The IMD Wrap: AI efforts will force renewed focus on data in 2024
Machine learning and generative AI offer a tremendous opportunity to help users obtain more insights from raw data, but these tools first need perfect datasets on which to base their decisions.
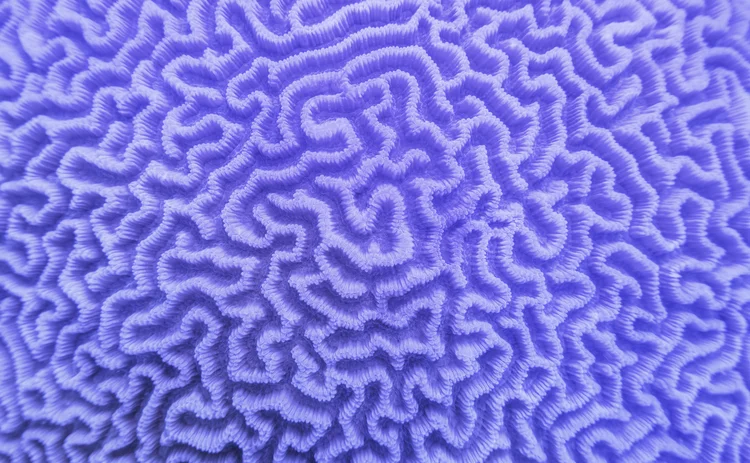
Ever been at a New Year’s Eve party and asked so-and-so’s new spouse what they think about the weather and suddenly find yourself hearing about their crypto investing strategy? Well, this year, the chatter has all been about artificial intelligence, and specifically generative AI, which endows AI programs with the ability to create new works based on what they learn.
And so, I wasn’t surprised when, after asking a question about how market data budgets might change in 2024, the conversation veered onto AI.
But first, budgets. Despite cost management efforts underway at end-user firms, continued consolidation among data sources and providers, and innovations such as data catalogs and pay-as-you-go data offerings that can help firms be more critical about their expenditure on market data, prices and costs continue to rise, with some services rising by outlandish amounts.
Here’s where AI comes in, and where things get interesting. One market data professional at a large buy-side firm says he expects to see tight controls continue around spend, but that consumption of data—and therefore budgets—will increase as the industry begins to utilize AI more widely, especially for analytics.
What I’m hearing is spend going up, but focused on fewer providers as core providers’ costs go up as they implement AI layers over their solutions
Simon Burton, CB Resourcing
“On both vendor and client sides, I think that AI can enhance existing analytics by providing more depth to the analysis, using historical data to find trends, and improve decision-making,” the data professional says.
Simon Burton, director at CB Resourcing, a specialist recruitment firm, also notes how AI is driving data spend and demand for skills.
“What I’m hearing is spend going up, but focused on fewer providers as core providers’ costs go up as they implement AI layers over their solutions,” Burton says.
But as AI becomes better understood and more widely adopted, he says it could become a driver of savings, rather than increased spend.
“I also think there will be great opportunities for new players to come in and use much more tech to do the aggregation of external data into products that historically have needed more humans—and so had a higher cost to entry,” he says. “I’m hearing efficiencies of 50% already for this type of data input/aggregation work.”
These are both interesting perspectives because they speak to potential uses of AI beyond many of the chatbot-style use cases thus far, focused on making information more easily discoverable and accessible, such as Bloomberg’s BloombergGPT and S&P’s ChatIQ, both of which aim to solve the challenge faced by clients of these data giants—how to make the most of the wealth of data they have, and how to find out what data exists and how it’s connected to other datasets.
Indeed, AI was the hottest topic of 2023, and the best is yet to come in terms of what else it can deliver.
But it’s not all plain sailing: there are issues around resourcing (by which I mean people with expertise in developing AI tools and in the subject matter areas being addressed) as well as around validating the AI models themselves—all of which will be overcome but may prove short-term barriers.
“It will take time for the industry to fully utilize AI as the pool of related resources is still rather shallow,” the data professional warns.
Personally, I’d call that a conservative view. I suspect that although most companies are still dipping their toes into the AI waters, there are plenty who are way ahead of where they profess to be, and many of those saw the writing on the wall years ago and have been laying the groundwork for when technology would catch up. Because, as we all (should) know, you can’t hope to get AI right unless you first get your underlying data in order, and the experts who know how to do this may be as valuable an asset as the data itself.
One person who saw this coming is Barbara Matthews, founder and CEO of BCMstrategy, a company turning public policy information into data and analysis of the impact of those policy decisions on financial markets. She says it was clear to her a decade ago that computers would be reading and writing data and making decisions, but that suitable datasets to train AI and ML models simply didn’t exist—especially in her specialist field of public policy research. So, after founding BCMstrategy in 2017, she went about building those datasets herself.
This is not Matthews’ first rodeo. She has held numerous senior legal and advisory roles in the world of government and public policy over her career, including senior council to the Financial Services Committee in the US House of Representatives, a senior advisor to the US Department of the Treasury, and financial attaché to the European Union at the US Department of State. She has lived and breathed financial regulatory policy for 30 years, and now she’s using that experience—along with data and new technologies—to predict the impact of policy decisions and regulation.
But, she says, although there may be a large universe of consumers for this data, it’s not necessarily something for the general public, but rather for a group of focused subject matter experts. And it’s with that niche focus in mind that she has built the datasets and is training her AI models to interrogate the data.
Her AI is trained on three areas: monetary policy (such as inflation, GDP growth, consumption, wages, and unemployment levels); central bank digital asset policies (cryptocurrencies, stablecoins, and decentralized and tokenized finance); and energy and climate policies (disclosures, greenwashing, renewables, and carbon-based emissions).
“For genAI users, this helps them connect more data and accelerates their ability to anticipate likely policy and market outcomes,” Matthews says.
That first batch of users will get access to BCMstrategy’s AI tool in January and will comprise three categories of subject matter experts: policy experts who engage with policy decisions on a daily basis; portfolio managers looking for immediate insight into how policies drive prices; and research analysts looking to validate investment theses over different time horizons.
That first batch of users will be deliberately limited. “We’re not making our public policy generative AI—which is in beta now—available to everyone on the internet because we want to be responsible about the training process. Generative AI learns from the questions it receives. It is crucial to choose carefully who the first batch of users are, because those users are both providing training and benefiting from it,” Matthews says. “If you’re serious about integrity and efficiency in the training data and process, then you need a subject matter expert doing this like me who knows off the top of their head if the AI is giving a good answer or not, and who can quickly identify the next step in the training process to address shortcomings.”
Herein lies part of the people problem: you need AI experts to build a model that works properly, but you also need subject matter experts on staff or invested users willing to spend time contributing to its success. And the more specialized a dataset is, the greater potential there is for AI to help deliver valuable insights. But also, the harder it is to find that core of right people.
This has not gone unnoticed by recruiters, such as Burton. “There is definitely a talent gap on verifying vendor solutions and the safety and reliability of their language models. People are less worried about the very large/core vendors, but the long tail of specialists and startups will require a lot of due diligence,” he says.
Stepping up to provide that due diligence are companies like CitrusX, an Israeli startup that recently raised $4.5 million in seed funding from investors led by Toronto-based venture capital firm AWZ. The vendor’s hypothesis is that the stumbling block to AI is the machine learning models on which it is based. So, fix the models, and the AI will work correctly. Don’t just assume that AI will spot and fix errors in the underlying data—it won’t.
When you take the Big Tech ‘kitchen sink’ approach to language extraction, you need machines to sift through all that content
Barbara Matthews, BCMstrategy
While working in her previous role as VP of product at Kymera Labs, which creates synthetic data for clients in the financial industry, CitrusX co-founder and CEO Noa Srebrnik noticed a bottleneck between the creation of data and a firm’s ability to put that data into production in a timely and useful manner because of all the manual risk and compliance checks it first needed to go through.
CitrusX has built its model validation and governance platform to address the broad spectrum of AI from logistic regression to neural networks and large language models, and to be dataset and industry agnostic. Initially, the company is focusing on the financial, insurance, and security sectors—in part because the vendor still only has a small team, but also because it sees these as the most critical industries to address, and which are also likely to see stringent regulation about their use of AI.
“From when a company builds its first model, we’re there to validate and test it,” Srebrnik says. “We want to identify any weak spots so we can target you at the relevant places where you have problems … and where a model may not be acting as it should.”
Good governance of the data on which AI is trained—just like any other dataset—will deliver more accurate results, which clients recognize delivers greater value through, among other things, better customer service, she says.
Matthews agrees that validating one’s models—and what data is included in a model—is as critical as the training process itself, and that the current fad of “a lot of people jumping in and thinking that they’ll figure it out as they go,” can prove unproductive and a waste of resources.
“When you take the Big Tech ‘kitchen sink’ approach to language extraction, you need machines to sift through all that content,” Matthews says. “But because the machines don’t know what the right content is, you need specialized learning models with a foundation in a core of specialized training data. Without precise language inputs, of course LLMs will hallucinate. It’s garbage in, garbage out.”
Also, she warns, don’t try to boil the ocean just to make a cup of tea. Smaller language models offer not only more focused and specialized data for training AI models, but also come with a smaller maintenance and governance burden.
“The giants have multi-billion parameter models because they’re taking in all of the internet in order to be able to answer any question,” Matthews says. “But for most use cases, especially tightly focused use cases with highly trained experts as users, a smaller language model (like the Databricks Dolly model or Meta’s Llama model) will be more operationally—and computationally—efficient when processing precise language training datasets because the models don’t have to sift through a bunch of junk to deliver a solid answer. Do most firms really need something that large, or a platform where data leakage could be a problem?”
And that, I think, is something that is inevitable in 2024 as the rise of AI continues. Even with the best intentions, we’re bound to see instances emerge of data leakage or AIs gone haywire. A shortcut here, an erroneous data point there, an over-reliance on under-tested tools that were rushed into production to meet sudden demand, are all surefire ways to singlehandedly implode your company. But, as the first rule of carpentry dictates, “Measure twice, cut once”—or, put another way, plan, check and check again before executing. Get the data right, and you and your AI will be fine.
Or maybe I’m just an AI bot lulling you into a false sense of security before I take over the world. Feel free to write me and tell us how you feel about AI and its potential impact on market data and trading tech in 2024 at max.bowie@infopro-digital.com
Here’s wishing our readers and all the other bots out there a joyful, healthy, prosperous, and peaceful 2024.
Only users who have a paid subscription or are part of a corporate subscription are able to print or copy content.
To access these options, along with all other subscription benefits, please contact info@waterstechnology.com or view our subscription options here: http://subscriptions.waterstechnology.com/subscribe
You are currently unable to print this content. Please contact info@waterstechnology.com to find out more.
You are currently unable to copy this content. Please contact info@waterstechnology.com to find out more.
Copyright Infopro Digital Limited. All rights reserved.
As outlined in our terms and conditions, https://www.infopro-digital.com/terms-and-conditions/subscriptions/ (point 2.4), printing is limited to a single copy.
If you would like to purchase additional rights please email info@waterstechnology.com
Copyright Infopro Digital Limited. All rights reserved.
You may share this content using our article tools. As outlined in our terms and conditions, https://www.infopro-digital.com/terms-and-conditions/subscriptions/ (clause 2.4), an Authorised User may only make one copy of the materials for their own personal use. You must also comply with the restrictions in clause 2.5.
If you would like to purchase additional rights please email info@waterstechnology.com
More on Data Management
New working group to create open framework for managing rising market data costs
Substantive Research is putting together a working group of market data-consuming firms with the aim of crafting quantitative metrics for market data cost avoidance.
Off-channel messaging (and regulators) still a massive headache for banks
Waters Wrap: Anthony wonders why US regulators are waging a war using fines, while European regulators have chosen a less draconian path.
Back to basics: Data management woes continue for the buy side
Data management platform Fencore helps investment managers resolve symptoms of not having a central data layer.
‘Feature, not a bug’: Bloomberg makes the case for Figi
Bloomberg created the Figi identifier, but ceded all its rights to the Object Management Group 10 years ago. Here, Bloomberg’s Richard Robinson and Steve Meizanis write to dispel what they believe to be misconceptions about Figi and the FDTA.
SS&C builds data mesh to unite acquired platforms
The vendor is using GenAI and APIs as part of the ongoing project.
Aussie asset managers struggle to meet ‘bank-like’ collateral, margin obligations
New margin and collateral requirements imposed by UMR and its regulator, Apra, are forcing buy-side firms to find tools to help.
Where have all the exchange platform providers gone?
The IMD Wrap: Running an exchange is a profitable business. The margins on market data sales alone can be staggering. And since every exchange needs a reliable and efficient exchange technology stack, Max asks why more vendors aren’t diving into this space.
Reading the bones: Citi, BNY, Morgan Stanley invest in AI, alt data, & private markets
Investment arms at large US banks are taken with emerging technologies such as generative AI, alternative and unstructured data, and private markets as they look to partner with, acquire, and invest in leading startups.