Quants look to language models to predict market impact
Oxford-Man Institute says LLM-type engine that ‘reads’ order-book messages could help improve execution
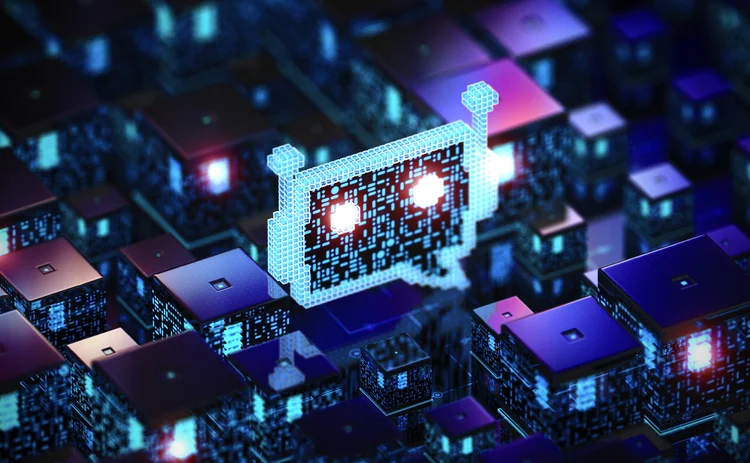
Quants using the technology behind tools such as ChatGPT have developed a model that can forecast how large trades might move asset prices.
A team at the Oxford-Man Institute—a research unit at the University of Oxford co-founded and funded by the Man Group hedge fund—has built a machine learning model that roots out patterns in the messages traders send to an exchange’s limit order book.
Stefan Zohren, a research fellow at the institute and a quant at Man Group overlooking futures execution research, says the model can “look inside” the order book and build a better picture of trading activity. He says traders and quants might use the model to plan out trading trajectories and improve execution algorithms.
Unlike other efforts to model limit order books, the Oxford-Man model reads individual order messages and guesses what the next orders in the sequence are most likely to be. It can pick up, for example, that a large sell order might induce cancellations on the bid side of the book that could lead to a price shift.
You might see at one level a whole bunch of smaller orders and at another level just one big order. That might imply a form of imbalance or price pressure, even though, in terms of total level size, there might be no imbalance
Stefan Zohren, Oxford-Man Institute
Its approach is similar to those of so-called large language models, such as ChatGPT. Data scientists train LLMs by making them attempt, often millions of times over, to replace missing words in unfinished sentences.
Like LLMs, the Oxford-Man engine converts messages into so-called tokens to reduce, normalise and simplify the data.
Better execution
The hidden costs of so-called market impact—how far a firm can move prices against itself with its own trades—eat into the profitability of investing strategies. Hedge funds, including Man Group, invest heavily in ways to execute trades so as to minimize such effects.
Zohren says the model can also generate richer synthetic data than other methods—data that could be used to train other machine learning models for tasks such as execution, or to make price predictions and identify investing opportunities. Quants sometimes create such data to help train machine learning models when real-world data is insufficient.
Past efforts to model the dynamics of limit order books have used data relating to levels of supply and demand at different price levels in the book. Zohren says the new model picks up additional information that would otherwise remain hidden.
“You might see at one level a whole bunch of smaller orders and at another level just one big order,” he says. “That might imply a form of imbalance or price pressure, even though, in terms of total level size, there might be no imbalance.”
Zohren says the language-model approach does a better job than the generative adversarial networks that quants have attempted to use for similar tasks. These networks have mostly been used to generate synthetic price data, but they can also generate order book data that looks realistic. However, they are blind to the interplay between market participants that determines an order book’s composition.
The researchers trained the new model using data for Alphabet and Intel stocks that traded on Nasdaq from July to December 2022. Starting with 500 historical messages and tasked with generating the next 100, the model produced data with significant correlations to real world mid prices for 80 to 100 messages into the future. For most stocks, that would mean about 15 to 60 seconds of trading time.
Other quants have welcomed the work as an important step in addressing a longstanding problem.
Roel Oomen, global head of fixed income and currencies quantitative trading at Deutsche Bank, says that even a generator that was too slow or impractical to be used in live trading could help to calibrate execution or market-making algorithms.
“Evaluating the performance of a hypothetical trading strategy against naively resampled historical data doesn’t capture the fact that the strategy would likely have altered the evolution of the market itself,” he says.
Oomen adds that a realistic market generator—one that accurately captures how the probability of a particular change in the order book is conditional on information such as order sizes, time of day and trade history—provides “a more coherent way of simulating a market”. Such a model, he says, “can incorporate your hypothetical strategy by recognizing, for instance, that a large order is going to impact future liquidity in the order book”.
Oomen is also working on ways to simulate limit order books, but is using different approaches to those of Zohren and his colleagues.
Jean-Philippe Bouchaud, chair and head of research at Capital Fund Management, sees modeling market impact as something of a “holy grail” in quant finance.
He says his firm conducted similar research to that of Oxford-Man but sought to model effects over longer timeframes, and that this ultimately proved unsuccessful.
Bouchaud points out that financial market phenomena occur over multiple timescales, and that modeling market impact over seconds is only one part of the “super-difficult problem” of modeling it over minutes, hours or even days.
The quants behind Oxford-Man’s research say their approach can be used more widely in finance. In a working paper, the researchers state that the “radical bottom-up approach” that has driven recent advances in LLMs “could similarly usher in the next generation of generative financial models”.
Zohren says the institute’s research has sometimes led to strategies that Man Group has itself put into practice. However, he adds that this specific limit order book research has yet to be applied in live trading.
Only users who have a paid subscription or are part of a corporate subscription are able to print or copy content.
To access these options, along with all other subscription benefits, please contact info@waterstechnology.com or view our subscription options here: http://subscriptions.waterstechnology.com/subscribe
You are currently unable to print this content. Please contact info@waterstechnology.com to find out more.
You are currently unable to copy this content. Please contact info@waterstechnology.com to find out more.
Copyright Infopro Digital Limited. All rights reserved.
As outlined in our terms and conditions, https://www.infopro-digital.com/terms-and-conditions/subscriptions/ (point 2.4), printing is limited to a single copy.
If you would like to purchase additional rights please email info@waterstechnology.com
Copyright Infopro Digital Limited. All rights reserved.
You may share this content using our article tools. As outlined in our terms and conditions, https://www.infopro-digital.com/terms-and-conditions/subscriptions/ (clause 2.4), an Authorised User may only make one copy of the materials for their own personal use. You must also comply with the restrictions in clause 2.5.
If you would like to purchase additional rights please email info@waterstechnology.com
More on Emerging Technologies
This Week: Startup Skyfire launches payment network for AI agents; State Street; SteelEye and more
A summary of the latest financial technology news.
Waters Wavelength Podcast: Standard Chartered’s Brian O’Neill
Brian O’Neill from Standard Chartered joins the podcast to discuss cloud strategy, costs, and resiliency.
SS&C builds data mesh to unite acquired platforms
The vendor is using GenAI and APIs as part of the ongoing project.
Chevron’s absence leaves questions for elusive AI regulation in US
The US Supreme Court’s decision to overturn the Chevron deference presents unique considerations for potential AI rules.
Reading the bones: Citi, BNY, Morgan Stanley invest in AI, alt data, & private markets
Investment arms at large US banks are taken with emerging technologies such as generative AI, alternative and unstructured data, and private markets as they look to partner with, acquire, and invest in leading startups.
Startup helps buy-side firms retain ‘control’ over analytics
ExeQution Analytics provides a structured and flexible analytics framework based on the q programming language that can be integrated with kdb+ platforms.
The IMD Wrap: With Bloomberg’s headset app, you’ll never look at data the same way again
Max recently wrote about new developments being added to Bloomberg Pro for Vision. Today he gives a more personal perspective on the new technology.
LSEG unveils Workspace Teams, other products of Microsoft deal
The exchange revealed new developments in the ongoing Workspace/Teams collaboration as it works with Big Tech to improve trader workflows.