The coming AI revolution in QIS
The first machine learning-based equity indexes launched in 2019. They are finally gaining traction with investors.
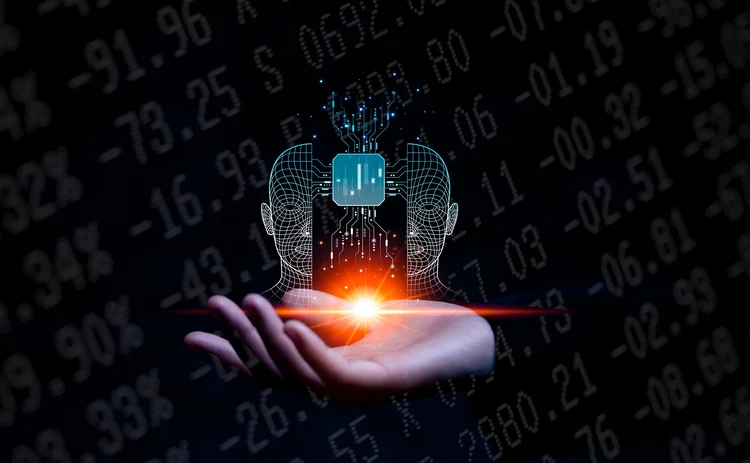
Need to know
- Investors are starting to embrace machine learning-based quantitative investment strategies after all but ignoring them for the past five years.
- ML-powered asset allocation products are becoming a fixture of fixed indexed annuities, while pension funds are showing interest in single-stock QIS.
- “We’re getting to a time when investors are ready to put money in ML-based models,” says Societe Generale’s Sandrine Ungari.
- Index provider MerQube recently launched a series of ML-powered equity factor indexes aimed at retail investors and is working closely with bank QIS desks on several other projects.
- ML-based QIS products still have short track records, while not all of them meet institutional transparency and documentation standards.
If you ask ChatGPT, quantitative investment strategies (QIS) are “exceptionally well-suited” to the application of artificial intelligence and machine learning techniques—and at least some dealers think investors are coming round to the same conclusion.
“As more and more clients are cognizant of ChatGPT, we also see a renewal of interest in how to use this technique within the context of systematic trading,” says Sandrine Ungari, head of cross-asset quant research at Societe Generale.
The bank is finally starting to see some real interest in its SG Machine Learning Global Equity Index, which has quietly built an impressive five-year track record since going live in 2019. “We’re getting to a time when investors are ready to put money in ML-based models,” says Ungari.
Insurers have been among the earliest adopters of ML-powered indexes. Strategies developed by banks including Citi, HSBC and Societe Generale have found their way into popular retirement products known as fixed indexed annuities sold by US carriers such as Athene and Securian Financial.
More recently, the SG Machine Learning Global Equity Index has drawn interest from pension funds, while indexing firm MerQube is targeting a broader audience for a set of AI-powered equity factor indexes it launched in October.
But not everyone is seeing the same trend. “I can’t say we’re inundated with requests about machine learning strategies,” says Spyros Mesomeris, global head of structuring at UBS.
The Swiss bank is using machine learning in a limited capacity—for instance, to collate data for economic forecasting—while sticking to well-established, explainable techniques such as regressions and reinforcement learning.
“Regressions are the first part of machine learning and regression-based tools are used for the construction of strategies,” says Mesomeris. “We’ve used these reinforcement tools for portfolio construction for a while, but we don’t see much selling of machine learning strategies across the Street.”
JP Morgan, which has led the way in adopting AI in other business lines, is also treading cautiously when it comes to QIS. The bank uses ML in a limited number of existing strategies, primarily for data extraction and price forecasting, but has no immediate plans to launch a fully AI-powered QIS index.
Even some of the most ardent proponents of ML initiatives in QIS warn against getting carried away at this early stage. Vinit Srivastava, chief executive and co-founder of MerQube, says that while ML works well for asset allocation and stock picking, applying it to more complex strategies may prove to be a more difficult problem.
“The amount of research that needs to happen and the innovation you need for that space is not trivial,” says Srivastava. “If you’re trying to allocate along the commodity curve or pick a strip of options based on machine learning and you’re facing sophisticated institutional clients, you need to explain why it’s better.”
Even so, he believes the technology will have a lasting impact on the QIS business. “People have promised a lot and delivered very little with new technologies in the past, but I do see, given where technology is today, that the time to create new ideas and actually get to market will be massively shortened if innovation continues at this pace.”
Performance anxiety
Launched in 2019, the SG Machine Learning Global Equity Index was among the first QIS products of its kind to hit the market.
The model uses Microsoft’s Light GBM—an open-source gradient-boosting framework based on decision tree algorithms—to scour over 80 common equity factors, such as price trends, earnings momentum and risk indicators, as well as fundamental metrics including profitability, leverage and earnings. The resulting index has long and short exposure to 330 names across Europe, Japan and the US.
Andrew Lapthorne, head of the quantitative index research group at Societe Generale, sees the index as a good litmus test for the use of ML in QIS strategies. “We wanted to see whether the technology would work in a live context, fully costed, long/short, because no one’s going to believe the technology unless you test it and demonstrate that we’re convinced by this application,” he says.
The ML system did not discover anything groundbreaking but has found novel ways to make use of existing factors and research. For instance, the model identified short-term reversals—the rate at which stocks move from over to undervalued—as one of the most significant factors in forecasting short-term price movements and correctly incorporated this into its trading strategy.
We’re getting to a time when investors are ready to put money in ML-based models
Sandrine Ungari, Societe Generale
“It hasn’t come up with a factor we weren’t aware of and it’s not saying 30 or 40 years of factor research is rubbish,” says Lapthorne. “It’s just doing it in a far more effective way and allows us to test new data sources quite well.”
Performance is also comparable to other factor strategies. “You’re not shooting the lights out. Our Sharpe is about one. It’s not making you 10% per annum, it’s making you 5% and for a lot of work. It’s not a miracle recipe, but if you think about the history of factor investing, it ends up with excess returns of around 4–5%, which is about where we’re at,” says Lapthorne.
Most importantly, out-of-sample performance over the past five years has closely mirrored backtest results—the ultimate proof of a well-designed quant strategy.
“I’ve never seen a backtest that aligned with out-of-sample performance. It’s not a perfect line up, there’s volatility in it around the global financial crisis, a bit around the Covid crisis, and we’re including not inconsequential trading costs given the high turnover in it, but it works. It’s made money over the last five-year period,” says Lapthorne.
That has been enough to convince some clients to commit money to the strategy. While SG does not disclose assets under management in the product, Lapthorne says some pension funds have begun implementing the model in their portfolios, while others are adapting it for specific use cases, such as small-cap equities or strong balance sheet names to hedge credit risk.
Societe Generale is not the only bank using ML in stock-picking strategies. HSBC also launched its AiPEX index—developed in conjunction with AI investment specialist EquBot—in 2019. The model uses machine learning tools provided by IBM Watson to analyze millions of data sources such as financial statements, economic releases and news articles to select from a universe of 1,000 US stocks. AiPEX is currently used in fixed indexed annuity products sold by Athene.
MerQube is targeting a more traditional investor base with its QueensField US AI Index, which went live last October. The model uses deep learning and natural language processing tools to build a portfolio of large-cap stocks and aims to outperform the S&P 500 on an annual basis.
Srivastava says the backtest results were “spectacular” with annualized returns over five years of 17.12% compared to 13.2% for the S&P 500. Live performance through June 11 has been a different story, with the index delivering 7.4% compared to 12.97% for the S&P 500.
Srivastava isn’t worried though. “A six-month period doesn’t really show anything as we haven’t had any different regimes since we went live,” he says. “It’s been a global bull market since last year. These will need two or three years of history before we can say anything on live performance.”
He adds the firm is working with QueensField AI to address the discrepancy, but notes the strategy attempted to avoid forward bias in historical backtests, for example by using point-in-time training data and both in- and out-of-sample testing.
While ML-powered stock-picking strategies are gaining credibility, questions remain about scalability and long-term performance. Two-way turnover in the SG Machine Learning Equity Index is extremely high, at almost 100% for each monthly rebalancing, which can be a drag on returns.
Dealers have already shifted their attention to other strategies. Some of the earliest FIA-focused AI indexes used natural language processing to mine news articles for sentiment signals—for example, Credit Suisse’s RavenPack AI index which launched in 2017. More recently, deep learning models have been used for asset allocation. Launched in 2021, HSBC’s AiMAX uses the technology behind AiPEX to forecast future returns of 15 investible exchange-traded funds and optimize allocation between them.
That same year, Citi launched its Grandmaster Index in conjunction with former chess world champion Garry Kasparov. Citi’s strategy uses machine learning techniques to forecast more than 1,000 future market return scenarios and identify sequences of asset allocation likely to deliver the strongest risk-adjusted returns over time.
ML-based asset allocation strategies have proven particularly popular with insurers. The Grandmaster Index is sold exclusively by Athene, while HSBC’s AiMAX has also found its way into fixed indexed annuity products.
Societe Generale also muscled into this part of the market in 2022 with its multi-asset Global Alpha Index. Developed in partnership with Paris-based AI for Alpha, which specializes in so-called explainable AI, the Global Alpha Index uses a deep learning model to determine optimal portfolio allocation from vast amounts of market information and financial metrics.
MerQube is also working on asset allocation strategies that are informed by macro factor data—some in partnership with dealers. “We are running other strategies that are not live yet and working with clients to get them live,” Srivastava tells Risk.net, WatersTechnology’s sibling publication. “We are working with banks and QIS desks to get these out into the market.”
Under the hood
Transparency and explainability remain the biggest barriers to further development of ML-based QIS products—especially more complex strategies. Banks are required to document the formulas within their investible index products and explain unexpected outcomes—particularly when a strategy underperforms.
“From a QIS strategy and business perspective, it has to be transparent for the end-client,” says UBS’s Mesomeris. “In theory, the client should be able to replicate your backtest if they have access to the same data.”
Documenting these models can be an uphill struggle. “Some are self-learning, so you’re not going to get the same results,” says Mesomeris. “It makes it more difficult for QIS teams to distribute machine learning models based on their own intellectual property, so they would rely on external partnerships typically to do this.”
The time to create new ideas and actually get to market will be massively shortened if innovation continues at this pace
Vinit Srivastava, MerQube
A structurer at one dealer says some techniques are already well standardized and can be fully disclosed in documentation, but cautions that the explainability of predictions is “a more nuanced problem deserving attention, with some models being considerably more intuitive than others”.
Tristan Fletcher, chief executive and co-founder of ChAI, a provider of AI-based tools for commodity markets, says internal compliance teams typically act as a major blocker of AI implementation for both buy- and sell-side firms, particularly as machine learning models become more complex.
“In this new world of large language models, it’s harder to know what’s under the hood,” says Fletcher. “You can retroactively try to understand by testing one thing at a time, but that’s not the same as opening it up and seeing if it intrinsically has a relationship between two datasets. Some of the older ML techniques allowed you to do that.”
While there’s no shortage of ML-based QIS products hitting the market, not all meet the transparency and robustness standards of institutional investors. Tom Haines, chief investment officer at Annexus, an investment product development company working with banks and insurers, says he has been shown “numerous” new ML- and AI-powered strategies in the past year.
“We have only moved forward with a few of those strategies that transparently shared their models with us during our standard diligence process and ran the backtest out-of-sample for a sufficient period of time,” says Haines.
He also calls for such indexes to be independently calculated. Solactiv acts as the calculation agent on AiPEX, while S&P provides the role for Societe Generale’s Global Alpha. Citi acts as calculation agent on its Grandmaster Index.
Proponents of ML-based QIS believe these issues can largely be overcome. Srivastava says the technology could revolutionize the market for systematic indexes, not least by dramatically cutting time to market.
“In the last few years, we’ve taken steps to more convincingly do this faster, but this adds another dimension,” he says. “We’re not there yet, but it’s where the disruption comes. First you have to be able to build the model, but even when you build that model, it doesn’t mean things will be automatically created—you have to query it and test it—but the speed at which you can create new things will be massively shortened.”
He sees the future of indexing akin to an app store, where banks and other users can access data to build strategies that make sense to their own clients.
“The proliferation of data providers is huge and the number of investment strategies you can show to clients is limited. People have limited shelf space so there are a lot of considerations that go into deciding what makes it to an end-client.”
For Annexus’s Haines, the coming months and years will be critical for the future of machine learning in investible index products.
“Machine learning will ultimately continue to evolve. Assuming it’s developed with a strong academic foundation, I think it will prosper and be very relevant in financial strategies. If in these early years there are mishaps, you may not see these strategies continue at all,” he says.
“It’s a critical time where it’s incumbent on those pioneers to take the best moral efforts and best educational and implementation processes to set the foundation so it prospers.”
Editing by Kris Devasabai
Further reading
Only users who have a paid subscription or are part of a corporate subscription are able to print or copy content.
To access these options, along with all other subscription benefits, please contact info@waterstechnology.com or view our subscription options here: http://subscriptions.waterstechnology.com/subscribe
You are currently unable to print this content. Please contact info@waterstechnology.com to find out more.
You are currently unable to copy this content. Please contact info@waterstechnology.com to find out more.
Copyright Infopro Digital Limited. All rights reserved.
As outlined in our terms and conditions, https://www.infopro-digital.com/terms-and-conditions/subscriptions/ (point 2.4), printing is limited to a single copy.
If you would like to purchase additional rights please email info@waterstechnology.com
Copyright Infopro Digital Limited. All rights reserved.
You may share this content using our article tools. As outlined in our terms and conditions, https://www.infopro-digital.com/terms-and-conditions/subscriptions/ (clause 2.4), an Authorised User may only make one copy of the materials for their own personal use. You must also comply with the restrictions in clause 2.5.
If you would like to purchase additional rights please email info@waterstechnology.com
More on Emerging Technologies
This Week: Startup Skyfire launches payment network for AI agents; State Street; SteelEye and more
A summary of the latest financial technology news.
Waters Wavelength Podcast: Standard Chartered’s Brian O’Neill
Brian O’Neill from Standard Chartered joins the podcast to discuss cloud strategy, costs, and resiliency.
SS&C builds data mesh to unite acquired platforms
The vendor is using GenAI and APIs as part of the ongoing project.
Chevron’s absence leaves questions for elusive AI regulation in US
The US Supreme Court’s decision to overturn the Chevron deference presents unique considerations for potential AI rules.
Reading the bones: Citi, BNY, Morgan Stanley invest in AI, alt data, & private markets
Investment arms at large US banks are taken with emerging technologies such as generative AI, alternative and unstructured data, and private markets as they look to partner with, acquire, and invest in leading startups.
Startup helps buy-side firms retain ‘control’ over analytics
ExeQution Analytics provides a structured and flexible analytics framework based on the q programming language that can be integrated with kdb+ platforms.
The IMD Wrap: With Bloomberg’s headset app, you’ll never look at data the same way again
Max recently wrote about new developments being added to Bloomberg Pro for Vision. Today he gives a more personal perspective on the new technology.
LSEG unveils Workspace Teams, other products of Microsoft deal
The exchange revealed new developments in the ongoing Workspace/Teams collaboration as it works with Big Tech to improve trader workflows.