Domain-specific AI: the hot topic of 2024?
Generative AI is increasingly being applied to specific domains within finance. But experts are divided on whether targeted models will take over from their general-purpose cousins.
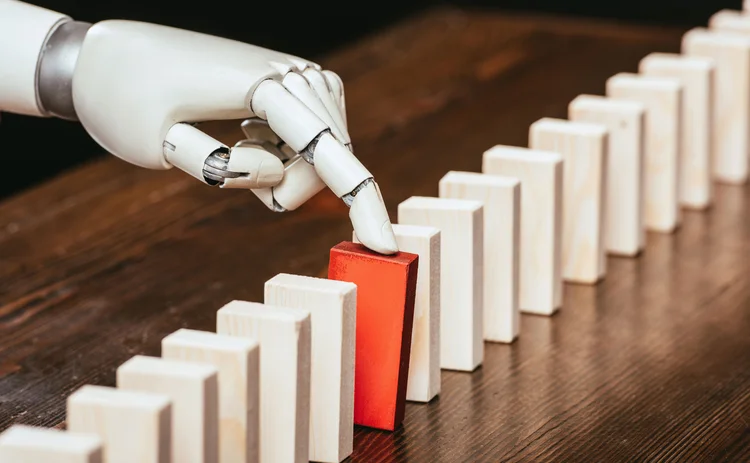
It will come as no surprise to fintech professionals that Collins Dictionary’s word of 2023 was ‘AI’. The technology hogged the headlines all year—from the release of GPT-4 to the engrossing standoff between OpenAI CEO Sam Altman and his board.
But while much of the news was driven by advances in AI’s ability to perform multiple tasks at a high level—the launch of Google’s latest multimodal model Gemini, for example—more targeted applications of AI were often overlooked. Domain-specific uses of generative AI have proliferated in the last year, and are driving significant efficiencies, particularly in financial markets. These can come in the form of purpose-built verticalized models or existing foundation models overlaid with industry-specific information.
Anju Kambadur, Bloomberg’s head of AI engineering, manages a team of almost 300 software engineers and researchers who build AI-enabled products and infrastructure. He explains that what we now call AI—a combination of machine learning, search, and natural language processing—is uniquely well suited to finance.
“The amount of unstructured information that contains key decision variables is immense in finance. Even if you’re just talking about the US and European markets, where transparency is amazing—you get filings that come out on time, regulations are strict, and you know exactly how a number is computed, because of generally accepted accounting principles—all of that is still textually represented. Just providing liquidity to unstructured data in finance is one of the most challenging and most valuable problems to address,” Kambadur says.
Decision-making in finance often means accounting for news stories, filings, prospectuses, analyst reports, market data, and filings. Generative AI helps professionals to synthesize that mass of structured and unstructured data and quickly find the most significant takeaways.
We believe large enterprises will require a multi-pronged strategy, using a combination of commercial, open-source, and home-grown, domain-specific models—which will all operate in harmony
Anju Kambadur, Bloomberg
Models allowing professionals in given roles to access more data tailored to their business needs and packaged to suit their consumption preferences could save firms incalculable sums when time, human resources, and cognitive burden are factored in. And this is exactly what tech companies and financial institutions are beginning to focus on.
Alongside the CTO’s machine-learning strategy team, Kambadur’s AI engineering group helped develop BloombergGPT, a large language model trained on a corpus of 700 billion tokens taken from public data sets as well as Bloomberg’s own archives. When research into BloombergGPT began in early 2022, Bloomberg decided that third-party models coupled with APIs were not up to the job, so it set to work designing its own LLM specifically for the financial domain from scratch. BloombergGPT is intended to enhance the Bloomberg Terminal, enriching capabilities such as sentiment analysis, named-entity recognition, news classification, charting, and question answering.
And Bloomberg is not the only company seeking to channel the benefits of generative AI towards specific use cases in finance.
Investment firm AllianceBernstein has used generative AI to read documents like prospectuses and regulatory filings and to write commentaries and market outlooks for clients. Now, the firm claims to have boosted the accuracy of its models to 95%—significantly ahead of the 60–70% achievable by ChatGPT.
Meanwhile, asset management firm Bridgewater Associates and Anthropic AWS are building an investment analyst assistant, which will help junior members of the team by performing data tasks and reasoning like an investment analyst.
And experts from IBM and Cognizant say that generative AI may soon transform high-frequency trading by enabling big firms to make significant transactions fractions of a second after triggering events without jeopardizing market stability.
With more and more use cases emerging, OpenAI is also seeking to capitalize on the trend for verticalized applications of generative AI. It recently launched custom GPTs, “a new way for anyone to create a tailored version of ChatGPT to be more helpful in their daily life, at specific tasks, at work, or at home”. The idea is that verified builders can design best-of-breed agents for targeted tasks, and then share them on a ‘GPT Store’, where they can earn money if their inventions gain traction.
A senior manager at a large cloud provider echoed the idea that specialized models are coming into vogue at a recent industry conference. “When we start working with customers, they’re really out there to prove use cases. These could be small, generalized use cases... As this journey progresses, we are increasingly seeing disillusionment surrounding the application of these generic large language models. The original use case may work well, but then the follow-on tasks might not work as well, because the model doesn’t accommodate a lot of business context,” they said. “The answer is either fine tuning (creating your own smaller models, which are more cost-efficient in the long term), or finding ways to apply a lot more business context into the large models.”
Hone or hydrate?
For all the enthusiasm they have generated, commercially available large language models still have their shortcomings. For example, they do not have access to real-time data sources, so information is updated slowly—a crucial weakness in a world like finance, where markets can move fast.
To improve the efficiency of models and ensure they have access to current information, a new method has become popular in the last year: retrieval-augmented generation. This forces models to perform a task by referring only to relevant data from an external data store (which can be regularly updated), reducing the scope for error and the amount of compute required. This technique, coupled with a rich ecosystem of libraries, APIs, and databases can allow domain-specific models to perform tasks with up-to-date information at low latency.
One company that has recently launched a domain-specific model is Reorg, a provider of credit news and data for leveraged finance and restructuring professionals including hedge funds, investment banks, and law firms. Reorg has 180 staff—reporters, analysts, and lawyers—writing content every day on credit-related events such as distress, bankruptcy, and issuances. By combining all of its proprietary content in a vector database and adding data feeds for external content like SEC filings, transcripts, and CLO ownership data, it has created a system that allows users to communicate with a repository of credit-related information. CreditAI can summarize articles, write an investment thesis in bullet points, and even loosely predict the likelihood of a bankruptcy in the near term based on past disclosures like 10-K forms.
Kent Collier, CEO of Reorg, says that one of the principal challenges for investors when the price of a security changes dramatically is the need to get up to speed on relevant information before others. This is why real-time access to Reorg’s own news stories is a key function of CreditAI.
“For us, the thing that it’s going to hack is the time to get information. Because remember, it’s not the answer that matters—the answers are all commoditized. The questions are what makes this process so special,” Collier says.
In other words, specialized models like CreditAI can cater to the varied needs of different users by presenting the necessary information in a particular format, learning how a hedge fund manager likes to consume information as opposed to a law firm, for example. It is able to shape its answers for different users based on what sorts of questions they ask and how they phrase them to get the most useful answers, a process called prompt engineering.
This system interacts with all the questions, and if one firm has a different way to think about revenue growth versus Ebitda margin, and another one has a different way to think about leverage, you can ask all those questions of the system, and the answer is very much tailored to the question, which I think is really the special sauce in the investing advisory space,” Collier says.
Other advantages offered by CreditAI include a permissions-based architecture and the ability to cite source documents for the information it presents, making it auditable.
In the future, Reorg plans to start indexing more data including the private credit documents of leveraged loan issuers to increase CreditAI’s content base. But the first step will be to review and respond to feedback from the platform’s 30,000 users who have had access to the tool for two months.
“Not everything is going to be perfect. It will probably have some hallucinations. But that’s where we learn and get better,” Collier told WatersTechnology shortly before the launch.
Nonetheless, Collier is optimistic that domain-specific applications of generative AI will increase in 2024 as the benefits become clearer. “People are going to continue to verticalize their GPT, because at the end of the day, that will provide better answers. That way you’re not trying to boil the ocean. I’m sure you’ll see GPTs for things like retail trends or shipbuilders, etc. If you can get a compact set of data, you can ask better questions of that data and probably get better answers.”
A third way
Although verticalization is getting more attention now, Bloomberg’s Kambadur explains that domain-specific AI is not a new concept. “Using domain-specific models are a common industry practice that pre-date LLMs, and I suspect will be needed even in this era of LLMs. While it’s true models like GPT-4 can perform well on private datasets for some tasks, what if you need over 99% accuracy on the answers in your use case, such as is required when extracting and publishing economic and company fundamentals that are found in public filings?” he says.
Kambadur adds that while a commercial foundational model fine-tuned on domain-specific data may do the job for some use cases, this is not necessarily the most effective approach if it has to be done on-prem or at cost to backfill large amounts of historical data. “We believe large enterprises will require a multi-pronged strategy, using a combination of commercial, open-source, and home-grown, domain-specific models—which will all operate in harmony.”
Training AI models can be very expensive, particularly given the need for high-quality graphics processing units (GPUs), which are needed for parallel processing. On top of this, building a domain-specific model also means employing subject-matter experts to generate and annotate data for the models to train on. This is a key step, as the mechanism used for selecting data and sorting it into discrete categories informs the model’s eventual understanding of the sector.
For this reason, Kambadur says, the most understated challenge in building domain-specific models for finance is finding the people and the data that capture the experiences you want users to have.
Bill Murphy, managing partner at consultancy Cresting Wave, agrees that a well-built model takes a good deal of general care from highly qualified specialists.
“I see a lot of people saying 'just hack something together'. And then it gets shown to the CEO and they ask a couple of questions and say 'oh, this is amazing'. But the right way is to build an ongoing platform that can scale. The right way is to make sure that all the data is dealt with appropriately. The truth is that there's probably some garbage in the way that you uploaded it, too, and that should be stripped out,” Murphy explains.
I’m a big believer in the broad models hydrated with specific information and layers of UI on top
Bill Murphy, Cresting Wave
Among the many companies promoting specialized applications of AI, most can be broken down into three types: those that interpret or format the queries; the providers of the LLMs themselves, which run the queries; and those that assemble and present the results. Most of the specialization is concentrated in the outer functions, with the process of running queries largely left to the big tech providers.
Cresting Wave’s Murphy does not believe many companies can afford to develop private offerings for all three of these functions. But he also warns against micro models developed to answer certain questions without reference to general learning.
“You’re going to wind up with these silos of well-trained models that understand how to talk to a specific role circa a certain data set or vocabulary. But maybe something massively changes in the world, and then you're constantly trying to keep up. So, I’m a big believer in the broad models hydrated with specific information and layers of UI on top,” Murphy says.
Murphy is also a member of the advisory board for BlueFlame AI, a company that helps alternative investment managers to take advantage of commercially available LLMs by hydrating the models with a corpus of knowledge and data from the company and industry to enable specialization.
Raj Bakhru, CEO and co-founder of BlueFlame, explains that the idea for the company was conceived in the period of enthusiasm that followed the release of ChatGPT.
“Prior to launching, we did close to 30 client interviews. We asked them 'you’ve played with ChatGPT, how would this apply to your business? How would you like to see this work? Where do you feel like there's opportunity? Where do you feel like you're doing really tedious manual work?’” Bakhru says.
BlueFlame now works on building specialized systems that understand industry context. In the case of private equity, for example, it can teach a model how to distinguish between closely related concepts such as a letter of intent, an indication of interest, and a bid letter.
While specialization is necessary to make an LLM useful when dealing with industry-specific concepts, Bakhru says, it is also crucial to train models with regulatory imperatives in mind.
“If you were to just go wild, build a system against whatever LLM is out there, just start connecting your data and your sources, you're going to end up afoul of regulatory expectations as well as your clients’ privacy and cyber expectations. You have all these kids coming out of college who think they can build a great system. But it's not financial services ready, it doesn't understand the semantics of the space, and it also just doesn't meet the baseline requirements of the space from a cyber, privacy, and compliance perspective,” Bakhru says.
But potential pitfalls notwithstanding, Bakhru and Murphy are convinced that specialized applications of existing LLMs—if carefully implemented—have the potential to transform finance by saving market players valuable time on lengthy information processing tasks.
Murphy compares the current attitude to AI with the widespread scepticism towards the potential for computers to beat chess grandmasters around the turn of the century. But even while many experts thought computers would never be a match for world chess champions, there was a period when a discipline called centaur chess—combining a well-trained chess player with a computer—could outsmart either a computer by itself or a grandmaster.
Much like centaur chess, Murphy believes commercially available LLMs provided with specialist data sets are the future. “If you call the general purpose the computer, and the grandmaster the industry professional, I think the specialized information plus the general model is eventually going to be the ideal combination,” he says.
Further reading
Only users who have a paid subscription or are part of a corporate subscription are able to print or copy content.
To access these options, along with all other subscription benefits, please contact info@waterstechnology.com or view our subscription options here: http://subscriptions.waterstechnology.com/subscribe
You are currently unable to print this content. Please contact info@waterstechnology.com to find out more.
You are currently unable to copy this content. Please contact info@waterstechnology.com to find out more.
Copyright Infopro Digital Limited. All rights reserved.
As outlined in our terms and conditions, https://www.infopro-digital.com/terms-and-conditions/subscriptions/ (point 2.4), printing is limited to a single copy.
If you would like to purchase additional rights please email info@waterstechnology.com
Copyright Infopro Digital Limited. All rights reserved.
You may share this content using our article tools. As outlined in our terms and conditions, https://www.infopro-digital.com/terms-and-conditions/subscriptions/ (clause 2.4), an Authorised User may only make one copy of the materials for their own personal use. You must also comply with the restrictions in clause 2.5.
If you would like to purchase additional rights please email info@waterstechnology.com
More on Data Management
New working group to create open framework for managing rising market data costs
Substantive Research is putting together a working group of market data-consuming firms with the aim of crafting quantitative metrics for market data cost avoidance.
Off-channel messaging (and regulators) still a massive headache for banks
Waters Wrap: Anthony wonders why US regulators are waging a war using fines, while European regulators have chosen a less draconian path.
Back to basics: Data management woes continue for the buy side
Data management platform Fencore helps investment managers resolve symptoms of not having a central data layer.
‘Feature, not a bug’: Bloomberg makes the case for Figi
Bloomberg created the Figi identifier, but ceded all its rights to the Object Management Group 10 years ago. Here, Bloomberg’s Richard Robinson and Steve Meizanis write to dispel what they believe to be misconceptions about Figi and the FDTA.
SS&C builds data mesh to unite acquired platforms
The vendor is using GenAI and APIs as part of the ongoing project.
Aussie asset managers struggle to meet ‘bank-like’ collateral, margin obligations
New margin and collateral requirements imposed by UMR and its regulator, Apra, are forcing buy-side firms to find tools to help.
Where have all the exchange platform providers gone?
The IMD Wrap: Running an exchange is a profitable business. The margins on market data sales alone can be staggering. And since every exchange needs a reliable and efficient exchange technology stack, Max asks why more vendors aren’t diving into this space.
Reading the bones: Citi, BNY, Morgan Stanley invest in AI, alt data, & private markets
Investment arms at large US banks are taken with emerging technologies such as generative AI, alternative and unstructured data, and private markets as they look to partner with, acquire, and invest in leading startups.