill Gates from a specific year in time but that it struggles with backtracking on patterns. “It becomes very complex to associate a date with a pattern that has come from a statistical multiplicity of inputs,” Haren says.
The right use cases
The use cases for this technology are taking shape, but CausaLens director of applied data science Samir Sheldenkar, has seen scenarios around inflation and interest rates. Financial instrument models and market-making have also popped up.
And Sheldenkar isn’t counting out that causal AI and generative AI can work in tandem. “Causal AI can be quite complimentary to those types of technologies,” he says. “Causal AI helps the modeling process in interpreting the true relationships from the data rather than learning from the historical patterns.”
He says the strongest client interest has come from those who have tried to use AI in the past but haven’t achieved the outcomes they needed. “I think that’s the problem we’ve seen from correlational-based AI: It’s difficult to get into production because it’s harder for non-technical users to understand, interpret and trust,” Sheldenkar says. “Whereas causal AI brings a lot of trust and interpretability.”
Garcia sees a shift: “Causal AI allows you to move from this descriptive and predicative world into a more prescriptive world where you’re saying, ‘What should I do?’ or ‘What would happen if I did this?’”
Just a few years ago, advancements in transformer-based neural networks led to breakthroughs in generative AI. In October 2022, it was hardly a topic on many finance CTOs’ lips. Just a few months later, the likes of Bloomberg, S&P Global, HSBC, Deutsche Börse, Intercontinental Exchange, and many others were talking about genAI and LLMs.
Causal AI is not the talk of Wall Street right now. But generative AI should’ve taught everyone that the pace of artificial intelligence evolution is rapid—and just because causal isn’t being brought up in boardrooms today, doesn’t mean it won’t be a year from now.
Only users who have a paid subscription or are part of a corporate subscription are able to print or copy content.
To access these options, along with all other subscription benefits, please contact info@waterstechnology.com or view our subscription options here: http://subscriptions.waterstechnology.com/subscribe
You are currently unable to print this content. Please contact info@waterstechnology.com to find out more.
You are currently unable to copy this content. Please contact info@waterstechnology.com to find out more.
Copyright Infopro Digital Limited. All rights reserved.
As outlined in our terms and conditions, https://www.infopro-digital.com/terms-and-conditions/subscriptions/ (point 2.4), printing is limited to a single copy.
If you would like to purchase additional rights please email info@waterstechnology.com
Copyright Infopro Digital Limited. All rights reserved.
You may share this content using our article tools. As outlined in our terms and conditions, https://www.infopro-digital.com/terms-and-conditions/subscriptions/ (clause 2.4), an Authorised User may only make one copy of the materials for their own personal use. You must also comply with the restrictions in clause 2.5.
If you would like to purchase additional rights please email info@waterstechnology.com
More on Emerging Technologies
This Week: Startup Skyfire launches payment network for AI agents; State Street; SteelEye and more
A summary of the latest financial technology news.
Waters Wavelength Podcast: Standard Chartered’s Brian O’Neill
Brian O’Neill from Standard Chartered joins the podcast to discuss cloud strategy, costs, and resiliency.
SS&C builds data mesh to unite acquired platforms
The vendor is using GenAI and APIs as part of the ongoing project.
Chevron’s absence leaves questions for elusive AI regulation in US
The US Supreme Court’s decision to overturn the Chevron deference presents unique considerations for potential AI rules.
Reading the bones: Citi, BNY, Morgan Stanley invest in AI, alt data, & private markets
Investment arms at large US banks are taken with emerging technologies such as generative AI, alternative and unstructured data, and private markets as they look to partner with, acquire, and invest in leading startups.
Startup helps buy-side firms retain ‘control’ over analytics
ExeQution Analytics provides a structured and flexible analytics framework based on the q programming language that can be integrated with kdb+ platforms.
The IMD Wrap: With Bloomberg’s headset app, you’ll never look at data the same way again
Max recently wrote about new developments being added to Bloomberg Pro for Vision. Today he gives a more personal perspective on the new technology.
LSEG unveils Workspace Teams, other products of Microsoft deal
The exchange revealed new developments in the ongoing Workspace/Teams collaboration as it works with Big Tech to improve trader workflows.